Data Synthesizing for Specific Human Action Segmentation
2023 IEEE International Conference on Industrial Technology (ICIT)(2023)
摘要
Temporal action segmentation has been one of the most challenging tasks in human activity analysis because it involves segmenting and recognizing actions in long action sequences, which requires a higher-level understanding of human activities. Many deep learning models have emerged and achieved excellent performance in action segmentation tasks. However, these models often require a large amount of labeled data for training. Generally, public datasets can help train models. But collecting and labeling sufficient training data can be costly for some specific actions that do not exist in public datasets. Therefore, we propose a framework for specific human action segmentation using data augmentation. Specifically, given a few labeled action sequences, we first segment them into short-trimmed action segments by label and then use a motion blending method to randomly combine these action segments representing different categories to form many new labeled action sequences. In this way, we can build a synthetic action dataset for the specific action, which provides enough data for training large-scale models. Our experimental results show that SOTA skeleton-based action segmentation models work well with our synthetic action dataset, and data augmentation can also improve the performance of these models.
更多查看译文
关键词
Skeleton-based action segmentation,Data augmentation,Motion blending
AI 理解论文
溯源树
样例
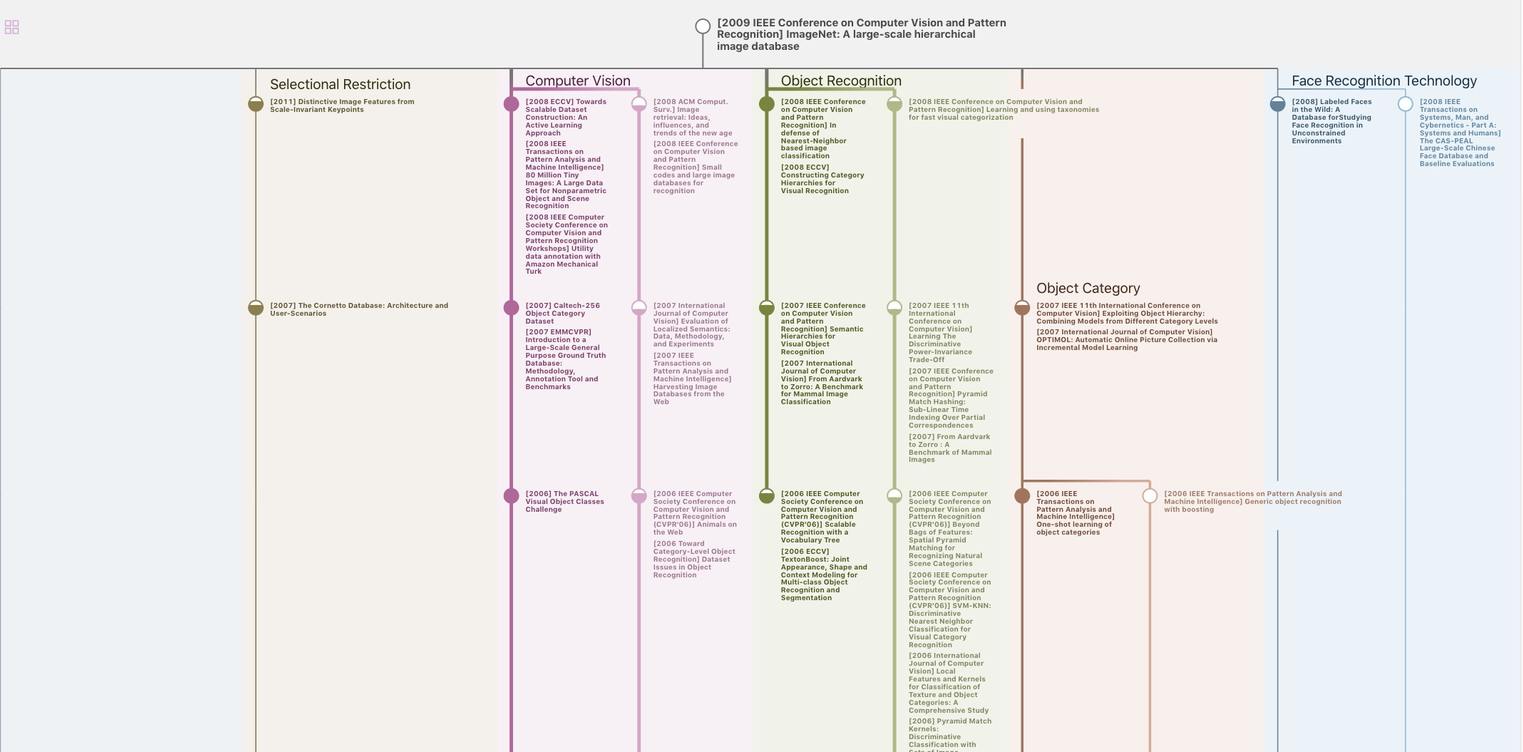
生成溯源树,研究论文发展脉络
Chat Paper
正在生成论文摘要