Faster Boundary-aware Transformer for Breast Cancer Segmentation
2023 15th International Conference on Advanced Computational Intelligence (ICACI)(2023)
摘要
In this paper, we propose an effective network, termed Faster Boundary-aware Transformer (FBAT), to tackle the critical problem in the breast cancer segmentation task: Imprecise segmentation results owing to ambiguous boundaries in medical images. Our motivation is that dear boundaries facilitate precise medical segmentation, so providing additional boundaries information will help network perform better. To do so, we introduce FBAT to comprehensively address the challenging segmentation task. Specifically, we incorporate a new boundary-wise attention block (BAB) into transformers to allow the entire network to effectively capture local details using boundary-wise prior knowledge. Meanwhile, we improve the transformer decoder’s cross-attention mechanism by generating reference points from decoder embeddings and object queries, thereby narrowing the scope of search objects and accelerating the training. We evaluate the proposed network on Breast Ultrasound Images, Breast Ultrasound Dataset B and our own Breast MRI Images datasets. FBAT achieves convincing segmentation results.
更多查看译文
关键词
Medical Image Segmentation, Deep Learning, Transformer
AI 理解论文
溯源树
样例
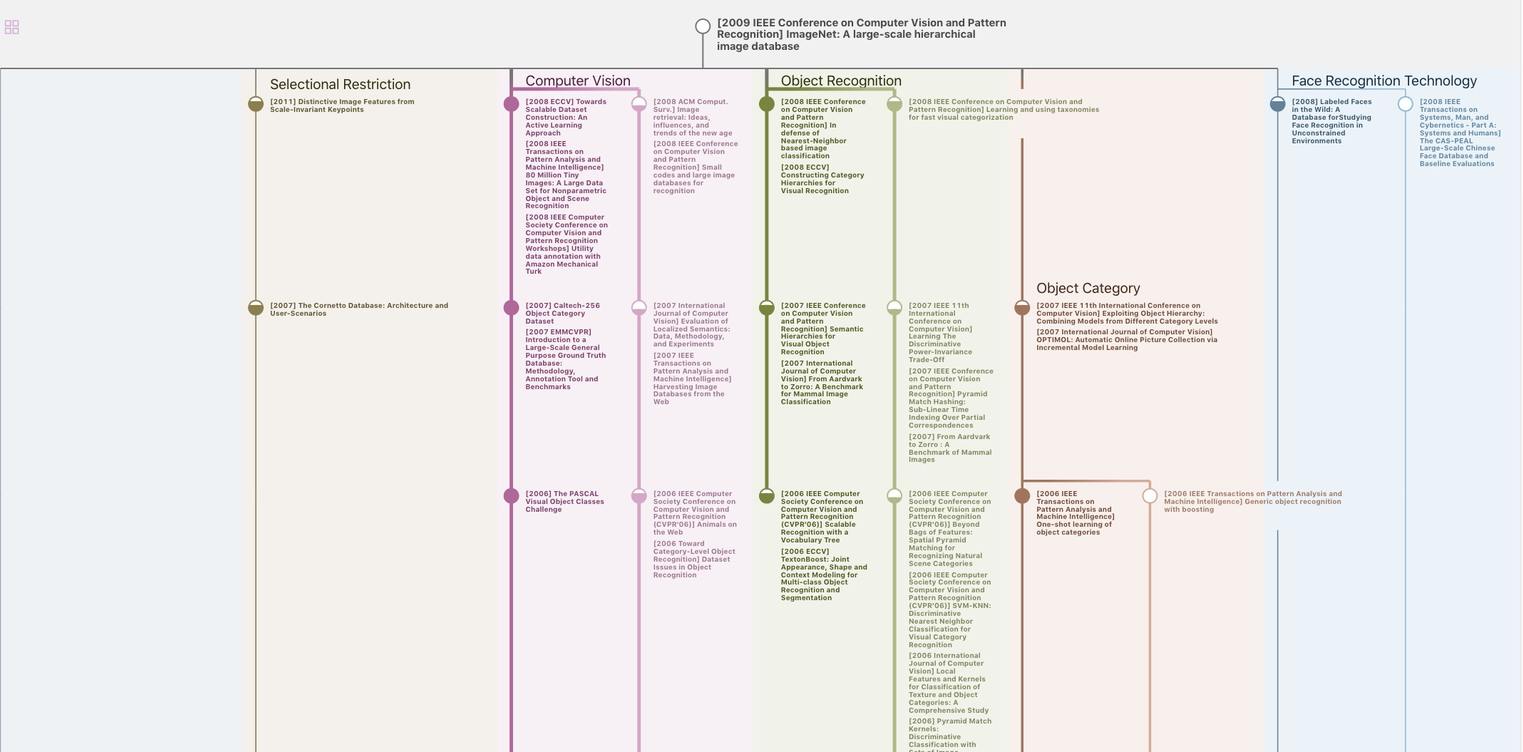
生成溯源树,研究论文发展脉络
Chat Paper
正在生成论文摘要