Weighting strategies for pairwise composite marginal likelihood estimation in case of unbalanced panels and unaccounted autoregressive structure of the errors
TRANSPORTATION RESEARCH PART B-METHODOLOGICAL(2024)
摘要
Composite Marginal Likelihood (CML) estimation and its advancements are popular ways to reduce the computational burden involved in the estimation of Multinomial Probit (MNP) models. CMLs use the product of marginal likelihoods of decision makers instead of the complete joint likelihood, reducing the numerical load. This allows for the estimation of models for larger and more complex data sets. The definition of the CML involves power weights on the marginal likelihoods that influence the statistical properties of the estimator. In this paper, we discuss how to effectively use the power weights in the cases of (1) unbalanced panel settings, where the weights help to reduce the variance of the estimator, and (2) unaccounted autoregressive structure of the errors, where the weights help to reduce the asymptotic bias of the estimator due to misspecification.
更多查看译文
关键词
Probit modelling,Composite marginal likelihood,Weighting,Unbalanced panel data,Unaccounted autoregressive error process,Efficiency
AI 理解论文
溯源树
样例
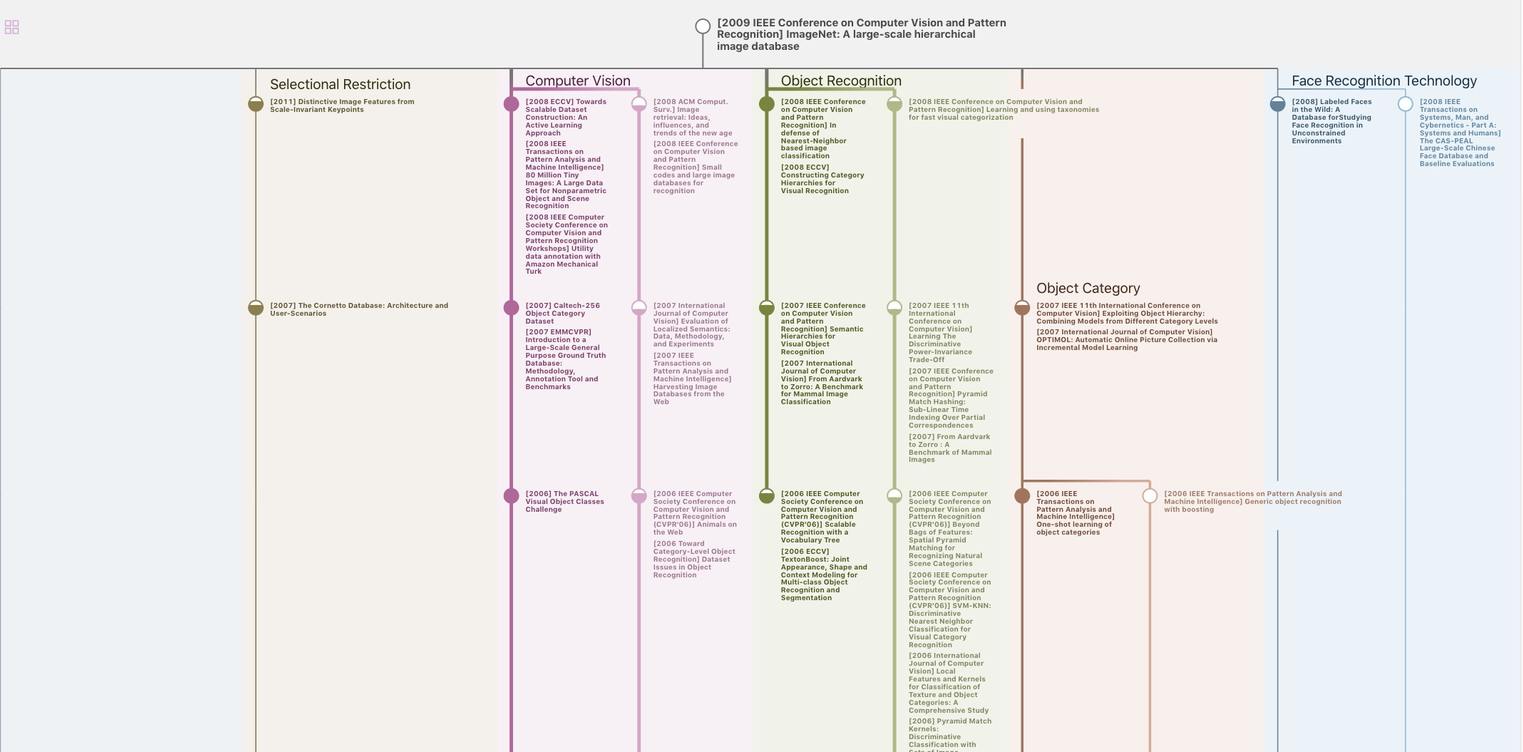
生成溯源树,研究论文发展脉络
Chat Paper
正在生成论文摘要