Label noise robust image representation learning based on supervised variational autoencoders in remote sensing
IGARSS 2023 - 2023 IEEE INTERNATIONAL GEOSCIENCE AND REMOTE SENSING SYMPOSIUM(2023)
摘要
Due to the publicly available thematic maps and crowdsourced data, remote sensing (RS) image annotations can be gathered at zero cost for training deep neural networks (DNNs). However, such annotation sources may increase the risk of including noisy labels in training data, leading to inaccurate RS image representation learning (IRL). To address this issue, in this paper we propose a label noise robust IRL method that aims to prevent the interference of noisy labels on IRL, independently from the learning task being considered in RS. To this end, the proposed method combines a supervised variational autoencoder (SVAE) with any kind of DNN. This is achieved by defining variational generative process based on image features. This allows us to define the importance of each training sample for IRL based on the loss values acquired from the SVAE and the task head of the considered DNN. Then, the proposed method imposes lower importance to images with noisy labels, while giving higher importance to those with correct labels during IRL. Experimental results show the effectiveness of the proposed method when compared to well-known label noise robust IRL methods applied to RS images. The code of the proposed method is publicly available at https://git.tu-berlin.de/rsim/RS-IRL-SVAE.
更多查看译文
关键词
Representation learning,label noise,variational autoencoders,deep learning,remote sensing
AI 理解论文
溯源树
样例
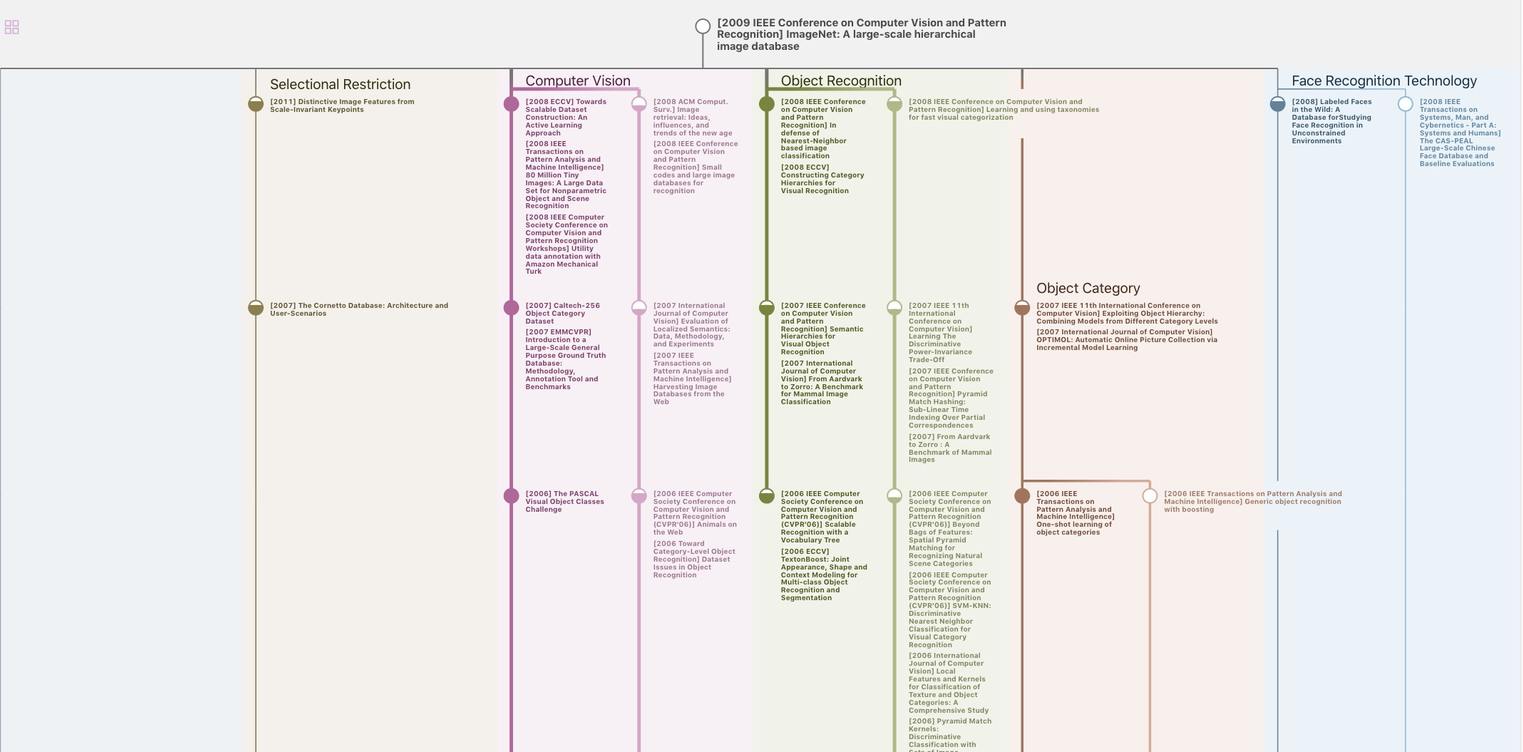
生成溯源树,研究论文发展脉络
Chat Paper
正在生成论文摘要