Invariant Node Representation Learning under Distribution Shifts with Multiple Latent Environments
ACM TRANSACTIONS ON INFORMATION SYSTEMS(2024)
摘要
Node representation learning methods, such as graph neural networks, show promising results when testing and training graph data come from the same distribution. However, the existing approaches fail to generalize under distribution shifts when the nodes reside in multiple latent environments. How to learn invariant node representations to handle distribution shifts with multiple latent environments remains unexplored. In this article, we propose a novel Invariant Node representation Learning (INL) approach capable of generating invariant node representations based on the invariant patterns under distribution shifts with multiple latent environments by leveraging the invariance principle. Specifically, we define invariant and variant patterns as ego-subgraphs of each node and identify the invariant ego-subgraphs through jointly accounting for node features and graph structures. To infer the latent environments of nodes, we propose a contrastive modularity-based graph clustering method based on the variant patterns. We further propose an invariant learning module to learn node representations that can generalize to distribution shifts. We theoretically show that our proposed method can achieve guaranteed performance under distribution shifts. Extensive experiments on both synthetic and real-world node classification benchmarks demonstrate that our method greatly outperforms state-of-the-art baselines under distribution shifts.
更多查看译文
关键词
Graph neural networks,node representation learning,distribution shift
AI 理解论文
溯源树
样例
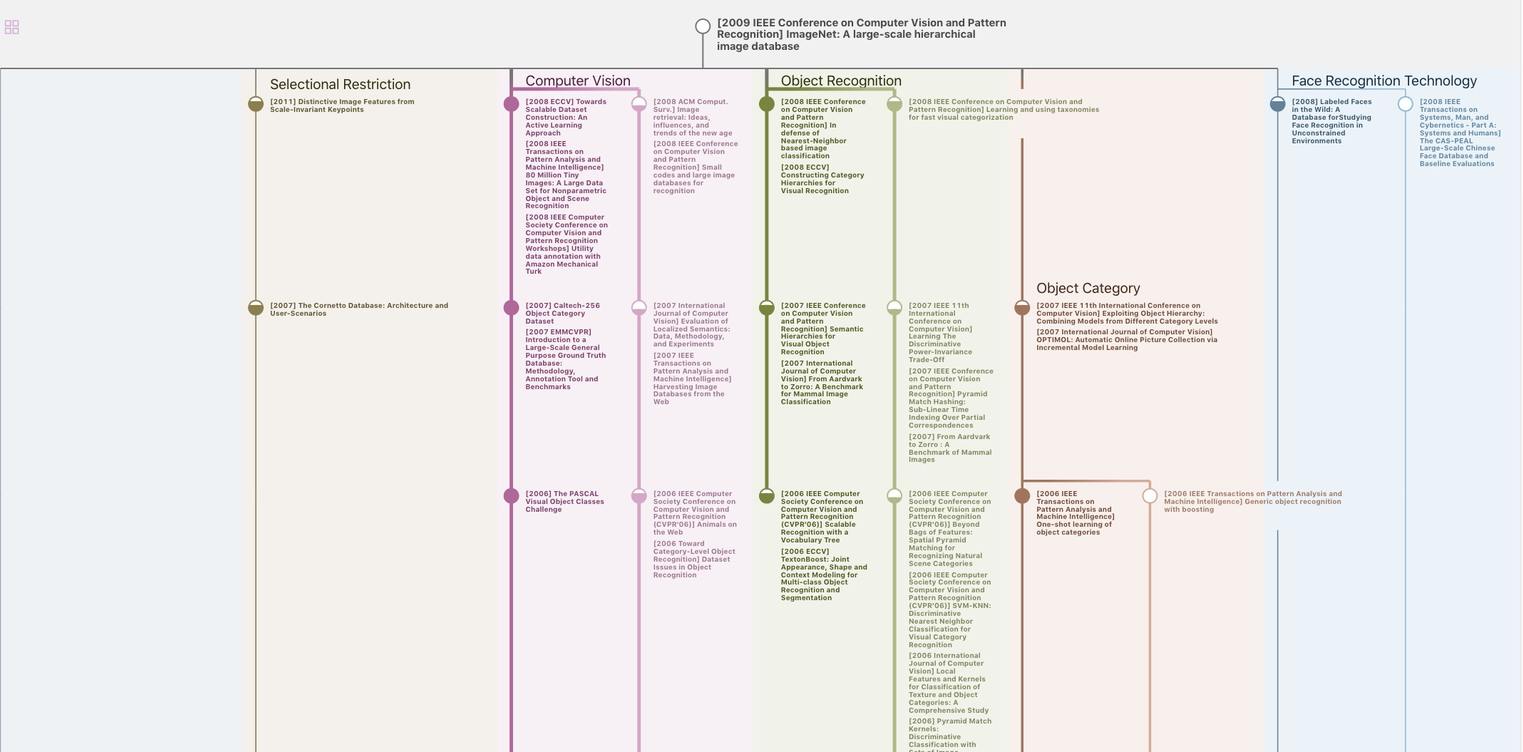
生成溯源树,研究论文发展脉络
Chat Paper
正在生成论文摘要