The generalized hyperbolic family and automatic model selection through the multiple-choice LASSO
STATISTICAL ANALYSIS AND DATA MINING(2023)
摘要
We revisit the generalized hyperbolic (GH) distribution and its nested models. These include widely used parametric choices like the multivariate normal, skew-t, Laplace, and several others. We also introduce the multiple-choice LASSO, a novel penalized method for choosing among alternative constraints on the same parameter. A hierarchical multiple-choice Least Absolute Shrinkage and Selection Operator (LASSO) penalized likelihood is optimized to perform simultaneous model selection and inference within the GH family. We illustrate our approach through a simulation study and a real data example. The methodology proposed in this paper has been implemented in R functions which are available as supplementary material.
更多查看译文
关键词
EM algorithm,generalized hyperbolic distribution,kurtosis,penalized likelihood,skewness
AI 理解论文
溯源树
样例
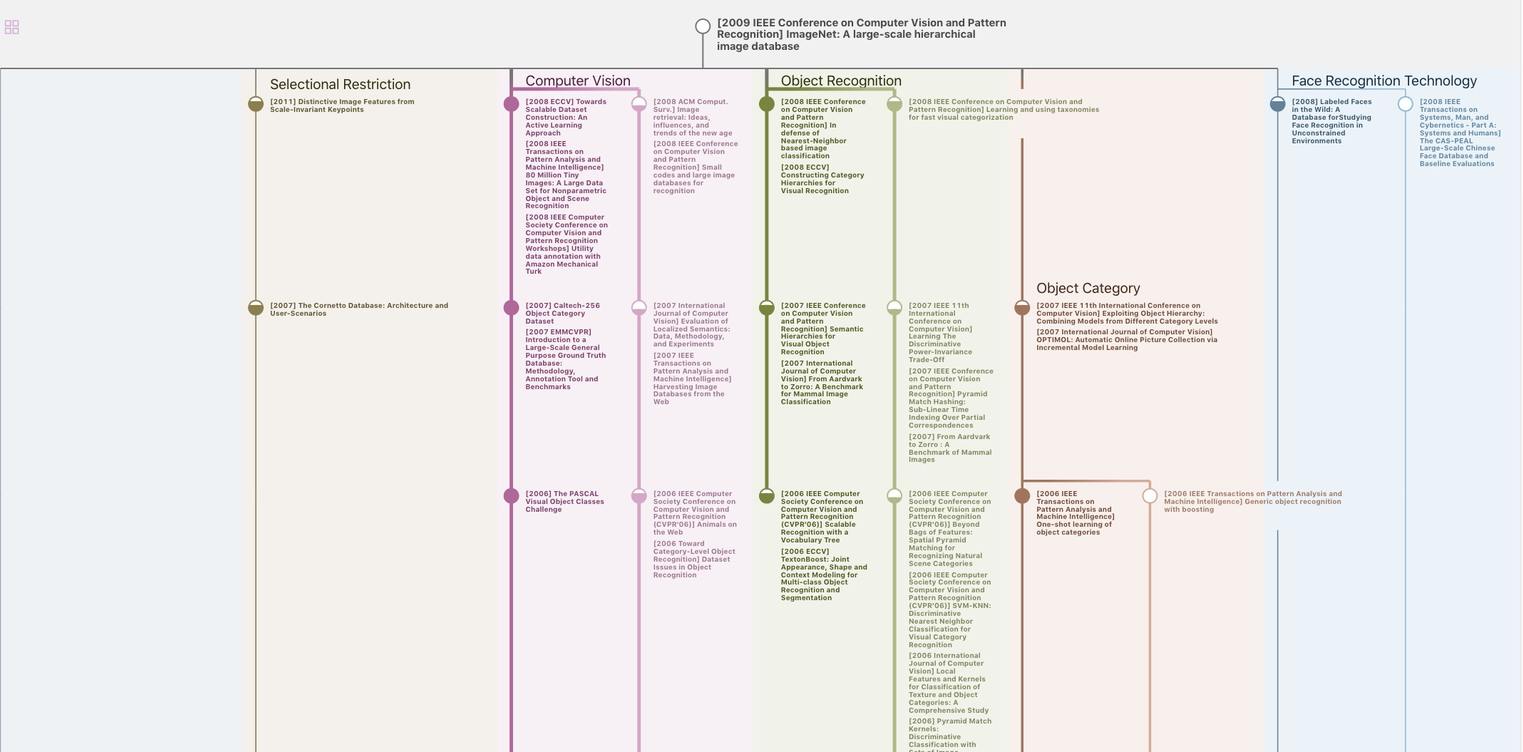
生成溯源树,研究论文发展脉络
Chat Paper
正在生成论文摘要