CD-CTFM: A Lightweight CNN-Transformer Network for Remote Sensing Cloud Detection Fusing Multiscale Features
IEEE JOURNAL OF SELECTED TOPICS IN APPLIED EARTH OBSERVATIONS AND REMOTE SENSING(2024)
摘要
Clouds in remote sensing images inevitably affect information extraction, which hinders the following analysis of satellite images. Hence, cloud detection is a necessary preprocessing procedure. However, most existing methods have numerous calculations and parameters. In this article, a lightweight convolutional neural network (CNN)-Transformer network, CD-CTFM, is proposed to solve the problem, which is based on encoder-decoder architecture and incorporates the attention mechanism. In the encoder part, we utilize a lightweight network combing CNN and Transformer as backbone, which is conducive to extracting local and global features simultaneously. The backbone of CD-CTFM also incorporates attention gate based on dark channel extraction module. Moreover, a lightweight feature pyramid module is designed to fuse multiscale features with contextual information. In the decoder part, a lightweight channel-spatial attention module is integrated into each skip connection between encoder and decoder to extract low-level features while suppressing irrelevant information without introducing many parameters. Finally, the proposed model is evaluated on two cloud datasets, 38-Cloud and MODIS. The results demonstrate that CD-CTFM achieves comparable accuracy as the state-of-art methods and outperforms in terms of efficiency.
更多查看译文
关键词
Feature extraction,Clouds,Transformers,Convolution,Remote sensing,Convolutional neural networks,Computer architecture,Attention mechanism,cloud detection,deep learning,lightweight network,vision transformer (ViT)
AI 理解论文
溯源树
样例
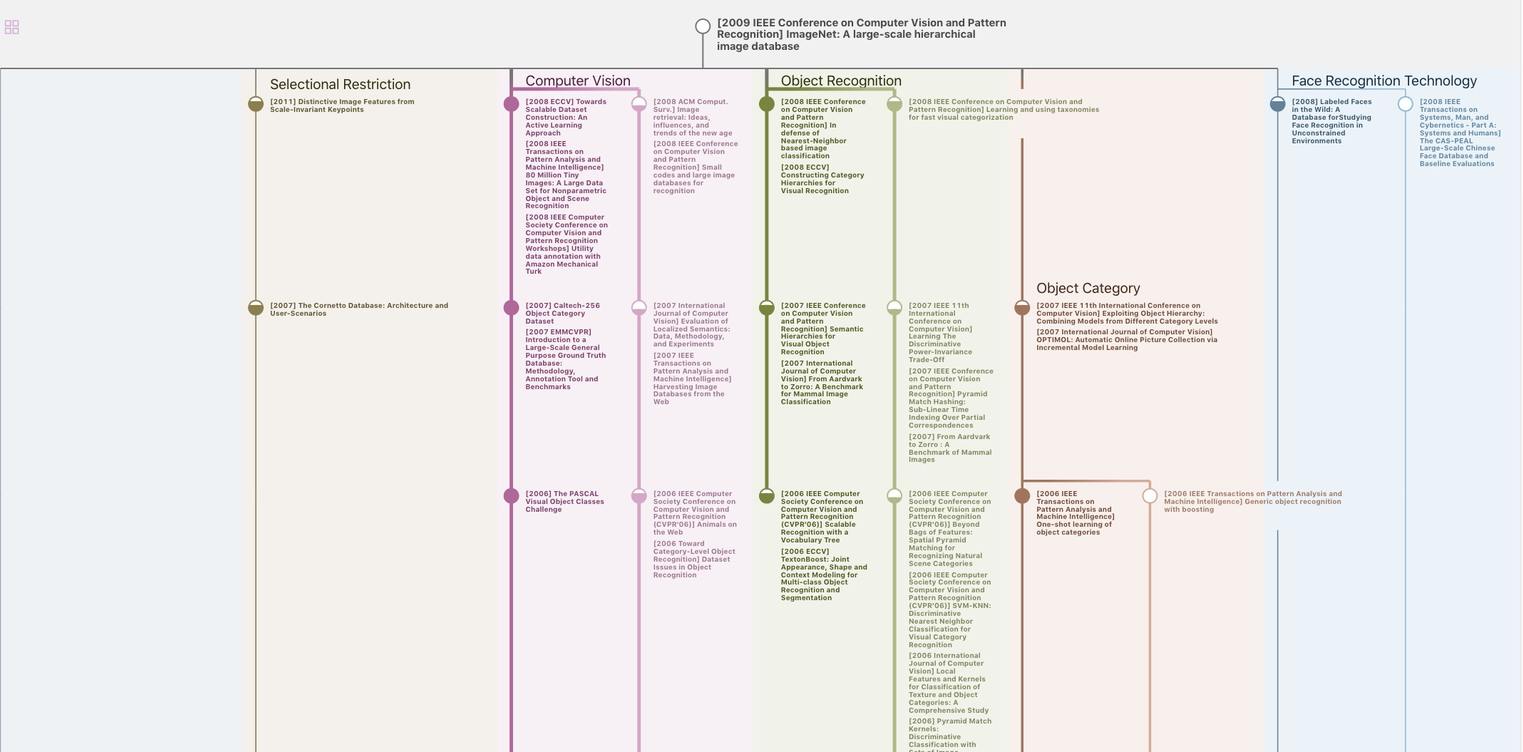
生成溯源树,研究论文发展脉络
Chat Paper
正在生成论文摘要