Combining machine learning with Cox models to identify predictors for incident post-menopausal breast cancer in the UK Biobank
Scientific Reports(2023)
摘要
We aimed to identify potential novel predictors for breast cancer among post-menopausal women, with pre-specified interest in the role of polygenic risk scores (PRS) for risk prediction. We utilised an analysis pipeline where machine learning was used for feature selection, prior to risk prediction by classical statistical models. An “extreme gradient boosting” (XGBoost) machine with Shapley feature-importance measures were used for feature selection among ≈ 1.7 k features in 104,313 post-menopausal women from the UK Biobank. We constructed and compared the “augmented” Cox model (incorporating the two PRS, known and novel predictors) with a “baseline” Cox model (incorporating the two PRS and known predictors) for risk prediction. Both of the two PRS were significant in the augmented Cox model ( p<0.001 ). XGBoost identified 10 novel features, among which five showed significant associations with post-menopausal breast cancer: plasma urea (HR = 0.95, 95
更多查看译文
关键词
Biochemistry,Biomarkers,Cancer,Diseases,Genetics,Medical research,Science,Humanities and Social Sciences,multidisciplinary
AI 理解论文
溯源树
样例
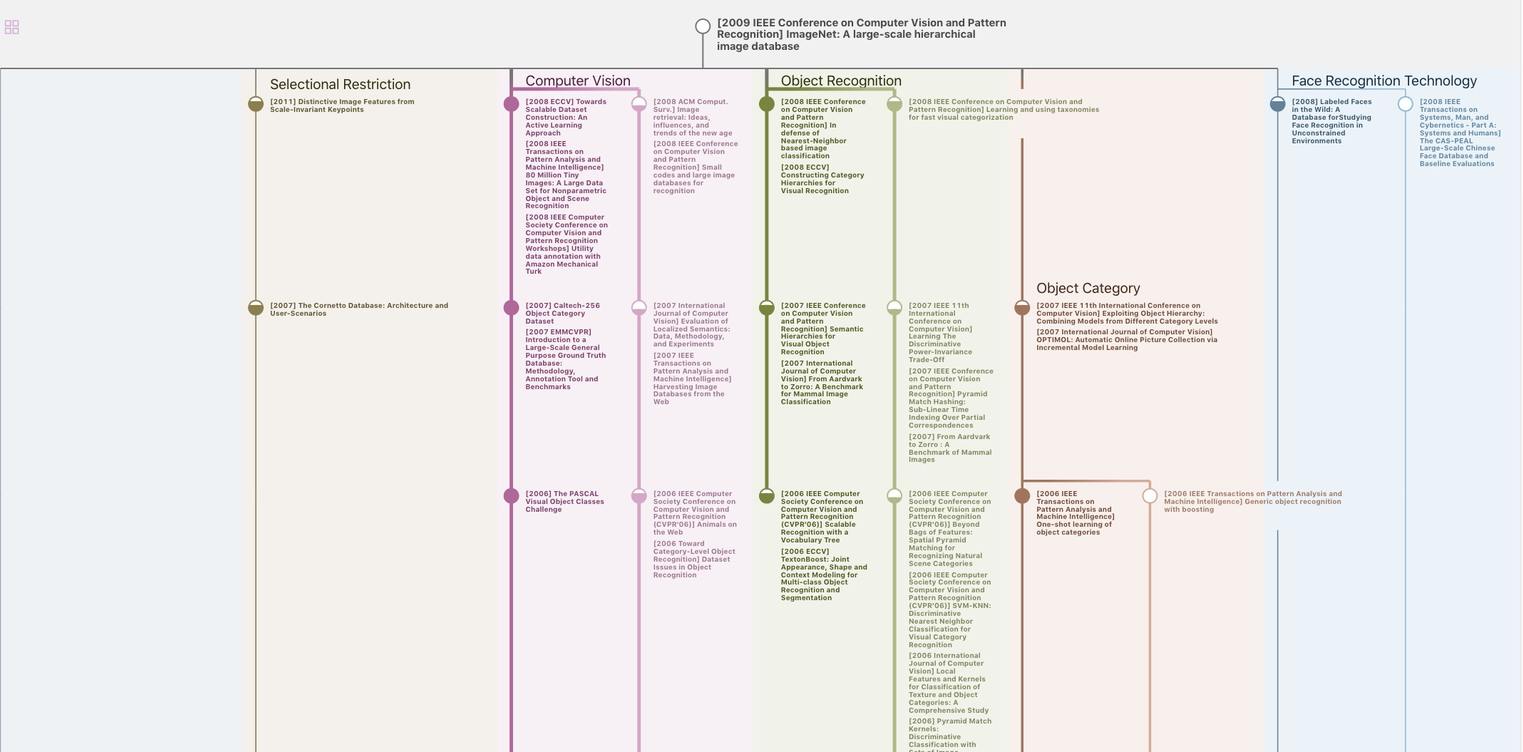
生成溯源树,研究论文发展脉络
Chat Paper
正在生成论文摘要