History Semantic Graph Enhanced Conversational KBQA with Temporal Information Modeling
conf_acl(2023)
摘要
Context information modeling is an important task in conversational KBQA. However, existing methods usually assume the independence of utterances and model them in isolation. In this paper, we propose a History Semantic Graph Enhanced KBQA model (HSGE) that is able to effectively model long-range semantic dependencies in conversation history while maintaining low computational cost. The framework incorporates a context-aware encoder, which employs a dynamic memory decay mechanism and models context at different levels of granularity. We evaluate HSGE on a widely used benchmark dataset for complex sequential question answering. Experimental results demonstrate that it outperforms existing baselines averaged on all question types.
更多查看译文
关键词
temporal,history,information
AI 理解论文
溯源树
样例
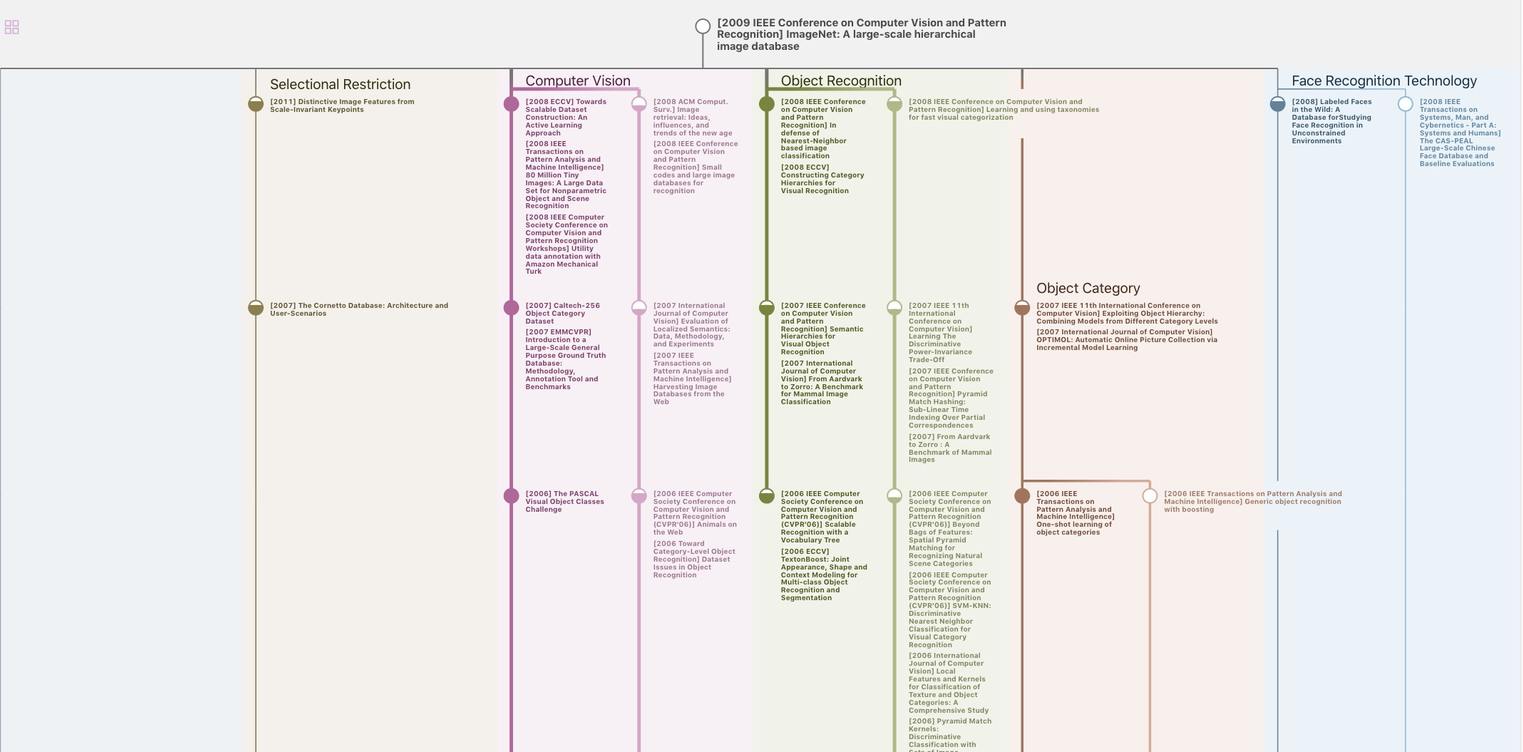
生成溯源树,研究论文发展脉络
Chat Paper
正在生成论文摘要