Localised Adaptive Spatial-Temporal Graph Neural Network
PROCEEDINGS OF THE 29TH ACM SIGKDD CONFERENCE ON KNOWLEDGE DISCOVERY AND DATA MINING, KDD 2023(2023)
摘要
Spatial-temporal graph models are prevailing for abstracting and modelling spatial and temporal dependencies. In this work, we ask the following question: \textit{whether and to what extent can we localise spatial-temporal graph models?} We limit our scope to adaptive spatial-temporal graph neural networks (ASTGNNs), the state-of-the-art model architecture. Our approach to localisation involves sparsifying the spatial graph adjacency matrices. To this end, we propose Adaptive Graph Sparsification (AGS), a graph sparsification algorithm which successfully enables the localisation of ASTGNNs to an extreme extent (fully localisation). We apply AGS to two distinct ASTGNN architectures and nine spatial-temporal datasets. Intriguingly, we observe that spatial graphs in ASTGNNs can be sparsified by over 99.5\% without any decline in test accuracy. Furthermore, even when ASTGNNs are fully localised, becoming graph-less and purely temporal, we record no drop in accuracy for the majority of tested datasets, with only minor accuracy deterioration observed in the remaining datasets. However, when the partially or fully localised ASTGNNs are reinitialised and retrained on the same data, there is a considerable and consistent drop in accuracy. Based on these observations, we reckon that \textit{(i)} in the tested data, the information provided by the spatial dependencies is primarily included in the information provided by the temporal dependencies and, thus, can be essentially ignored for inference; and \textit{(ii)} although the spatial dependencies provide redundant information, it is vital for the effective training of ASTGNNs and thus cannot be ignored during training. Furthermore, the localisation of ASTGNNs holds the potential to reduce the heavy computation overhead required on large-scale spatial-temporal data and further enable the distributed deployment of ASTGNNs.
更多查看译文
关键词
graph sparsification,spatial-temporal graph neural network,spatial-temporal data
AI 理解论文
溯源树
样例
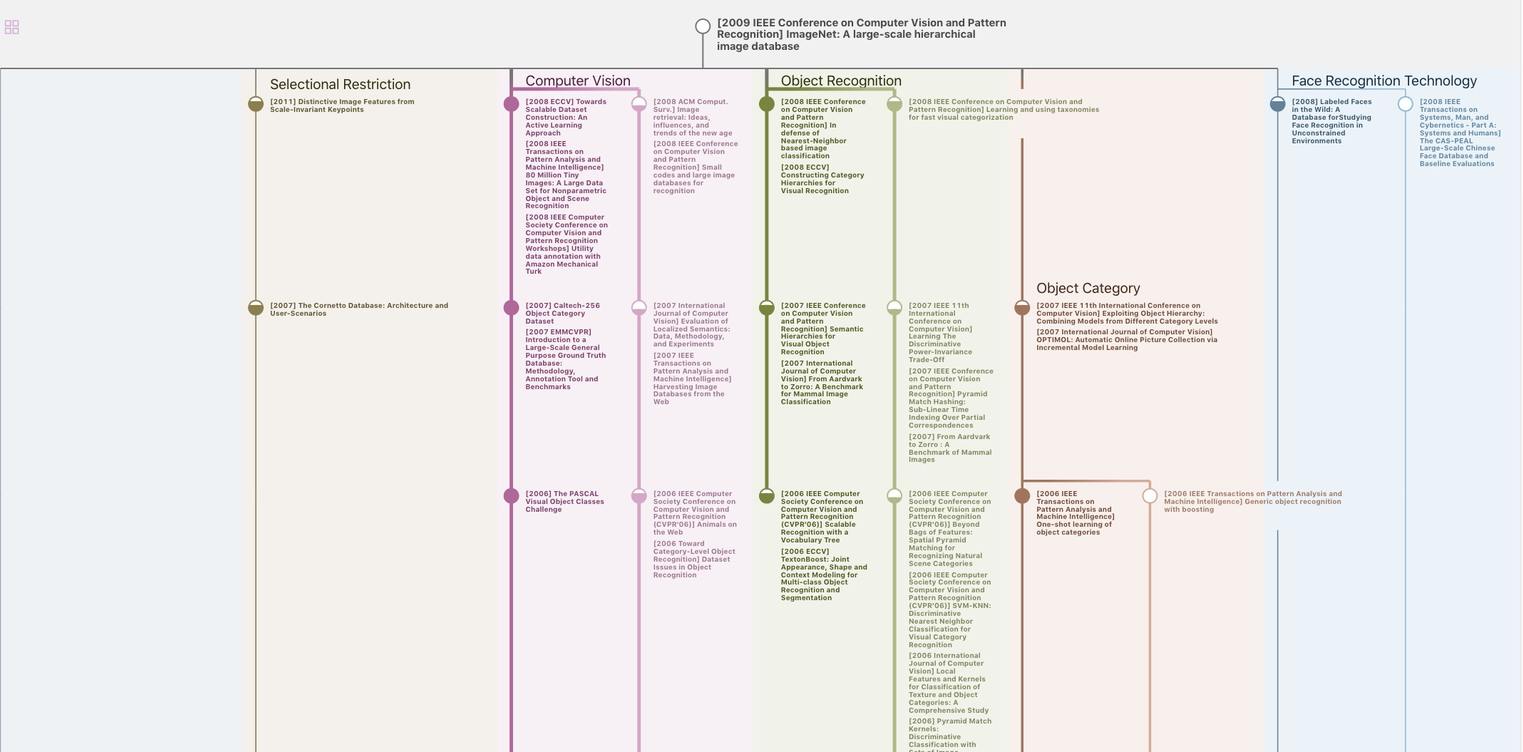
生成溯源树,研究论文发展脉络
Chat Paper
正在生成论文摘要