iNAV-drlSLAM: An Improved Indoor Self-Driving Framework for Mobile Robots using Deep Reinforcement Learning integrated with SLAM
2023 15th International Conference on Advanced Computational Intelligence (ICACI)(2023)
摘要
We introduce a new navigation framework for mobile robots that combines Simultaneous Localization and Mapping (SLAM) and deep reinforcement learning (DRL) techniques. While SLAM algorithms are effective at mapping and navigating through the environment, they struggle to avoid dynamic obstacles and can be computationally intensive. In contrast, DRL algorithms can manage dynamic situations but may result in sub-optimal performance when used alone. Our framework addresses this issue by using SLAM to generate a static path plan using the Ant Colony Optimization (ACO) algorithm. In dynamic environments, the robot follows the global path plan generated by SLAM and moves between way-points using a DRL-based local path planning algorithm. We evaluate the performance of three DRL-based navigation algorithms - Deep Q-Learning Network (DQN), Twin Delayed Deep Deterministic policy gradient (TD3), and Proximal Policy Optimization (PPO) - and compare them with existing navigation methods for mobile robots. Our approach overcomes the traditional reliance on static global and local maps, making it well-suited for dynamic environments. The proposed framework provides a promising alternative to current navigation methods for mobile robots, improving accuracy and efficiency while maintaining scalability and safety.
更多查看译文
关键词
Indoor Self-navigation,Simultaneous Localization and Mapping,Deep Reinforcement Learning,Autonomy,Mobile Robot
AI 理解论文
溯源树
样例
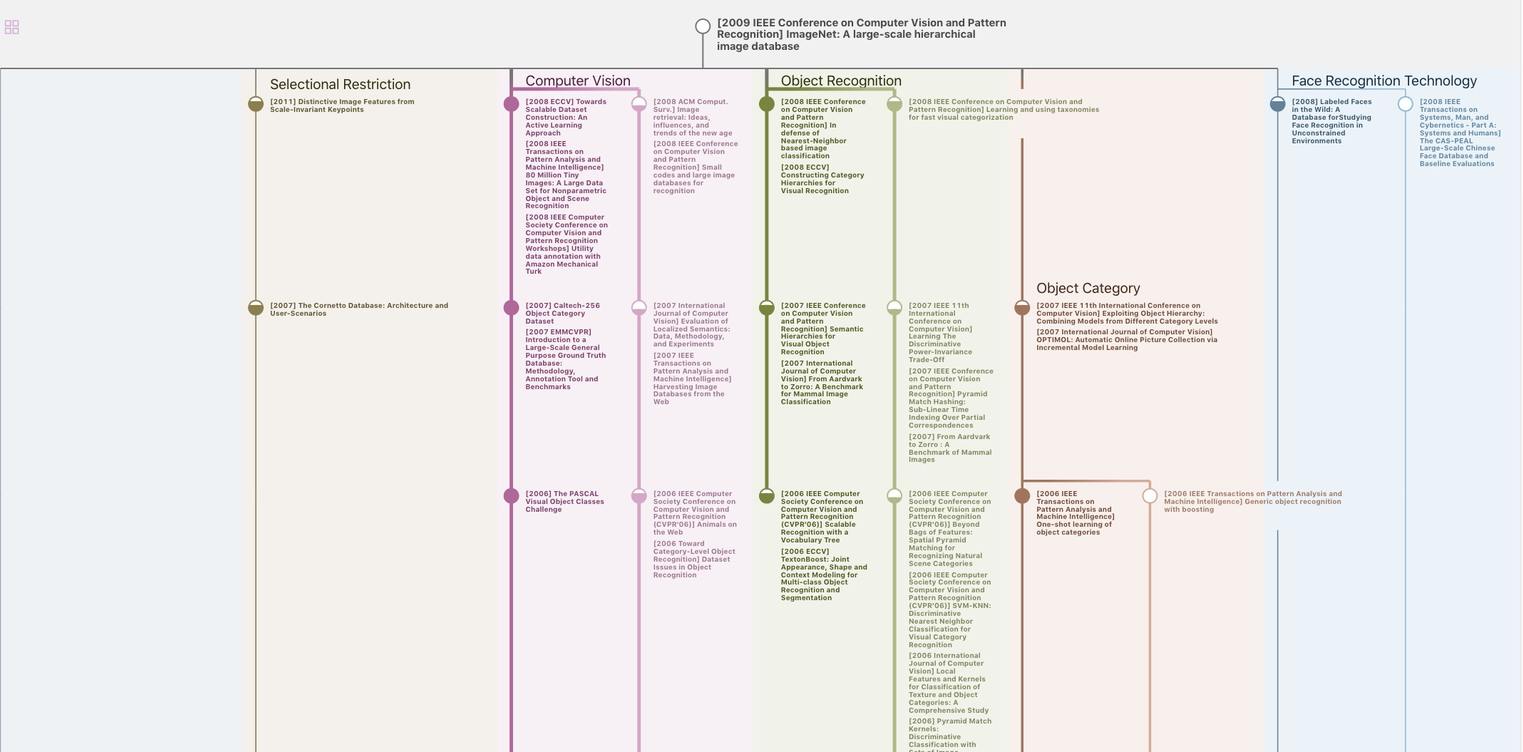
生成溯源树,研究论文发展脉络
Chat Paper
正在生成论文摘要