A multiview clustering framework for detecting deceptive reviews.
J. Comput. Secur.(2024)
摘要
Online reviews, which play a key role in the ecosystem of nowadays business, have been the primary source of consumer opinions. Due to their importance, professional review writing services are employed for paid reviews and even being exploited to conduct opinion spam. Posting deceptive reviews could mislead customers, yield significant benefits or losses to service vendors, and erode confidence in the entire online purchasing ecosystem. In this paper, we ferret out deceptive reviews originated from professional review writing services. We do so even when reviewers leverage a number of pseudonymous identities to avoid the detection. To unveil the pseudonymous identities associated with deceptive reviewers, we leverage the multiview clustering method. This enables us to characterize the writing style of reviewers (deceptive vs normal) and cluster the reviewers based on their writing style. Furthermore, we explore different neural network models to model the writing style of deceptive reviews. We select the best performing neural network to generate the representation of reviews. We validate the effectiveness of the multiview clustering framework using real-world Amazon review data under different experimental scenarios. Our results show that our approach outperforms previous research. We further demonstrate its superiority through a large-scale case study based on publicly available Amazon datasets.
更多查看译文
关键词
Multiview clustering,deceptive review detection,neural network
AI 理解论文
溯源树
样例
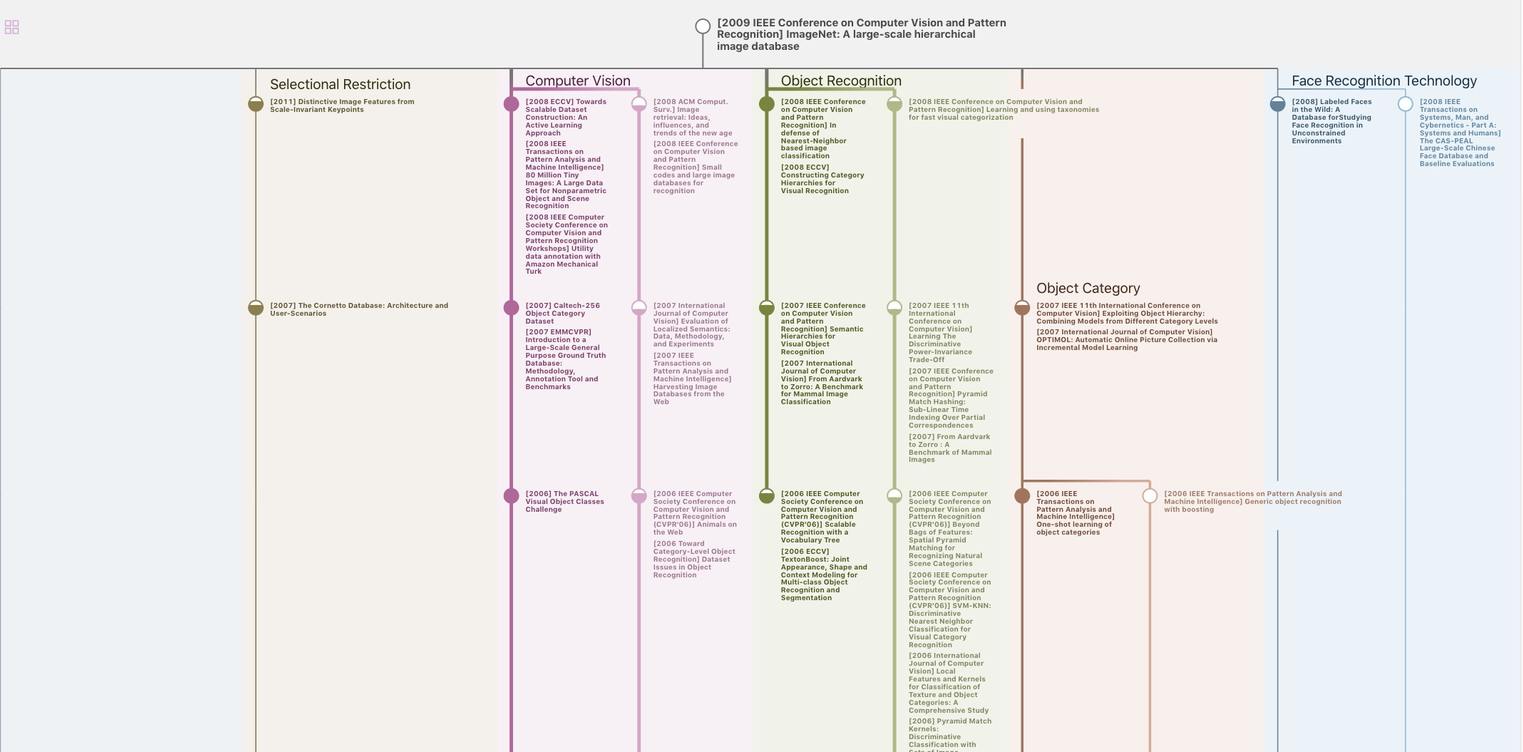
生成溯源树,研究论文发展脉络
Chat Paper
正在生成论文摘要