Unified Off-Policy Learning to Rank: a Reinforcement Learning Perspective
NeurIPS(2023)
摘要
Off-policy Learning to Rank (LTR) aims to optimize a ranker from data collected by a deployed logging policy. However, existing off-policy learning to rank methods often make strong assumptions about how users generate the click data, i.e., the click model, and hence need to tailor their methods specifically under different click models. In this paper, we unified the ranking process under general stochastic click models as a Markov Decision Process (MDP), and the optimal ranking could be learned with offline reinforcement learning (RL) directly. Building upon this, we leverage offline RL techniques for off-policy LTR and propose the Click Model-Agnostic Unified Off-policy Learning to Rank (CUOLR) method, which could be easily applied to a wide range of click models. Through a dedicated formulation of the MDP, we show that offline RL algorithms can adapt to various click models without complex debiasing techniques and prior knowledge of the model. Results on various large-scale datasets demonstrate that CUOLR consistently outperforms the state-of-the-art off-policy learning to rank algorithms while maintaining consistency and robustness under different click models.
更多查看译文
关键词
reinforcement learning,rank,off-policy
AI 理解论文
溯源树
样例
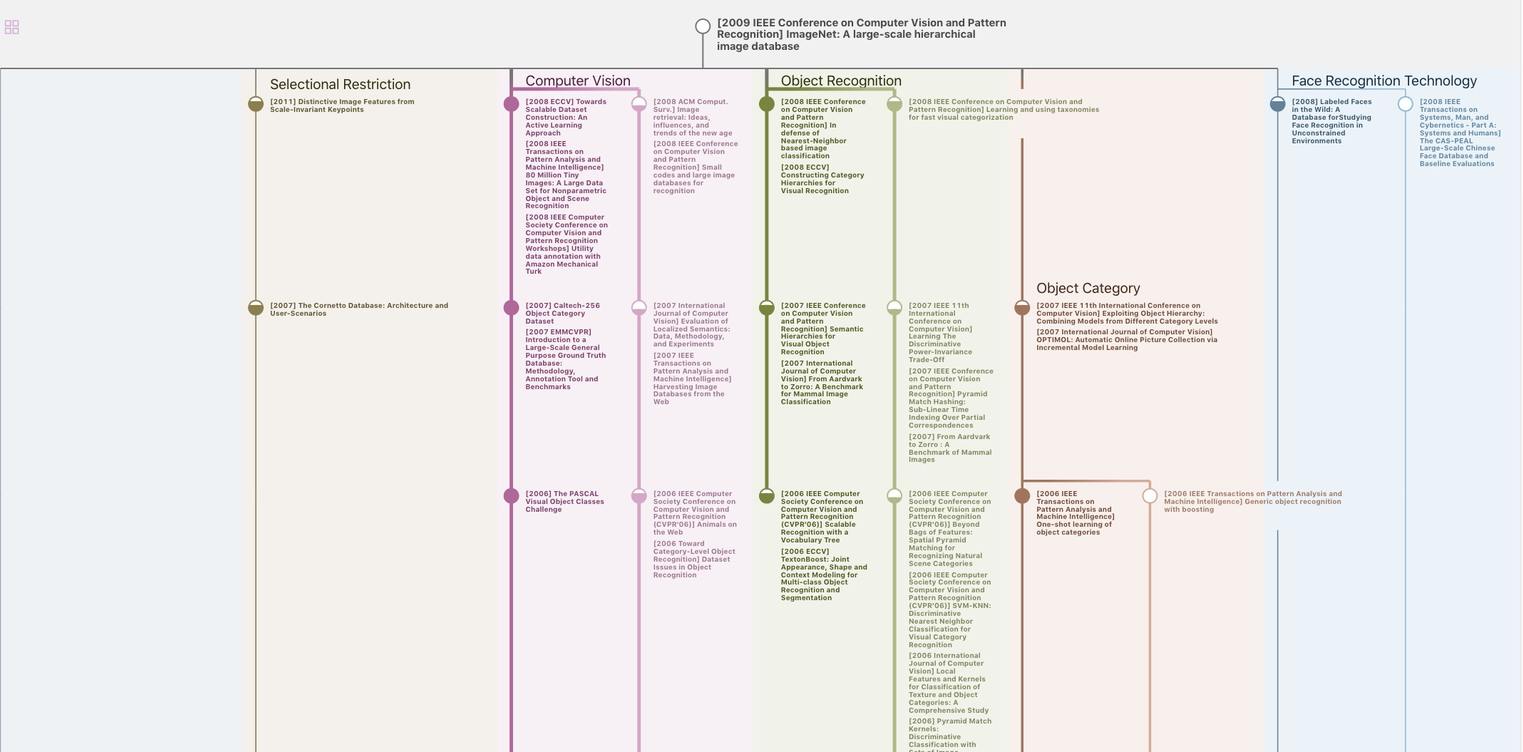
生成溯源树,研究论文发展脉络
Chat Paper
正在生成论文摘要