Imbalanced Multi-label Classification for Business-related Text with Moderately Large Label Spaces
CoRR(2023)
摘要
In this study, we compared the performance of four different methods for multi label text classification using a specific imbalanced business dataset. The four methods we evaluated were fine tuned BERT, Binary Relevance, Classifier Chains, and Label Powerset. The results show that fine tuned BERT outperforms the other three methods by a significant margin, achieving high values of accuracy, F1 Score, Precision, and Recall. Binary Relevance also performs well on this dataset, while Classifier Chains and Label Powerset demonstrate relatively poor performance. These findings highlight the effectiveness of fine tuned BERT for multi label text classification tasks, and suggest that it may be a useful tool for businesses seeking to analyze complex and multifaceted texts.
更多查看译文
关键词
classification,text,multi-label,business-related
AI 理解论文
溯源树
样例
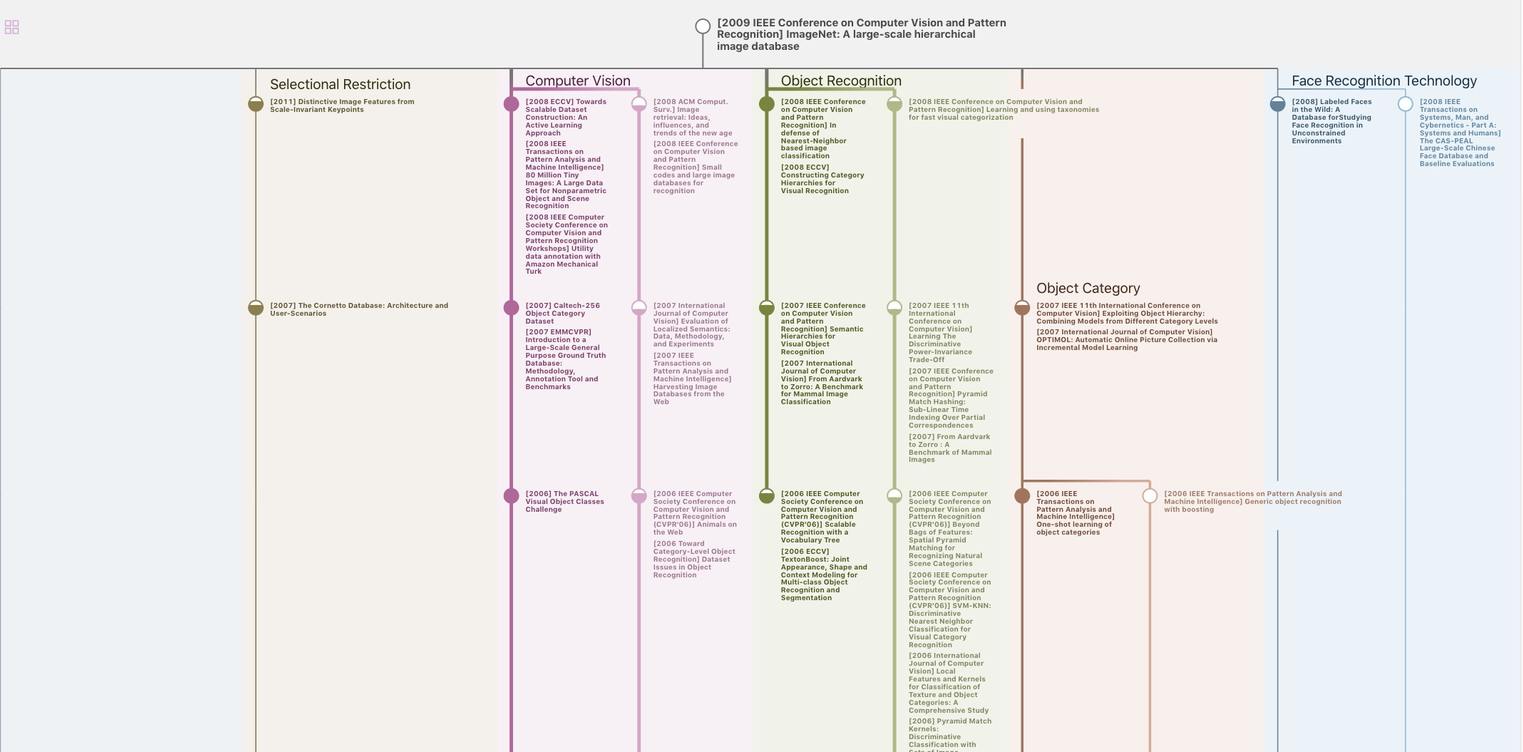
生成溯源树,研究论文发展脉络
Chat Paper
正在生成论文摘要