A generative artificial intelligence framework based on a molecular diffusion model for the design of metal-organic frameworks for carbon capture
Communications Chemistry(2024)
摘要
Metal-organic frameworks (MOFs) exhibit great promise for CO 2 capture. However, finding the best performing materials poses computational and experimental grand challenges in view of the vast chemical space of potential building blocks. Here, we introduce GHP-MOFassemble, a generative artificial intelligence (AI), high performance framework for the rational and accelerated design of MOFs with high CO 2 adsorption capacity and synthesizable linkers. GHP-MOFassemble generates novel linkers, assembled with one of three pre-selected metal nodes (Cu paddlewheel, Zn paddlewheel, Zn tetramer) into MOFs in a primitive cubic topology. GHP-MOFassemble screens and validates AI-generated MOFs for uniqueness, synthesizability, structural validity, uses molecular dynamics simulations to study their stability and chemical consistency, and crystal graph neural networks and Grand Canonical Monte Carlo simulations to quantify their CO 2 adsorption capacities. We present the top six AI-generated MOFs with CO 2 capacities greater than 2m mol g −1 , i.e., higher than 96.9% of structures in the hypothetical MOF dataset.
更多查看译文
AI 理解论文
溯源树
样例
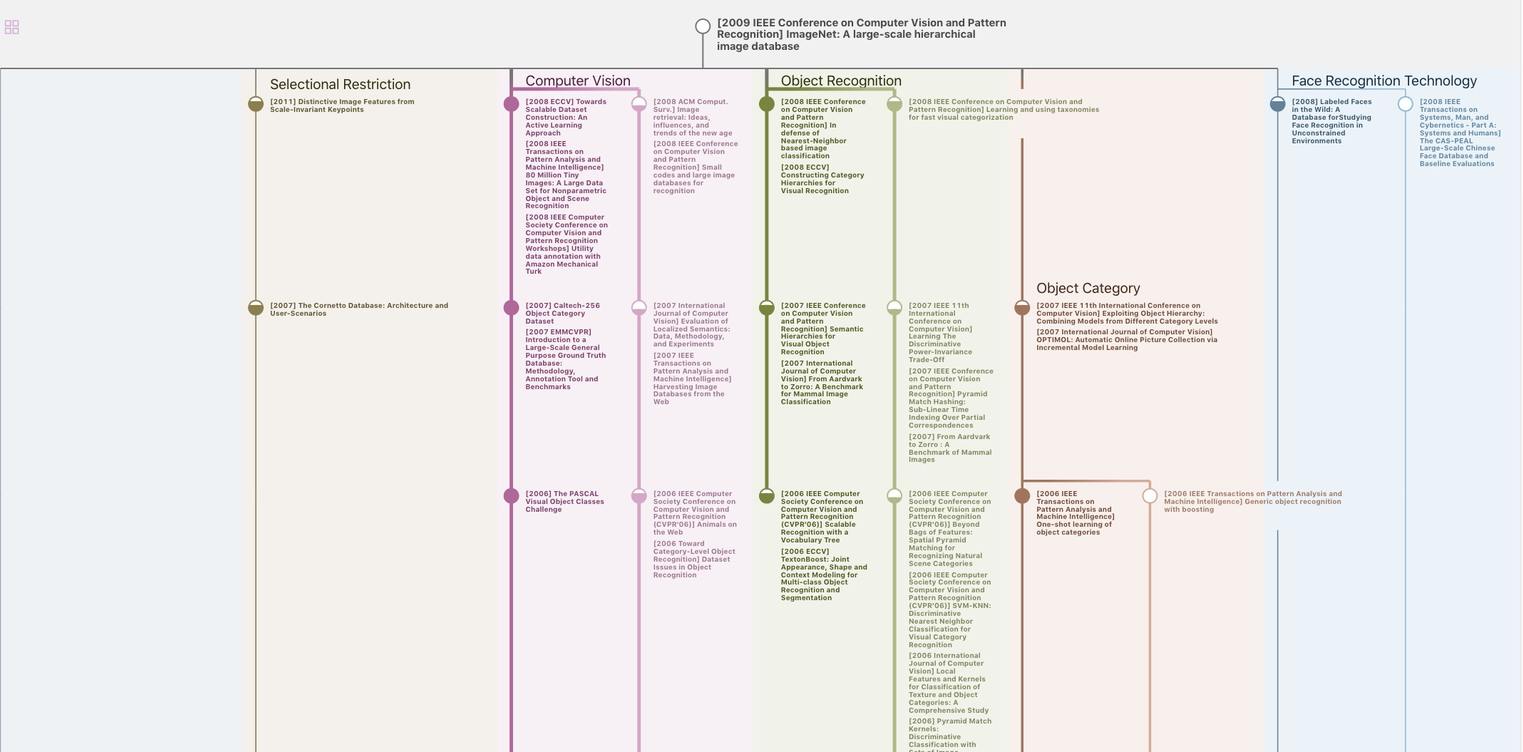
生成溯源树,研究论文发展脉络
Chat Paper
正在生成论文摘要