Distribution-free inference with hierarchical data
arXiv (Cornell University)(2023)
摘要
This paper studies distribution-free inference in settings where the data set has a hierarchical structure -- for example, groups of observations, or repeated measurements. In such settings, standard notions of exchangeability may not hold. To address this challenge, a hierarchical form of exchangeability is derived, facilitating extensions of distribution-free methods, including conformal prediction and jackknife+. While the standard theoretical guarantee obtained by the conformal prediction framework is a marginal predictive coverage guarantee, in the special case of independent repeated measurements, it is possible to achieve a stronger form of coverage -- the "second-moment coverage" property -- to provide better control of conditional miscoverage rates, and distribution-free prediction sets that achieve this property are constructed. Simulations illustrate that this guarantee indeed leads to uniformly small conditional miscoverage rates. Empirically, this stronger guarantee comes at the cost of a larger width of the prediction set in scenarios where the fitted model is poorly calibrated, but this cost is very mild in cases where the fitted model is accurate.
更多查看译文
关键词
data,distribution-free
AI 理解论文
溯源树
样例
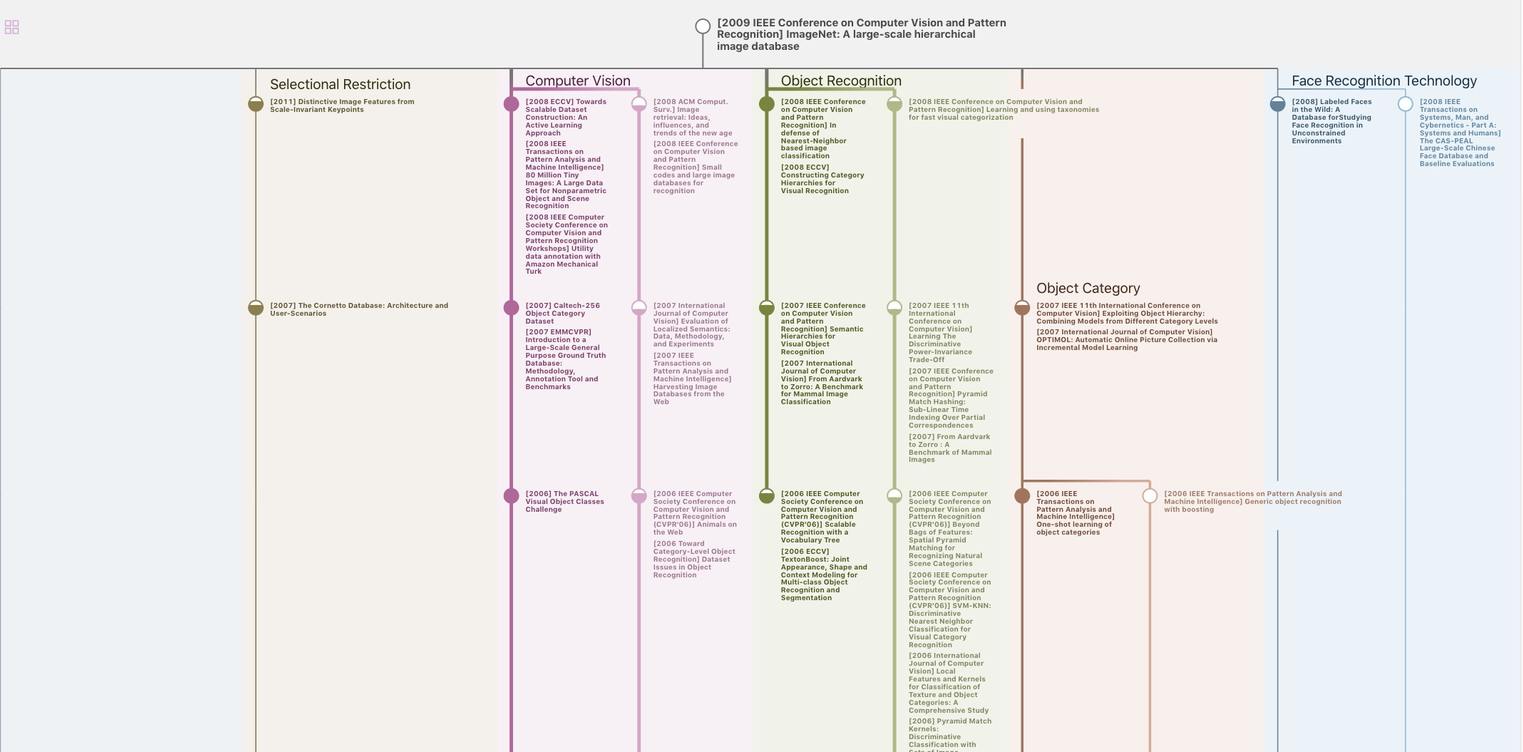
生成溯源树,研究论文发展脉络
Chat Paper
正在生成论文摘要