Generic Attacks against Cryptographic Hardware through Long-Range Deep Learning
CoRR(2023)
摘要
Hardware-based cryptographic implementations utilize countermeasures to resist side-channel attacks. In this paper, we propose a novel deep-learning architecture for side-channel analysis called SCANET that generalizes across multiple implementations and algorithms without manual tuning or trace pre-processing. We achieve this by combining a novel input processing technique with several advanced deep learning techniques including transformer blocks and multi-task learning. We demonstrate the generality of our approach by successfully attacking four hardware-accelerated countermeasures for elliptic curve digital signatures in an end-to-end manner without human tuning. Additionally, we showcase SCANET's ability to generalize across multiple algorithms by successfully replicating state-of-the-art attacks against protected AES without the need for trace preprocessing, hand-tuning, or model architectural changes. These results offer promising prospects for generic and automated side-channel leakage evaluation without manual effort.
更多查看译文
关键词
cryptographic hardware,deep
AI 理解论文
溯源树
样例
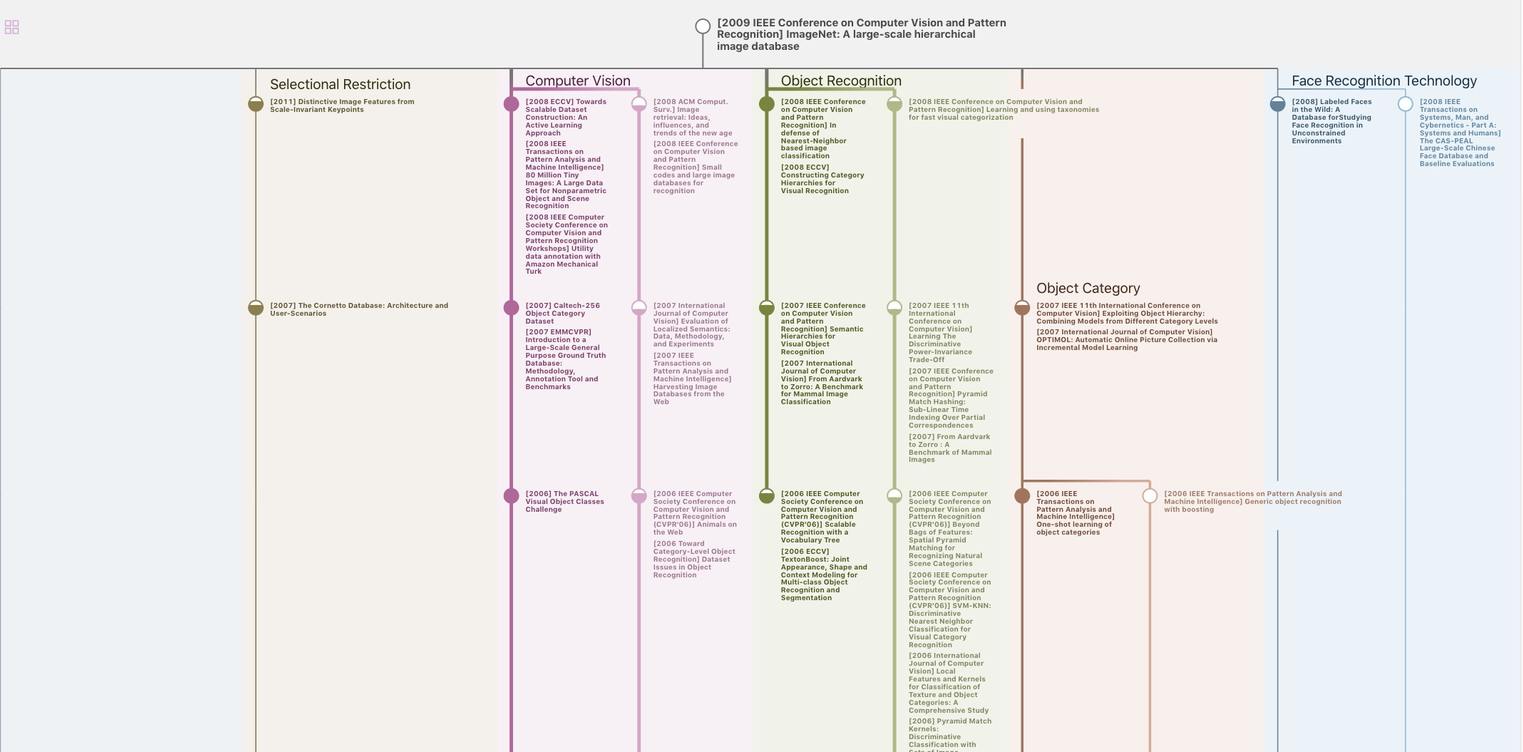
生成溯源树,研究论文发展脉络
Chat Paper
正在生成论文摘要