Channel Estimation Using CNN-LSTM in RIS-NOMA Assisted 6G Network
IEEE Transactions on Machine Learning in Communications and Networking(2023)
摘要
The combination of non-orthogonal multiple access (NOMA) and reconfigurable intelligent surface (RIS) technologies is proposed to meet the demands of data rate, latency, and connectivity in sixth-generation (6G) networks. The two techniques can support each other to increase the performance of the 6G system. In a RIS-aided system, channel estimation is a challenging problem, especially when applying passive RIS which has no signal processing. This paper proposes a deep learning (DL)-based channel estimation method using a convolutional long-short term memory (CNN-LSTM) model for RIS-NOMA wireless communication systems that integrate RIS and NOMA techniques. CNN-LSTM leverages both the benefits of convolutional neural network (CNN) as well as long-short term memory (LSTM), in which CNN can capture special features while LSTM can capture temporal features of time-series data. The simulation results indicate that the proposed CNN-LSTM model shows its robustness toward the variation of the RIS-NOMA system parameters, i.e., transmit signal-to-noise ratio (SNR), power allocation factor, and the number of RIS elements. The impacts of the RIS-NOMA system parameters on the prediction accuracy of the proposed DL-based channel estimation methods are evaluated via different performance metrics. The results reveal that the performance accuracy in terms of normalized root mean square error (NRMSE), coefficient of determination R-squared score (R2 score), mean absolute scaled error (MASE), and mean absolute percentage error (MAPE) increases with an increased transmit SNR, power allocation factor of the first user and the number of RIS elements. Additionally, the CNN-LSTM prediction performance shows its superiority as compared to those of the four benchmark models including the CNN1D-LSTM model using one-dimensional convolution layer (conv1D), CNN1D-BiLSTM model using bidirectional long-short term memory, CNN model and LSTM model.
更多查看译文
关键词
channel,network,cnn-lstm,ris-noma
AI 理解论文
溯源树
样例
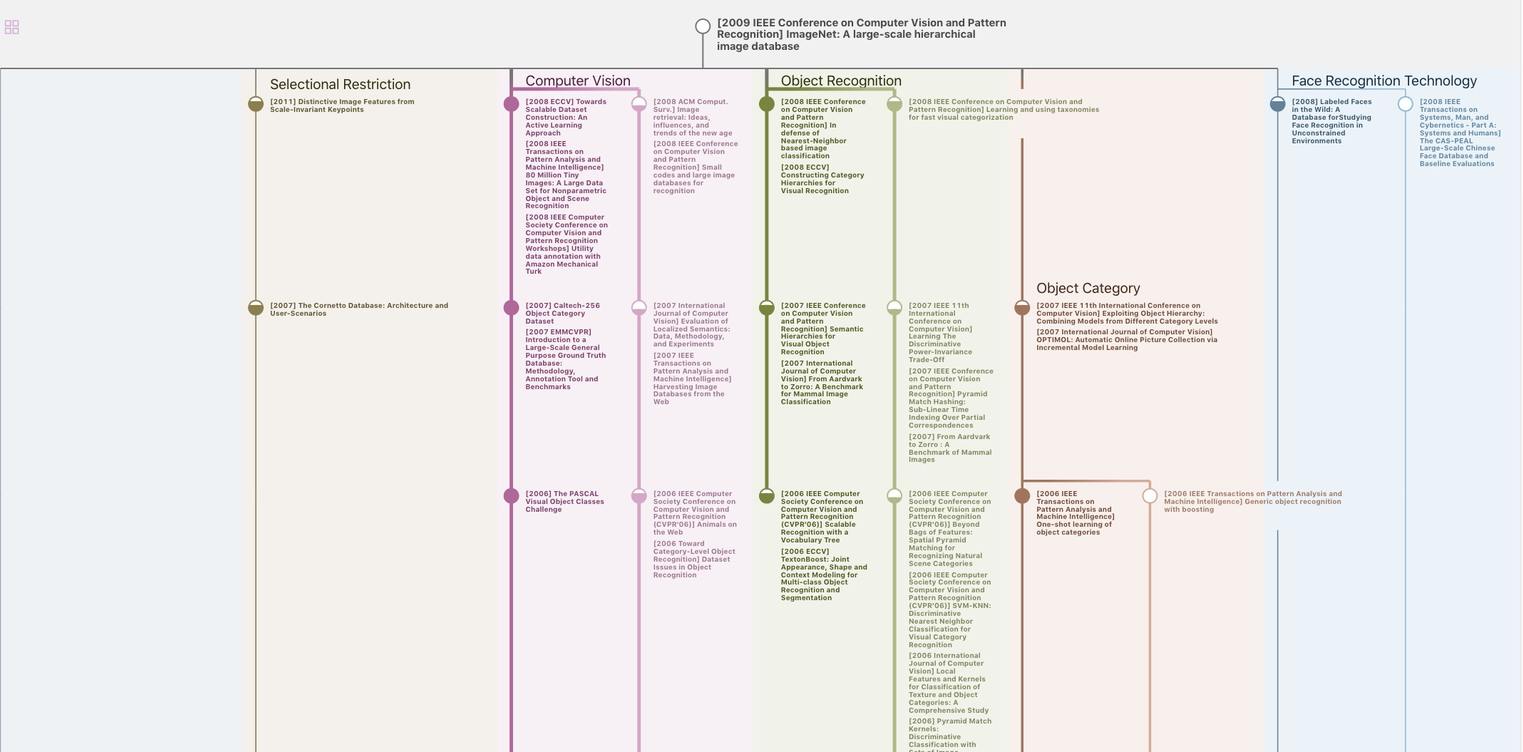
生成溯源树,研究论文发展脉络
Chat Paper
正在生成论文摘要