Uncertainty-Aware Robust Learning on Noisy Graphs
CoRR(2023)
摘要
Graph neural networks have shown impressive capabilities in solving various graph learning tasks, particularly excelling in node classification. However, their effectiveness can be hindered by the challenges arising from the widespread existence of noisy measurements associated with the topological or nodal information present in real-world graphs. These inaccuracies in observations can corrupt the crucial patterns within the graph data, ultimately resulting in undesirable performance in practical applications. To address these issues, this paper proposes a novel uncertainty-aware graph learning framework motivated by distributionally robust optimization. Specifically, we use a graph neural network-based encoder to embed the node features and find the optimal node embeddings by minimizing the worst-case risk through a minimax formulation. Such an uncertainty-aware learning process leads to improved node representations and a more robust graph predictive model that effectively mitigates the impact of uncertainty arising from data noise. Our experimental result shows that the proposed framework achieves superior predictive performance compared to the state-of-the-art baselines under various noisy settings.
更多查看译文
关键词
graphs,learning,uncertainty-aware
AI 理解论文
溯源树
样例
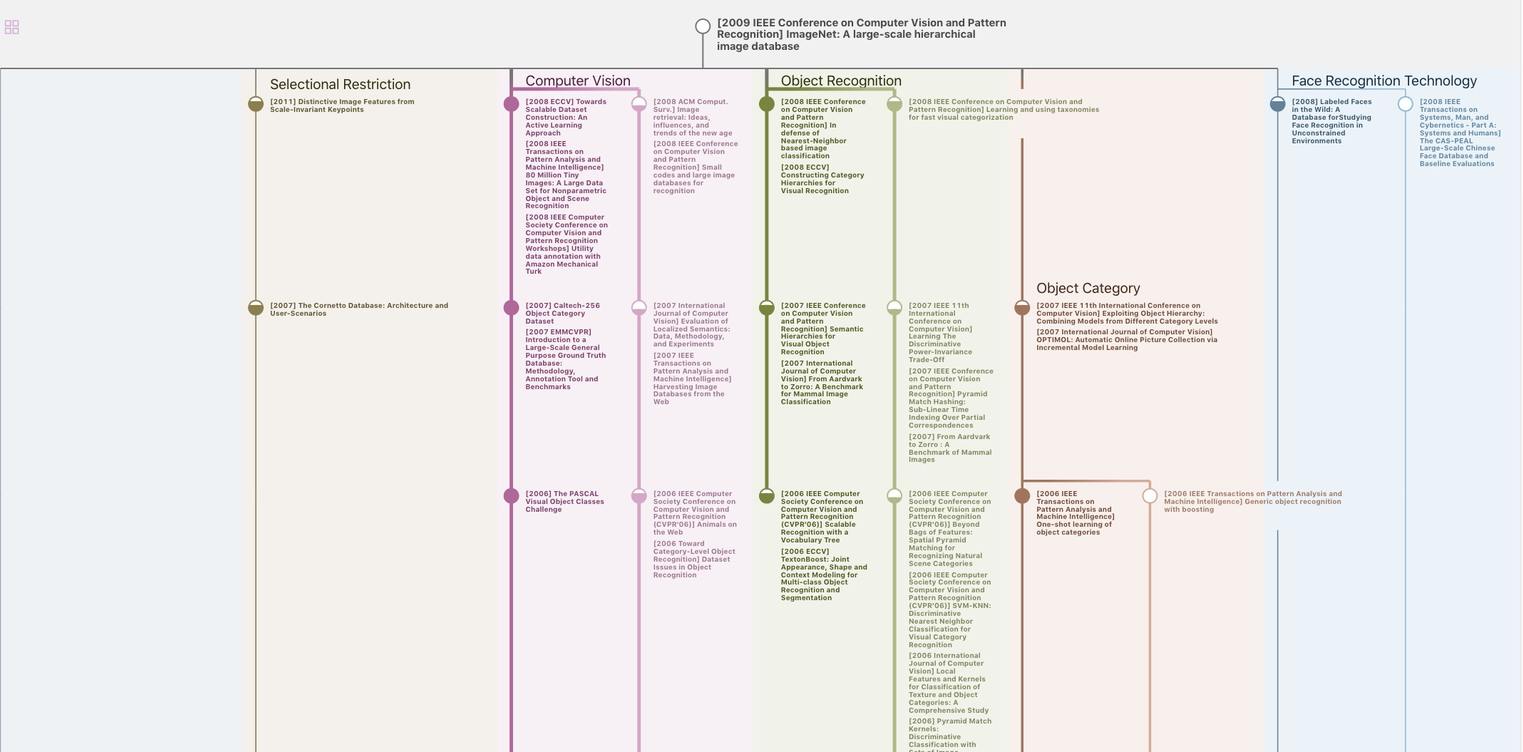
生成溯源树,研究论文发展脉络
Chat Paper
正在生成论文摘要