Lightning nowcasting with aerosol-informed machine learning and satellite-enriched dataset
Research Square (Research Square)(2023)
摘要
Abstract Fast and accurate prediction of lightning occurrence is important for protecting human health and global environment. The machine learning (ML)-based model has advantages in computation efficiency; however it suffers low accuracy due to insufficient depiction of complex lightning mechanisms and poor training dataset. To address such limitations, here we propose a novel ML-based lightning nowcast model (LightGBM) by incorporating aerosol feature to better capture the lightning mechanisms, together with the aid of enriched satellite observations from Geostationary Lightning Mapper (GLM). The well-trained LightGBM model has successfully predicted spatially continuous (0.25° by 0.25°) and hourly level lightning occurrence over summertime Contiguous United Stated (CONUS) with improved performance (accuracy = 94.3%, POD = 75.0%, FAR = 38.1%, PRC-AUC = 0.727) from competitive baselines (POD < 60%, FAR > 40%). Besides enriched dataset, such improved performance is also associated with the inclusion of aerosol feature (with > 10% enhancement on correct rejection rate when demand for POD exceeds 75%) which is proven to be essential for predicting lightning but overlooked in previous studies. Furthermore, the model reveals the contribution of aerosol composition and loading to the formation of lightning, suggesting high loading of aerosols compositing of sulfate and organic compounds tend to enhance the lightning while black carbon inhibits it. These findings are consistent with the current scientific knowledge and demonstrate the great potentials to explore the complex mechanisms of lightning associated with aerosols.
更多查看译文
关键词
dataset,machine learning,aerosol-informed,satellite-enriched
AI 理解论文
溯源树
样例
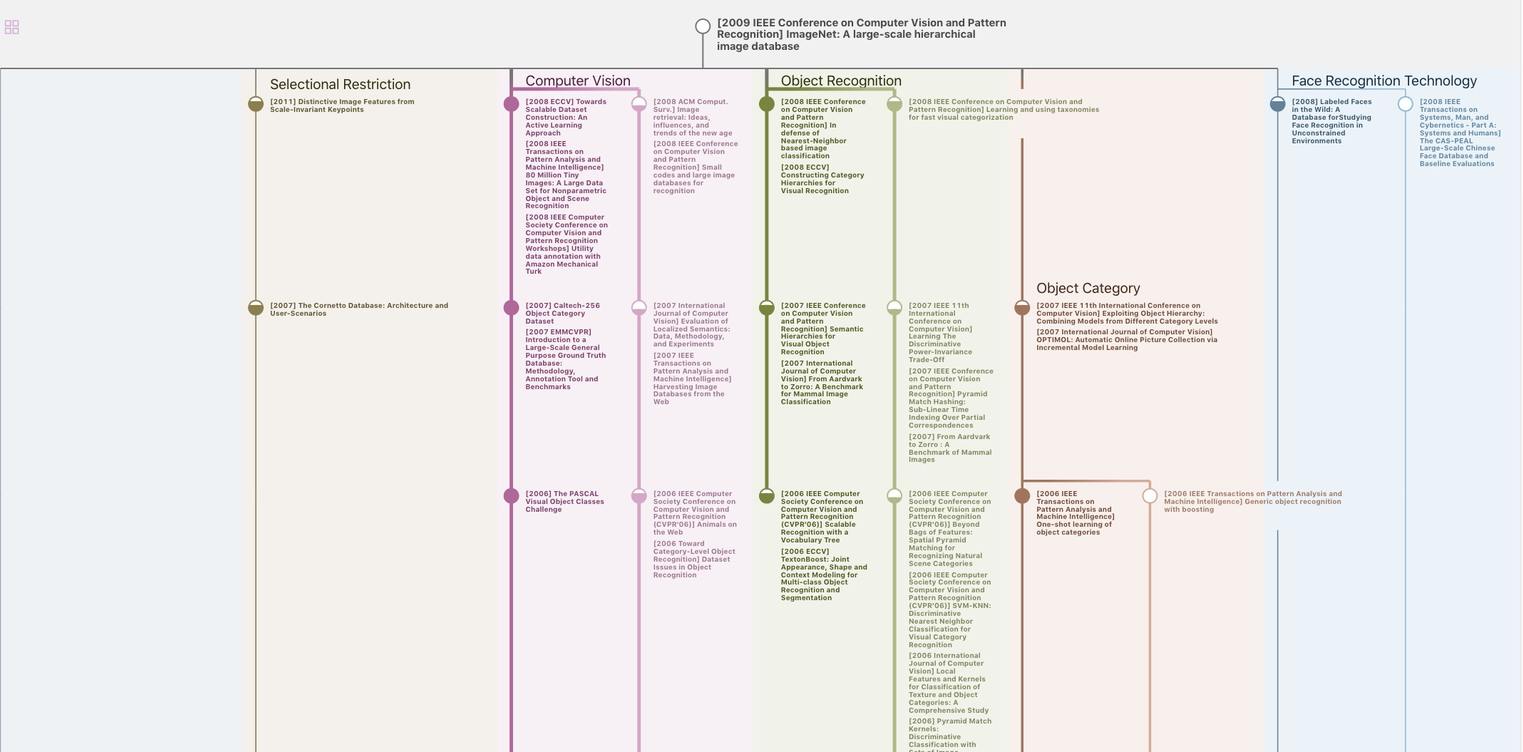
生成溯源树,研究论文发展脉络
Chat Paper
正在生成论文摘要