Hidden symmetries of ReLU networks
CoRR(2023)
摘要
The parameter space for any fixed architecture of feedforward ReLU neural networks serves as a proxy during training for the associated class of functions - but how faithful is this representation? It is known that many different parameter settings can determine the same function. Moreover, the degree of this redundancy is inhomogeneous: for some networks, the only symmetries are permutation of neurons in a layer and positive scaling of parameters at a neuron, while other networks admit additional hidden symmetries. In this work, we prove that, for any network architecture where no layer is narrower than the input, there exist parameter settings with no hidden symmetries. We also describe a number of mechanisms through which hidden symmetries can arise, and empirically approximate the functional dimension of different network architectures at initialization. These experiments indicate that the probability that a network has no hidden symmetries decreases towards 0 as depth increases, while increasing towards 1 as width and input dimension increase.
更多查看译文
关键词
relu networks,symmetries
AI 理解论文
溯源树
样例
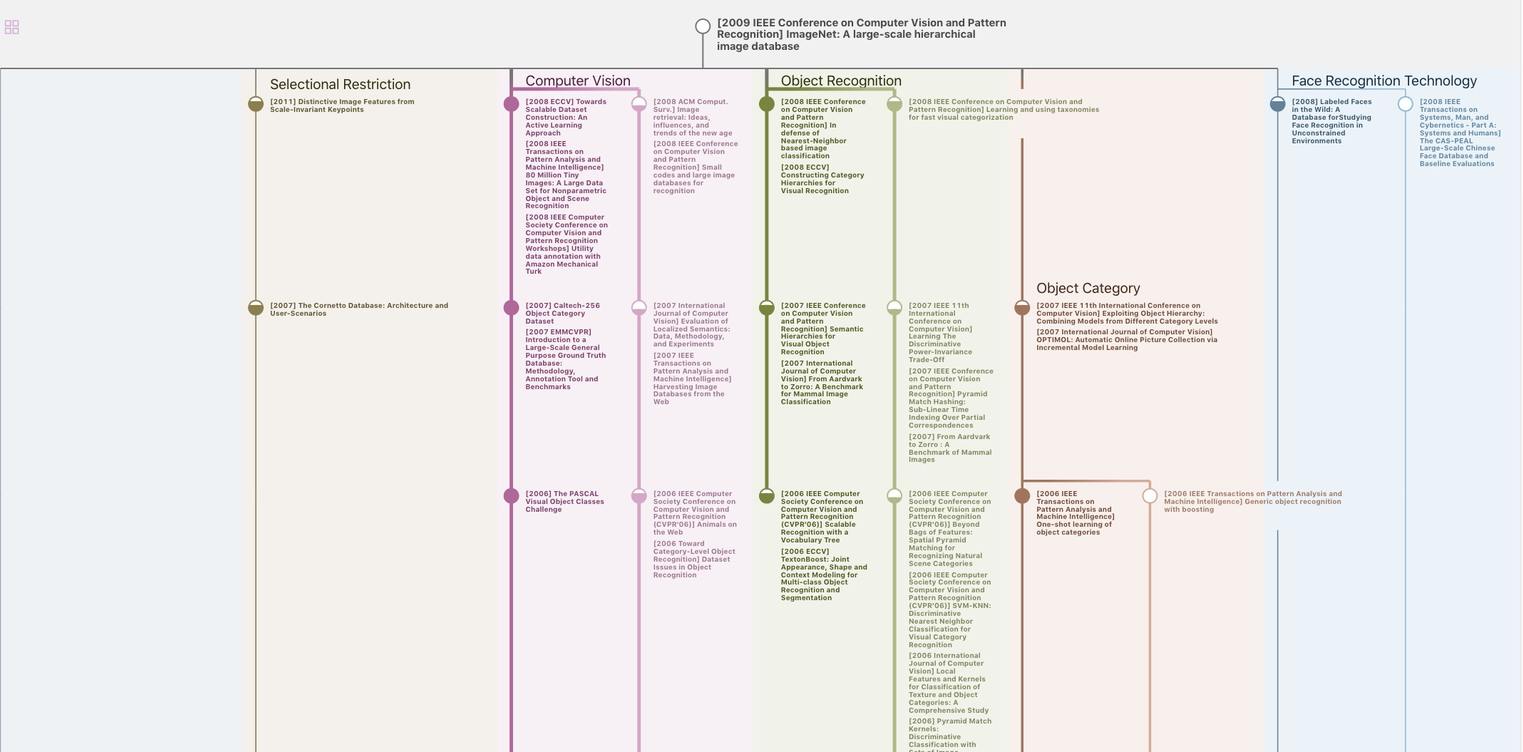
生成溯源树,研究论文发展脉络
Chat Paper
正在生成论文摘要