$E(2)$-Equivariant Vision Transformer
CoRR(2023)
摘要
Vision Transformer (ViT) has achieved remarkable performance in computer vision. However, positional encoding in ViT makes it substantially difficult to learn the intrinsic equivariance in data. Initial attempts have been made on designing equivariant ViT but are proved defective in some cases in this paper. To address this issue, we design a Group Equivariant Vision Transformer (GE-ViT) via a novel, effective positional encoding operator. We prove that GE-ViT meets all the theoretical requirements of an equivariant neural network. Comprehensive experiments are conducted on standard benchmark datasets, demonstrating that GE-ViT significantly outperforms non-equivariant self-attention networks. The code is available at https://github.com/ZJUCDSYangKaifan/GEVit.
更多查看译文
关键词
vision
AI 理解论文
溯源树
样例
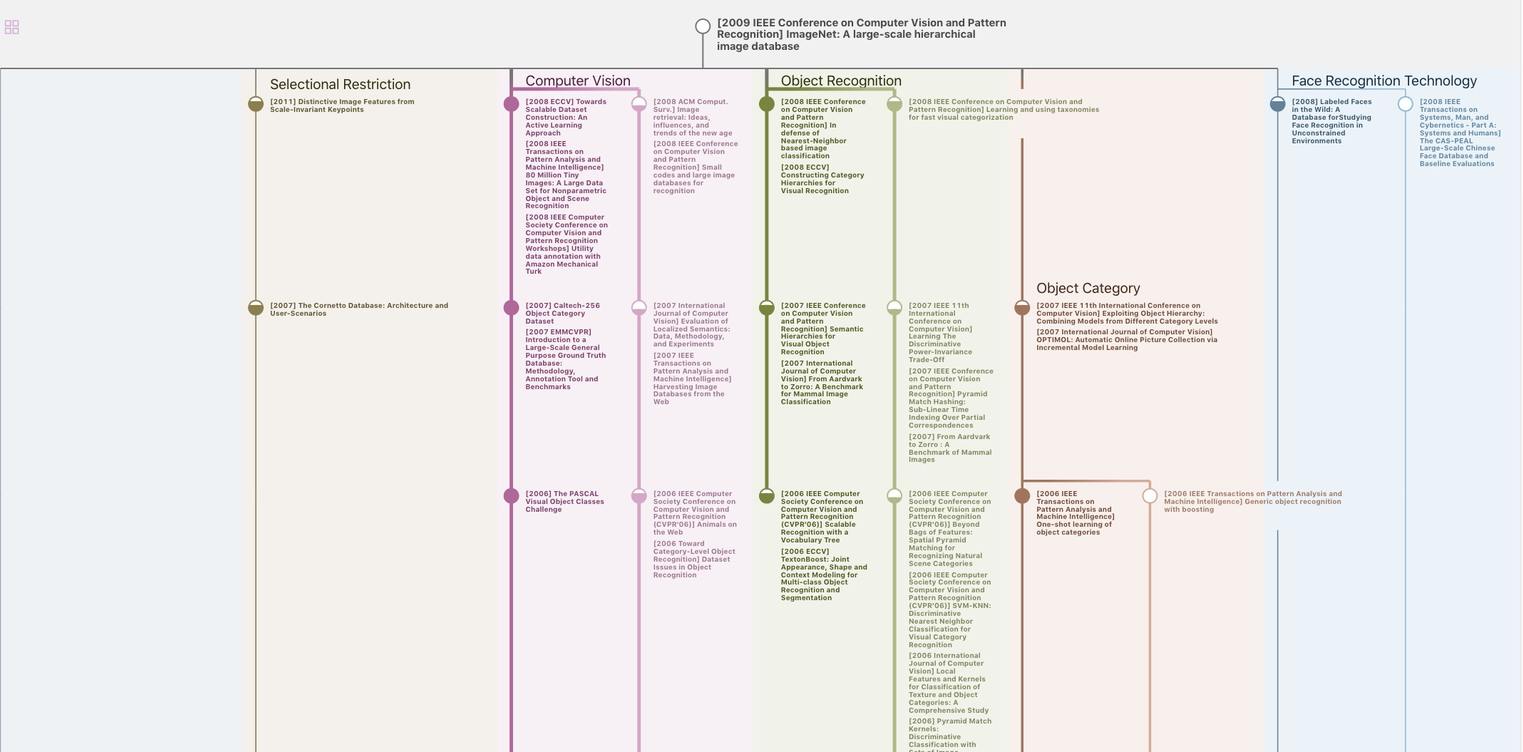
生成溯源树,研究论文发展脉络
Chat Paper
正在生成论文摘要