PCB Hardware Trojan Run-Time Detection Through Machine Learning
IEEE Transactions on Computers(2023)
摘要
The modern semiconductor electronic devices are becoming increasingly vulnerable to malicious implants called Hardware Trojans (HT). This problem is also greatly related to Printed Circuit Boards (PCB), which are widely used in almost all electronic devices. In this paper, two machine learning (ML) methods have been applied to detect HTs running on power from I/Os of legitimate chips on a PCB. A PCB prototype has been fabricated to obtain real-life data, which was used to train two ML algorithms: One-Class Support Vector Machine and Local Outlier Factor. For validation of the ML classifiers, one hundred categories of HT devices have been modelled and inserted into the Validation and Testing datasets. Simulation results show that using the proposed methodology an HT device can be detected with high prediction accuracy (F1-score above
$99.7\%$
for a
$50mW$
HT). Further, the ML model has been uploaded to the prototype PCB for hard-silicon validation of the methodology. To the best of our knowledge, this is the first work on real-time detection of PCB HTs, which are powered from the I/O pins of legitimate ICs. Experimental results show that the performance of the ML model on a real-life prototype is consistent with that of the simulations.
更多查看译文
关键词
Hardware trojan (HT), printed circuit board (PCB), machine learning on microcontroller, one class classification, one-class support vector machine, local outlier factor
AI 理解论文
溯源树
样例
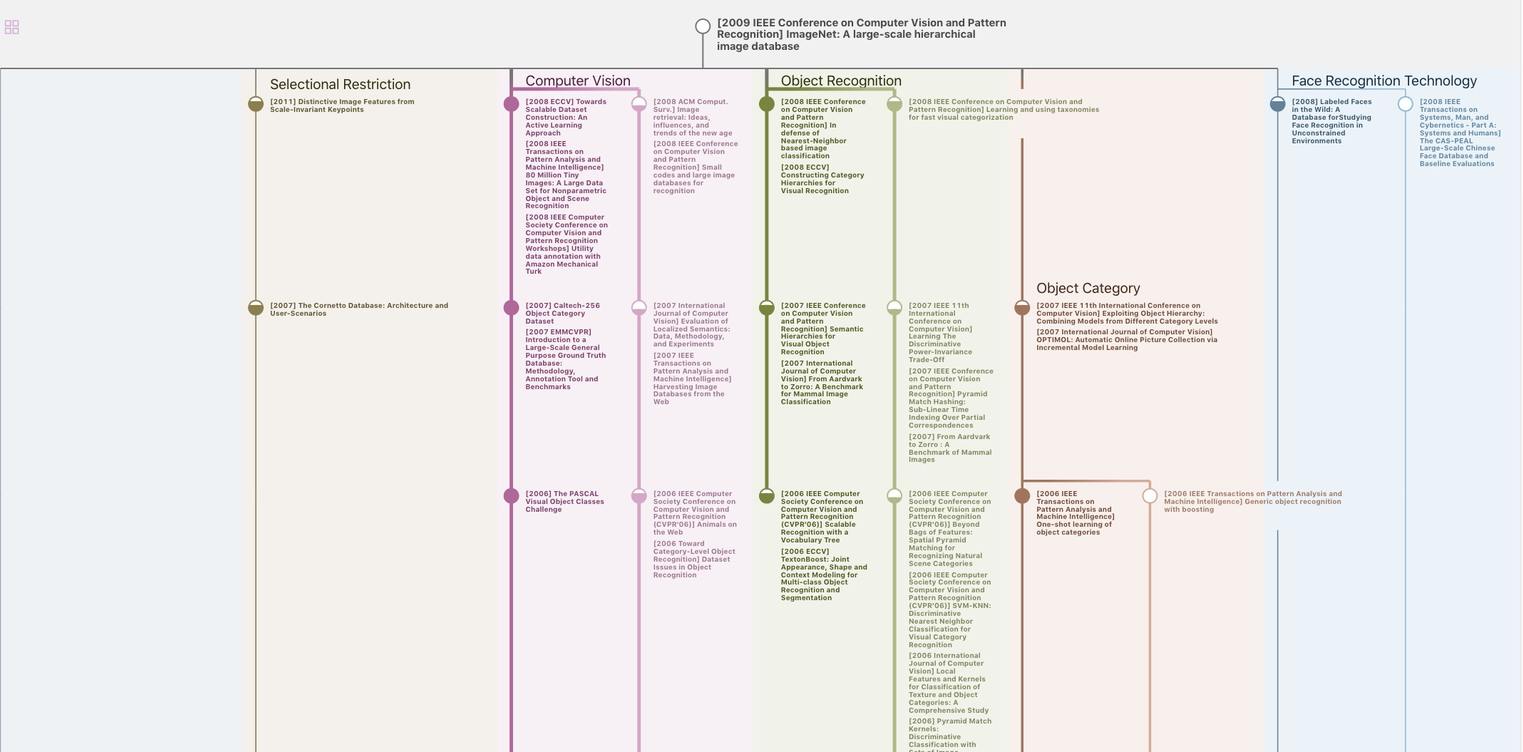
生成溯源树,研究论文发展脉络
Chat Paper
正在生成论文摘要