DEBI-NN: Distance-encoding biomorphic-informational neural networks for minimizing the number of trainable parameters.
Neural networks : the official journal of the International Neural Network Society(2023)
摘要
Modern artificial intelligence (AI) approaches mainly rely on neural network (NN) or deep NN methodologies. However, these approaches require large amounts of data to train, given, that the number of their trainable parameters has a polynomial relationship to their neuron counts. This property renders deep NNs challenging to apply in fields operating with small, albeit representative datasets such as healthcare. In this paper, we propose a novel neural network architecture which trains spatial positions of neural soma and axon pairs, where weights are calculated by axon-soma distances of connected neurons. We refer to this method as distance-encoding biomorphic-informational (DEBI) neural network. This concept significantly minimizes the number of trainable parameters compared to conventional neural networks. We demonstrate that DEBI models can yield comparable predictive performance in tabular and imaging datasets, where they require a fraction of trainable parameters compared to conventional NNs, resulting in a highly scalable solution.
更多查看译文
关键词
Biomorphic computing,Informational neural network,Distance encoding,Machine learning,Artificial intelligence,Medical AI
AI 理解论文
溯源树
样例
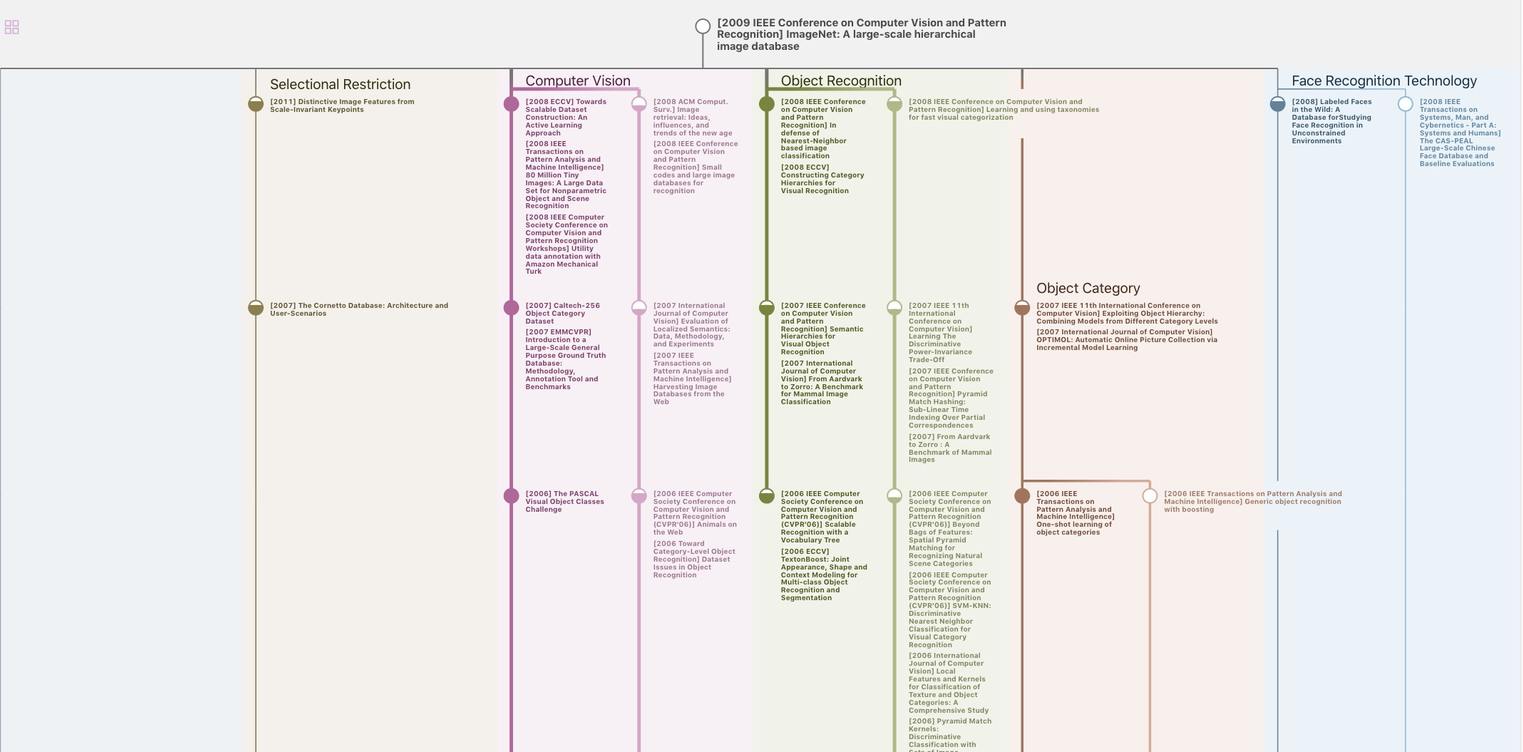
生成溯源树,研究论文发展脉络
Chat Paper
正在生成论文摘要