GTMNet: a vision transformer with guided transmission map for single remote sensing image dehazing
Scientific Reports(2023)
摘要
Existing dehazing algorithms are not effective for remote sensing images (RSIs) with dense haze, and dehazed results are prone to over-enhancement, color distortion, and artifacts. To tackle these problems, we propose a model GTMNet based on convolutional neural networks (CNNs) and vision transformers (ViTs), combined with dark channel prior (DCP) to achieve good performance. Specifically, a spatial feature transform (SFT) layer is first used to smoothly introduce the guided transmission map (GTM) into the model, improving the ability of the network to estimate haze thickness. A strengthen-operate-subtract (SOS) boosted module is then added to refine the local features of the restored image. The framework of GTMNet is determined by adjusting the input of the SOS boosted module and the position of the SFT layer. On SateHaze1k dataset, we compare GTMNet with several classical dehazing algorithms. The results show that on sub-datasets of Moderate Fog and Thick Fog, the PSNR and SSIM of GTMNet-B are comparable to that of the state-of-the-art model Dehazeformer-L, with only 0.1 times of parameter quantity. In addition, our method is intuitively effective in improving the clarity and the details of dehazed images, which proves the usefulness and significance of using the prior GTM and the SOS boosted module in a single RSI dehazing.
更多查看译文
关键词
image dehazing,vision transformer,remote sensing,gtmnet
AI 理解论文
溯源树
样例
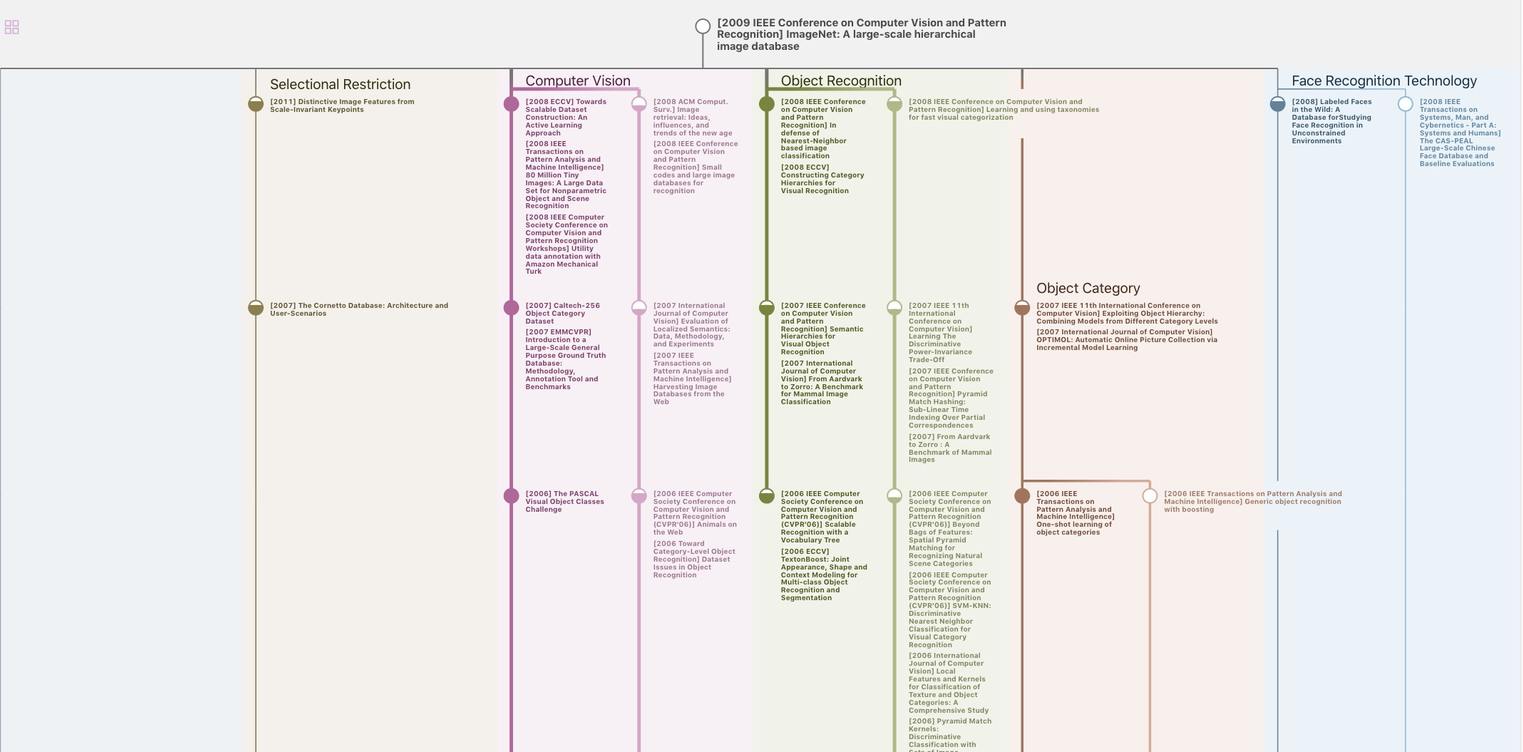
生成溯源树,研究论文发展脉络
Chat Paper
正在生成论文摘要