Comparison of Machine Learning Methods for Predicting Soil Total Nitrogen Content Using Landsat-8, Sentinel-1, and Sentinel-2 Images
Remote Sensing(2023)
摘要
Soil total nitrogen (STN) is a crucial component of the ecosystem's nitrogen pool, and accurate prediction of STN content is essential for understanding global nitrogen cycling processes. This study utilized the measured STN content of 126 sample points and 40 extracted remote sensing variables to predict the STN content and map its spatial distribution in the northeastern coastal region of Hebei Province, China, employing the random forest (RF), gradient boosting machine (GBM), and extreme gradient boosting (XGBoost) methods. The purpose was to compare the ability of remote sensing images (Landsat-8, Sentinel-1, and Sentinel-2) with different machine learning methods for predicting STN content. The research results show the following: (1) The three machine learning methods accurately predicted the STN content and the optimal model provided by the XGBoost method, with an R-2 of 0.627, RMSE of 0.127 g.kg(-1), and MAE of 0.092 g.kg(-1). (2) The combination of optical and synthetic aperture radar (SAR) images improved prediction accuracy, with the R-2 improving by 45.5%. (3) The importance of optical images is higher than that of SAR images in the RF, GBM, and XGBoost methods, with optical images accounting for 87%, 76%, and 77% importance, respectively. (4) The spatial distribution of STN content predicted by the three methods is similar. Higher STN contents are distributed in the northern part of the study area, while lower STN contents are distributed in coastal areas. The results of this study can be very useful for inventories of soil nitrogen and provide data support and method references for revealing nitrogen cycling.
更多查看译文
关键词
soil total nitrogen content,random forest,gradient boosting machine,extreme gradient boosting,remote sensing,digital soil mapping
AI 理解论文
溯源树
样例
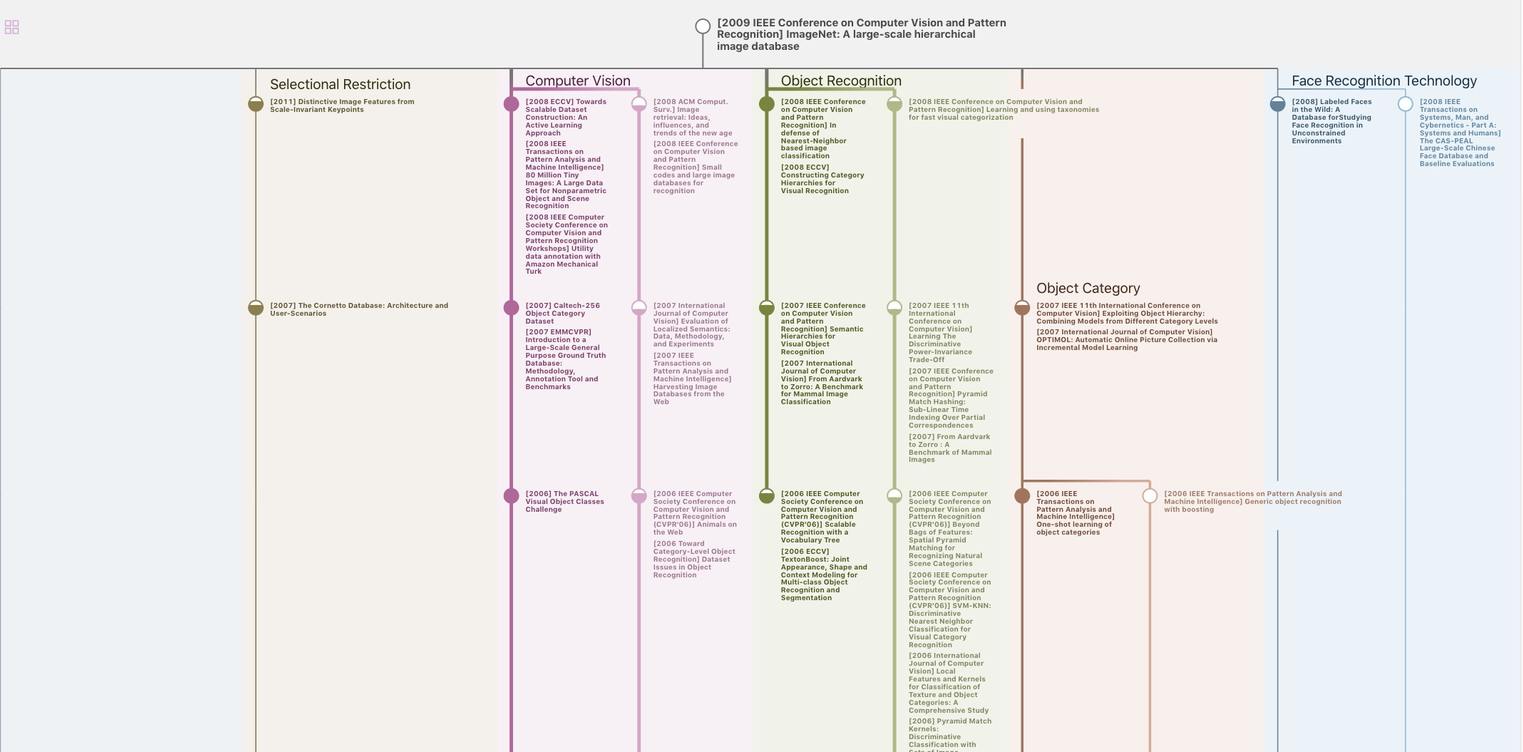
生成溯源树,研究论文发展脉络
Chat Paper
正在生成论文摘要