CAMEO: A Causal Transfer Learning Approach for Performance Optimization of Configurable Computer Systems
PROCEEDINGS OF THE 2023 ACM SYMPOSIUM ON CLOUD COMPUTING, SOCC 2023(2023)
摘要
Modern computer systems are highly configurable, with hundreds of configuration options that interact, resulting in an enormous configuration space. As a result, optimizing performance goals (e.g., latency) in such systems is challenging due to frequent uncertainties in their environments (e.g., workload fluctuations). Lately, there has been a utilization of transfer learning to tackle this issue, leveraging information obtained from configuration measurements in less expensive source environments, as opposed to the costly or sometimes impossible interventions required in the target environment. Recent empirical research showed that statistical models can perform poorly when the deployment environment changes because the behavior of certain variables in the models can change dramatically from source to target. To address this issue, we propose Cameo-a method that identifies invariant causal predictors under environmental changes, allowing the optimization process to operate in a reduced search space, leading to faster optimization of system performance. We demonstrate significant performance improvements over state-of-the-art optimization methods in MLperf deep learning systems, a video analytics pipeline, and a database system.
更多查看译文
关键词
Highly Configurable Systems,Performance Optimization,Causal Transfer Learning,Resource Constraints
AI 理解论文
溯源树
样例
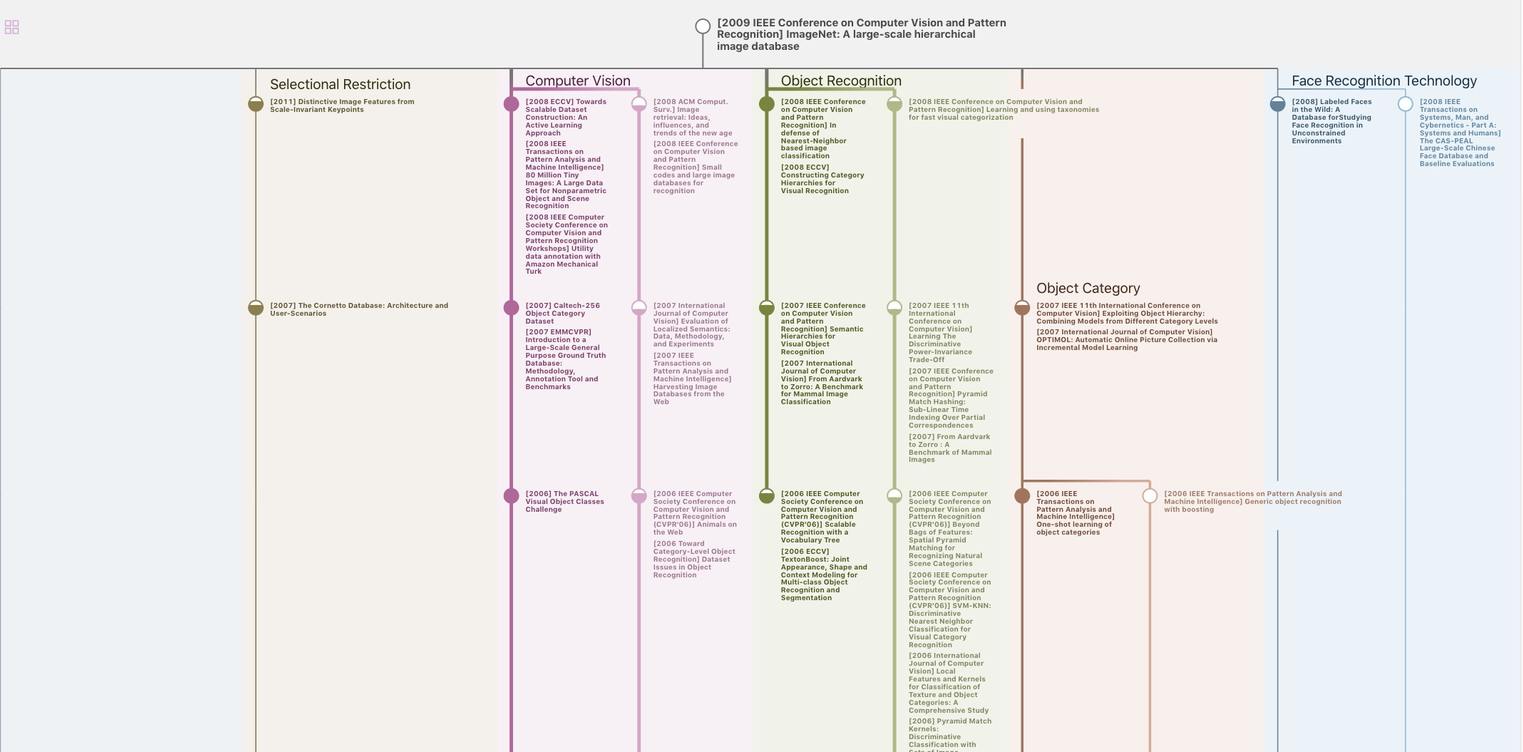
生成溯源树,研究论文发展脉络
Chat Paper
正在生成论文摘要