Fault Diagnosis of Wind Turbine Bearing on SATLBO-MLP
2022 China Automation Congress (CAC)(2022)
摘要
The vibration signal of fan bearing is easy to be disturbed, and the traditional model has many undetermined parameters or complex structure, which leads to the poor noise resistance and low accuracy of fault diagnosis. This paper presents a fault diagnosis method for fan bearings based on self-adaptive teaching-learning-based optimization multi-layer perceptron (SATLBO-MLP). Firstly, fast independent component analysis (FastICA) based on kurtosis index is used to enhance the signal features. Then the mel-cepstral coefficient (MFCC) features of the preprocessed signal are extracted. Finally, SATLBO is designed to optimize the MLP, which is used as the recognition model. In order to verify the effectiveness of the proposed method, this paper uses the publicly available bearing data from Case Western Reserve University. Meanwhile, the white gaussian noise is superimposed on the test set to simulate the complex working conditions. The experimental results show that the proposed method can not only enhance the bearing fault characteristics, but also accelerate the convergence of the model parameters optimization. The accuracy of the recognition model can reach 99.8% without noise interference. Even when the SNR is 30dB, the accuracy can achieve 98.97%.
更多查看译文
关键词
winds turbines,bearing,fault diagnosis,self- adaptive teaching-learning-based optimization
AI 理解论文
溯源树
样例
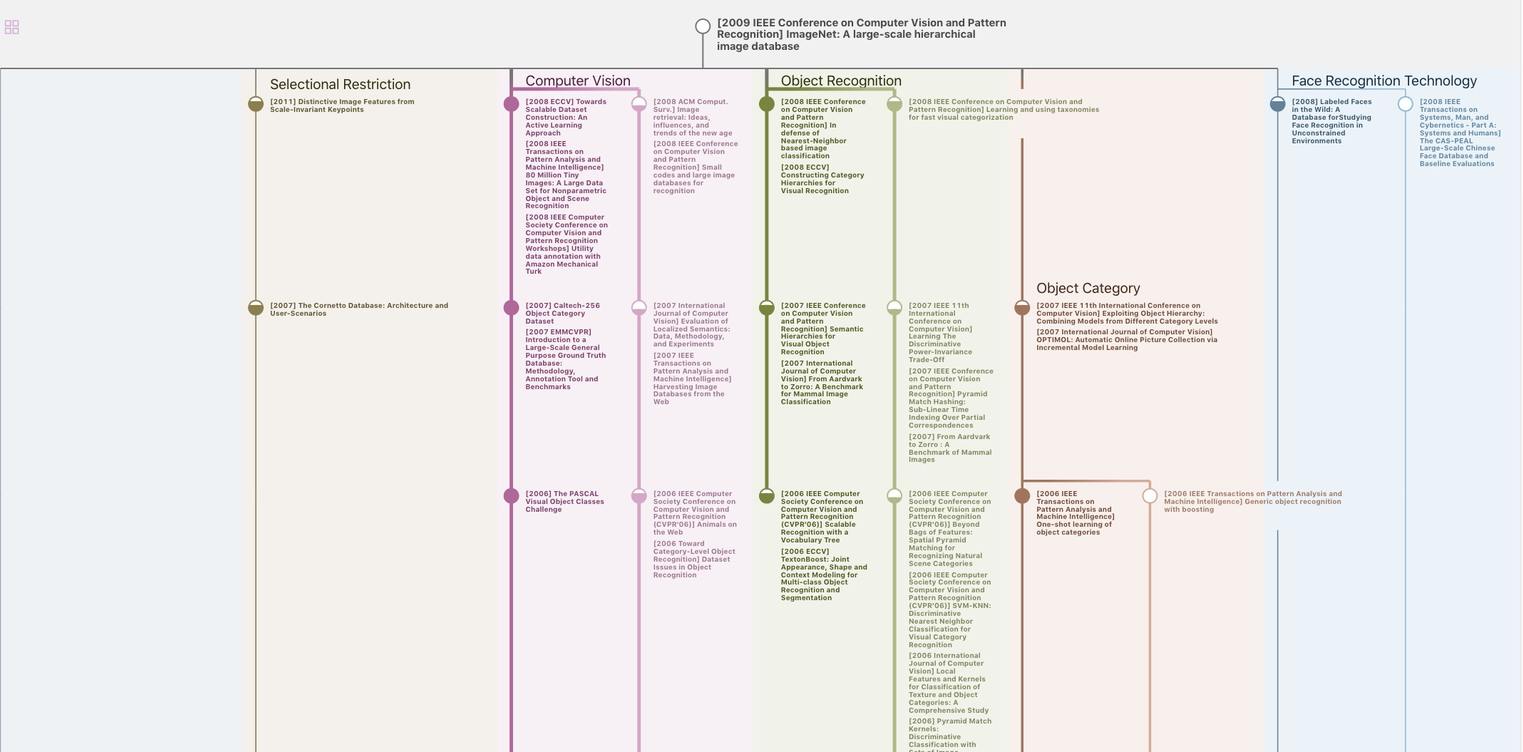
生成溯源树,研究论文发展脉络
Chat Paper
正在生成论文摘要