Dynamic contrast enhanced (DCE) MRI estimation of vascular parameters using knowledge-based adaptive models
Scientific Reports(2023)
摘要
We introduce and validate four adaptive models (AMs) to perform a physiologically based Nested-Model-Selection (NMS) estimation of such microvascular parameters as forward volumetric transfer constant, K trans , plasma volume fraction, v p , and extravascular, extracellular space, v e , directly from Dynamic Contrast-Enhanced (DCE) MRI raw information without the need for an Arterial-Input Function (AIF). In sixty-six immune-compromised-RNU rats implanted with human U-251 cancer cells, DCE-MRI studies estimated pharmacokinetic (PK) parameters using a group-averaged radiological AIF and an extended Patlak-based NMS paradigm. One-hundred-ninety features extracted from raw DCE-MRI information were used to construct and validate (nested-cross-validation, NCV) four AMs for estimation of model-based regions and their three PK parameters. An NMS-based a priori knowledge was used to fine-tune the AMs to improve their performance. Compared to the conventional analysis, AMs produced stable maps of vascular parameters and nested-model regions less impacted by AIF-dispersion. The performance (Correlation coefficient and Adjusted R-squared for NCV test cohorts) of the AMs were: 0.914/0.834, 0.825/0.720, 0.938/0.880, and 0.890/0.792 for predictions of nested model regions, v p , K trans , and v e , respectively. This study demonstrates an application of AMs that quickens and improves DCE-MRI based quantification of microvasculature properties of tumors and normal tissues relative to conventional approaches.
更多查看译文
关键词
Cancer,Cancer imaging,Computational science,Mathematics and computing,Statistics,Science,Humanities and Social Sciences,multidisciplinary
AI 理解论文
溯源树
样例
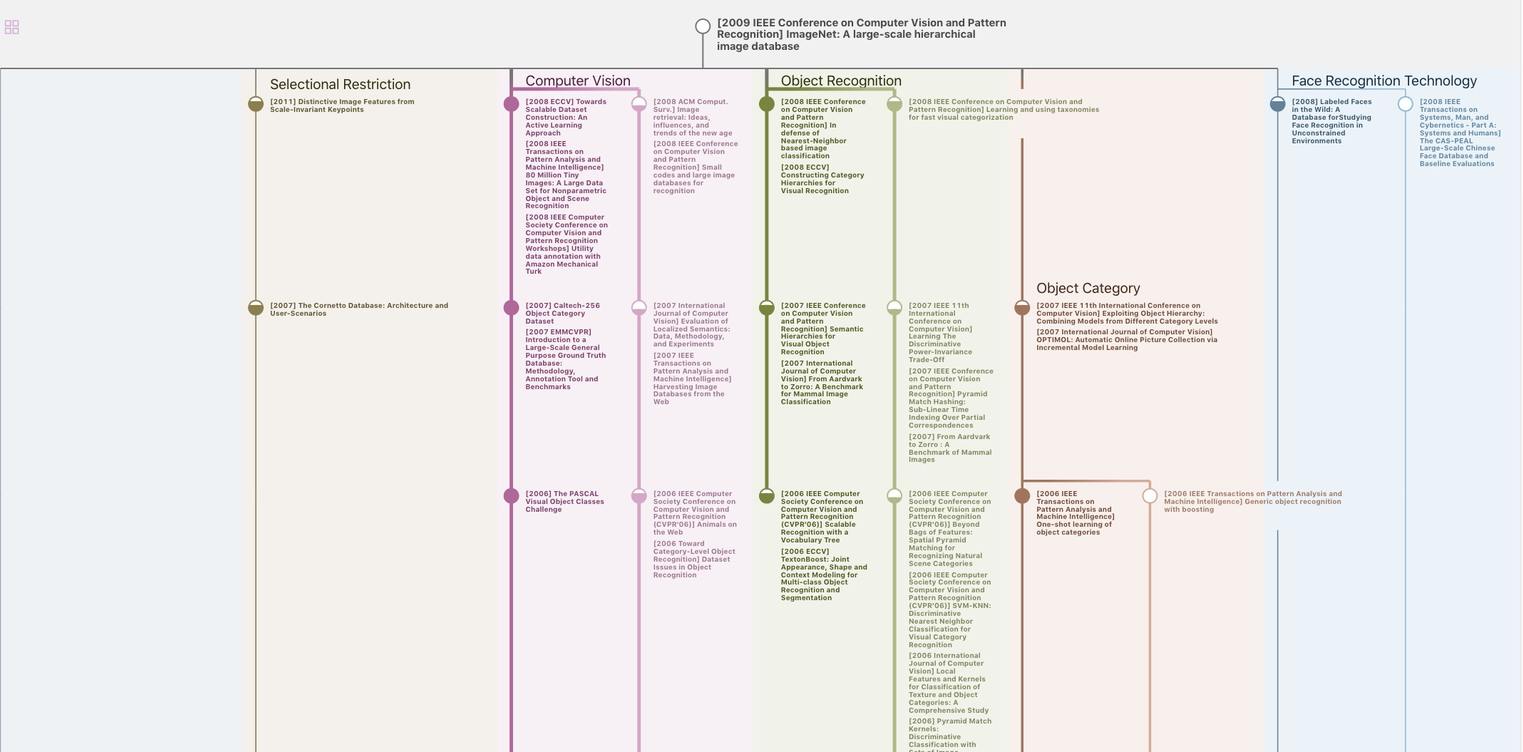
生成溯源树,研究论文发展脉络
Chat Paper
正在生成论文摘要