When to Use Efficient Self Attention? Profiling Text, Speech and Image Transformer Variants
arXiv (Cornell University)(2023)
摘要
We present the first unified study of the efficiency of self-attention-based Transformer variants spanning text, speech and vision. We identify input length thresholds (tipping points) at which efficient Transformer variants become more efficient than vanilla models, using a variety of efficiency metrics (latency, throughput, and memory). To conduct this analysis for speech, we introduce L-HuBERT, a novel local-attention variant of a self-supervised speech model. We observe that these thresholds are (a) much higher than typical dataset sequence lengths and (b) dependent on the metric and modality, showing that choosing the right model depends on modality, task type (long-form vs. typical context) and resource constraints (time vs. memory). By visualising the breakdown of the computational costs for transformer components, we also show that non-self-attention components exhibit significant computational costs. We release our profiling toolkit at https://github.com/ajd12342/profiling-transformers .
更多查看译文
关键词
efficient self attention,profiling text,speech
AI 理解论文
溯源树
样例
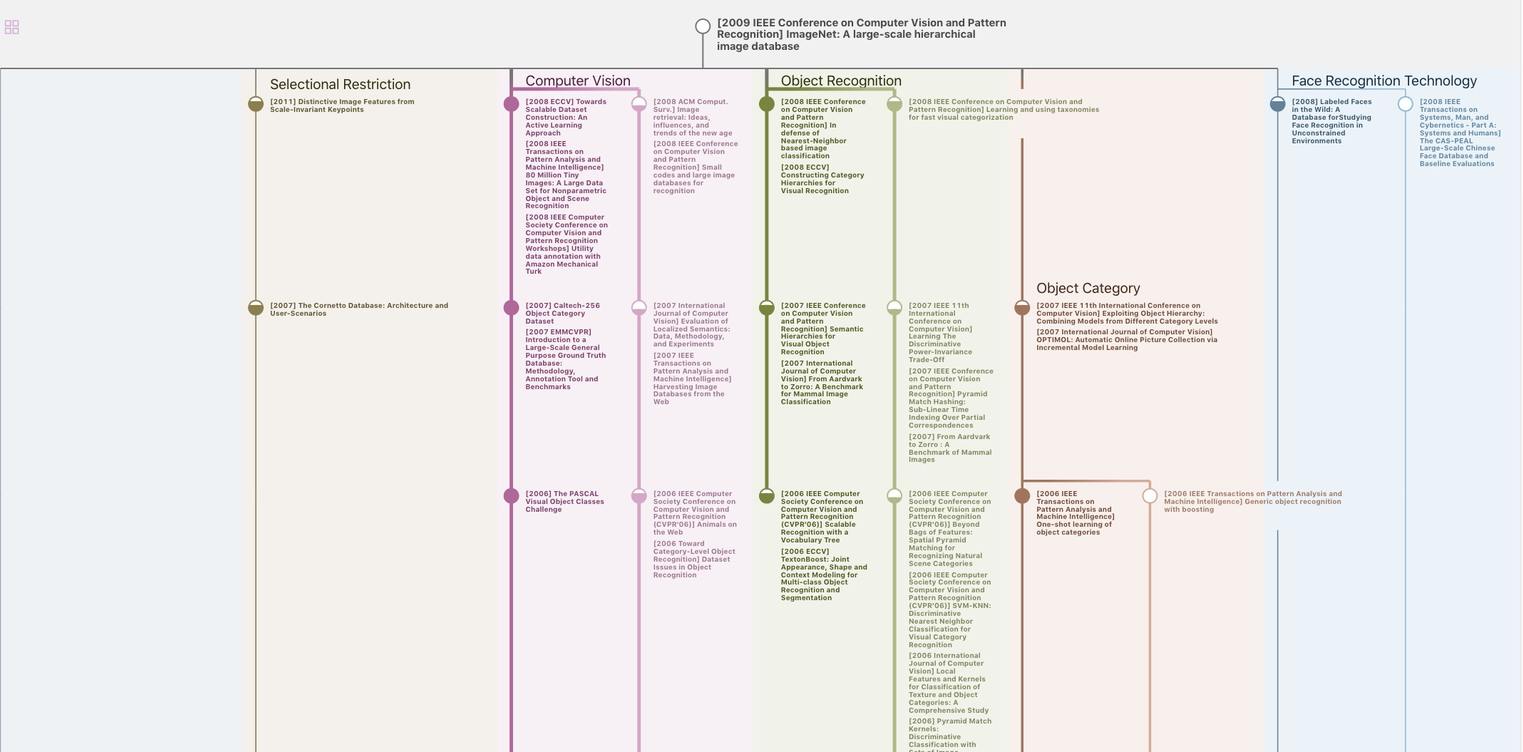
生成溯源树,研究论文发展脉络
Chat Paper
正在生成论文摘要