Ranking and Selection in Large-Scale Inference of Heteroscedastic Units
arXiv (Cornell University)(2023)
摘要
The allocation of limited resources to a large number of potential candidates presents a pervasive challenge. In the context of ranking and selecting top candidates from heteroscedastic units, conventional methods often result in over-representations of subpopulations, and this issue is further exacerbated in large-scale settings where thousands of candidates are considered simultaneously. To address this challenge, we propose a new multiple comparison framework that incorporates a modified power notion to prioritize the selection of important effects and employs a novel ranking metric to assess the relative importance of units. We develop both oracle and data-driven algorithms, and demonstrate their effectiveness in controlling the error rates and achieving optimality. We evaluate the numerical performance of our proposed method using simulated and real data. The results show that our framework enables a more balanced selection of effects that are both statistically significant and practically important, and results in an objective and relevant ranking scheme that is well-suited to practical scenarios.
更多查看译文
关键词
ranking,selection,large-scale
AI 理解论文
溯源树
样例
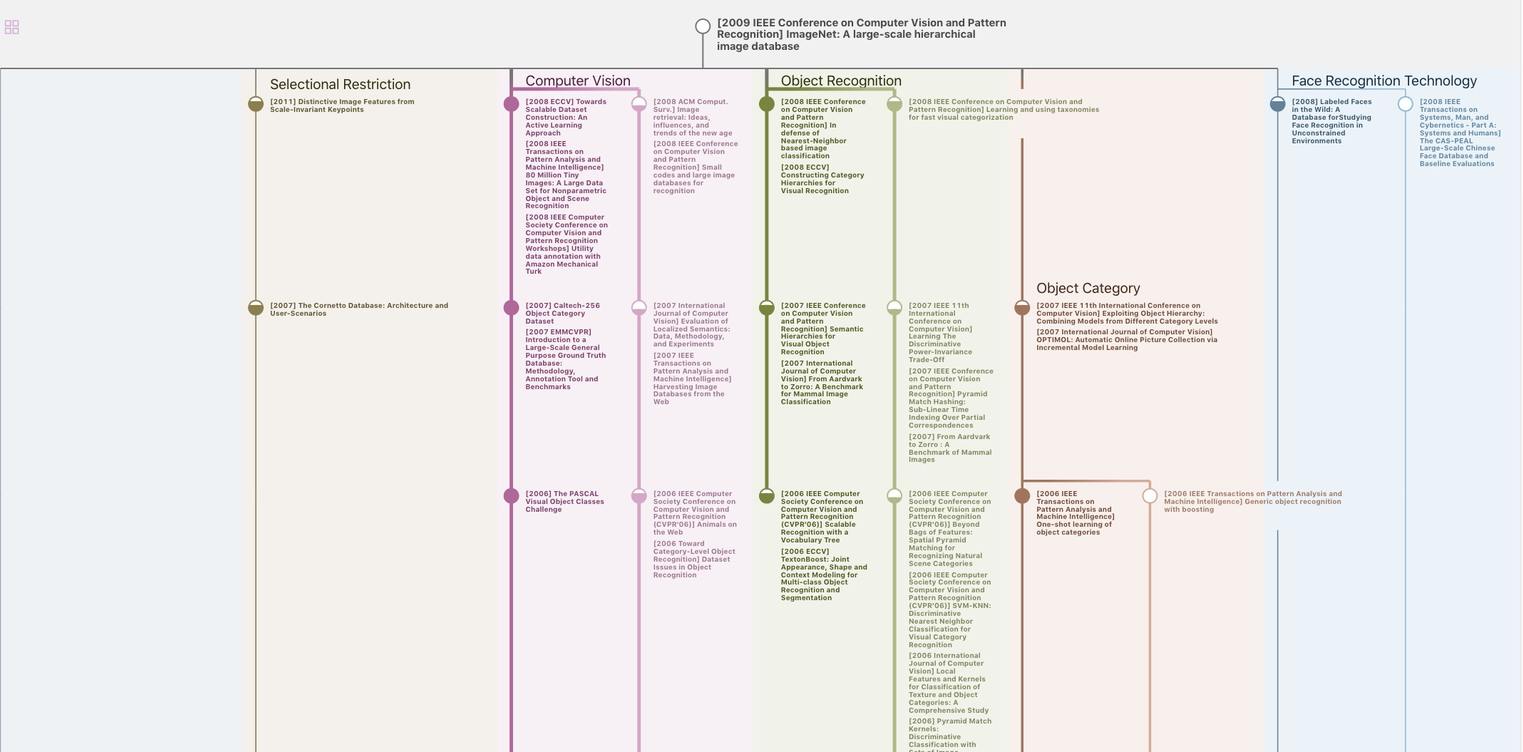
生成溯源树,研究论文发展脉络
Chat Paper
正在生成论文摘要