Federated Learning-based Vehicle Trajectory Prediction against Cyberattacks
CoRR(2023)
摘要
With the development of the Internet of Vehicles (IoV), vehicle wireless communication poses serious cybersecurity challenges. Faulty information, such as fake vehicle positions and speeds sent by surrounding vehicles, could cause vehicle collisions, traffic jams, and even casualties. Additionally, private vehicle data leakages, such as vehicle trajectory and user account information, may damage user property and security. Therefore, achieving a cyberattack-defense scheme in the IoV system with faulty data saturation is necessary. This paper proposes a Federated Learning-based Vehicle Trajectory Prediction Algorithm against Cyberattacks (FL-TP) to address the above problems. The FL-TP is intensively trained and tested using a publicly available Vehicular Reference Misbehavior (VeReMi) dataset with five types of cyberattacks: constant, constant offset, random, random offset, and eventual stop. The results show that the proposed FL-TP algorithm can improve cyberattack detection and trajectory prediction by up to 6.99% and 54.86%, respectively, under the maximum cyberattack permeability scenarios compared with benchmark methods.
更多查看译文
关键词
Internet of vehicles, federated learning, cyberattack, trajectory prediction
AI 理解论文
溯源树
样例
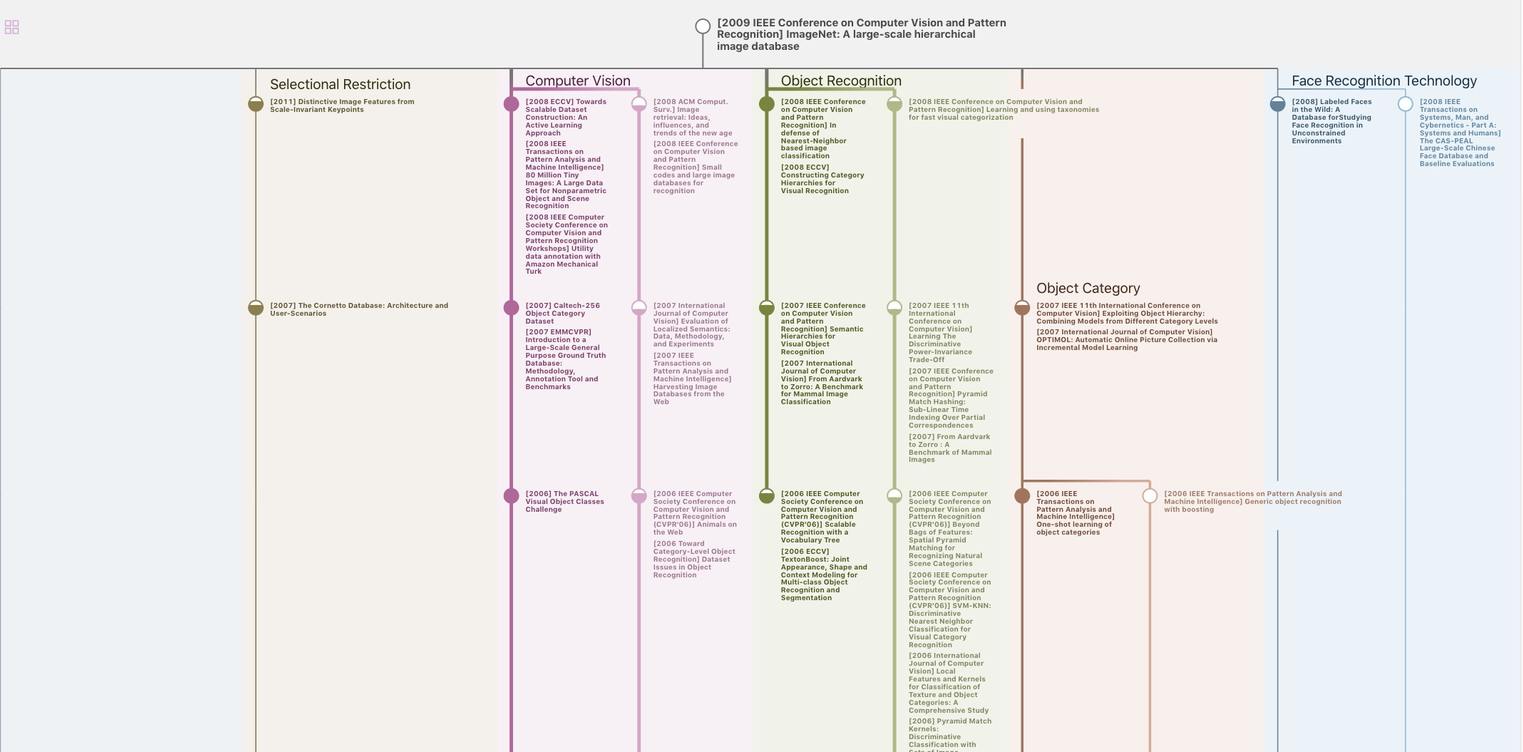
生成溯源树,研究论文发展脉络
Chat Paper
正在生成论文摘要