Generative deep-learning reveals collective variables of Fermionic systems
arXiv (Cornell University)(2023)
摘要
Complex processes ranging from protein folding to nuclear fission often follow a low-dimension reaction path parameterized in terms of a few collective variables. In nuclear theory, variables related to the shape of the nuclear density in a mean-field picture are key to describing the large amplitude collective motion of the neutrons and protons. Exploring the adiabatic energy landscape spanned by these degrees of freedom reveals the possible reaction channels while simulating the dynamics in this reduced space yields their respective probabilities. Unfortunately, this theoretical framework breaks down whenever the systems encounters a quantum phase transition with respect to the collective variables. Here we propose a generative-deep-learning algorithm capable of building new collective variables highly representative of a nuclear process while ensuring a differentiable mapping to its Fermionic wave function. Within this collective space, the nucleus can evolve continuously from one of its adiabatic quantum phase to the other at the price of crossing a potential energy barrier. This approach applies to any Fermionic system described by a single Slater determinant, which encompasses electronic systems described within the density functional theory.
更多查看译文
关键词
deep-learning deep-learning,collective variables
AI 理解论文
溯源树
样例
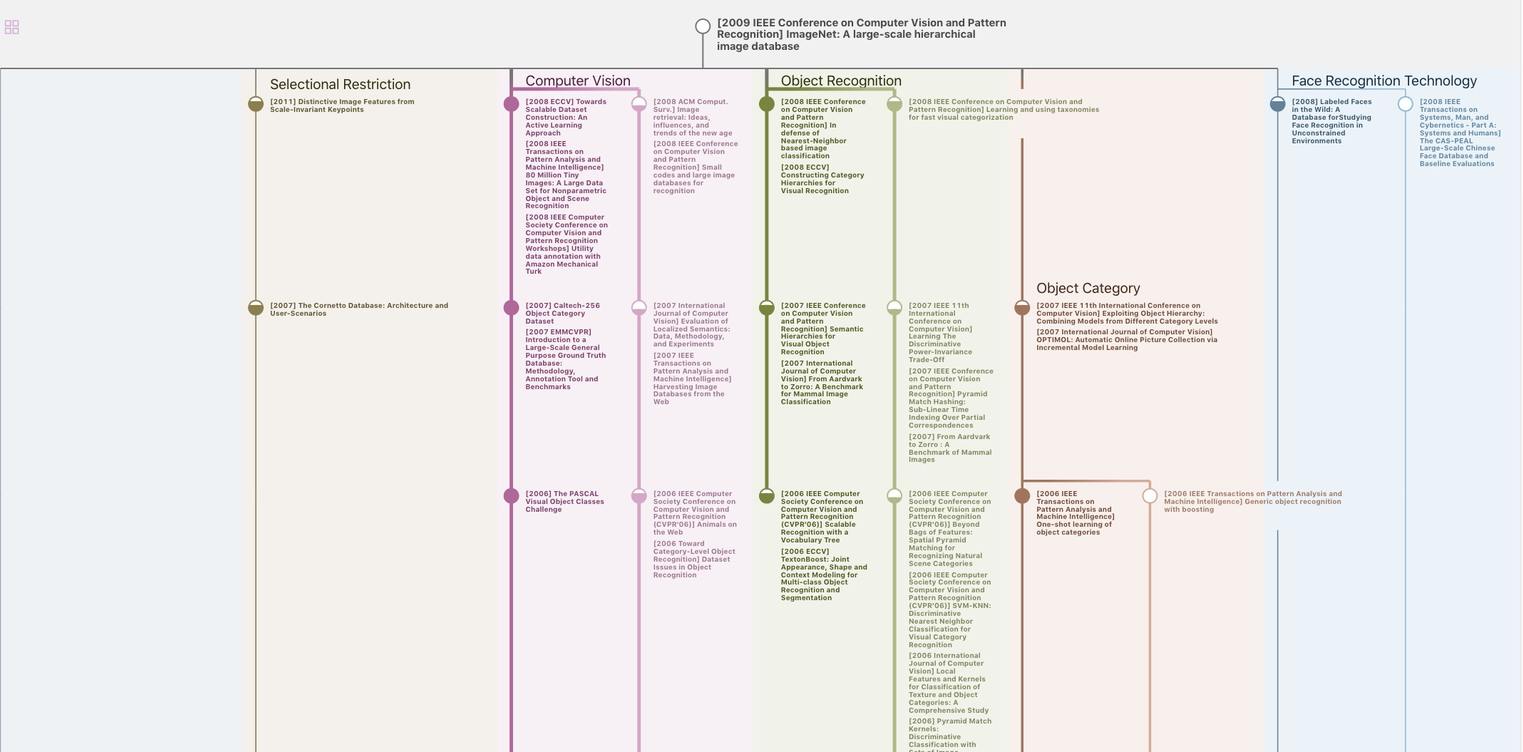
生成溯源树,研究论文发展脉络
Chat Paper
正在生成论文摘要