Dynamic Partial Computation Offloading for the Metaverse in In-Network Computing
IEEE ACCESS(2024)
摘要
The computing in the network (COIN) paradigm is a promising solution that leverages unused network resources to perform tasks to meet computation-demanding applications, such as the metaverse. In this vein, we consider the partial computation offloading problem in the metaverse for multiple subtasks in a COIN environment to minimize energy consumption and delay while dynamically adjusting the offloading policy based on the changing computational resource status. The problem is NP-hard, and we transform it into two subproblems: the task-splitting problem (TSP) on the user side and the task-offloading problem (TOP) on the COIN side. We model the TSP as an ordinal potential game and propose a decentralized algorithm to obtain its Nash equilibrium (NE). Then, we model the TOP as a Markov decision process and propose the double deep Q-network (DDQN) to solve for the optimal offloading policy. Unlike the conventional DDQN algorithm, where intelligent agents sample offloading decisions randomly within a certain probability, the COIN agent explores the NE of the TSP and the deep neural network. Finally, the simulation results reveal that the proposed model approach allows the COIN agent to update its policies and make more informed decisions, leading to improved performance over time compared to the traditional baseline.
更多查看译文
关键词
Task analysis,Metaverse,Resource management,X reality,Dynamic scheduling,Delays,Optimization,Computational offloading,deep reinforcement learning,game theory,in-network computing,metaverse
AI 理解论文
溯源树
样例
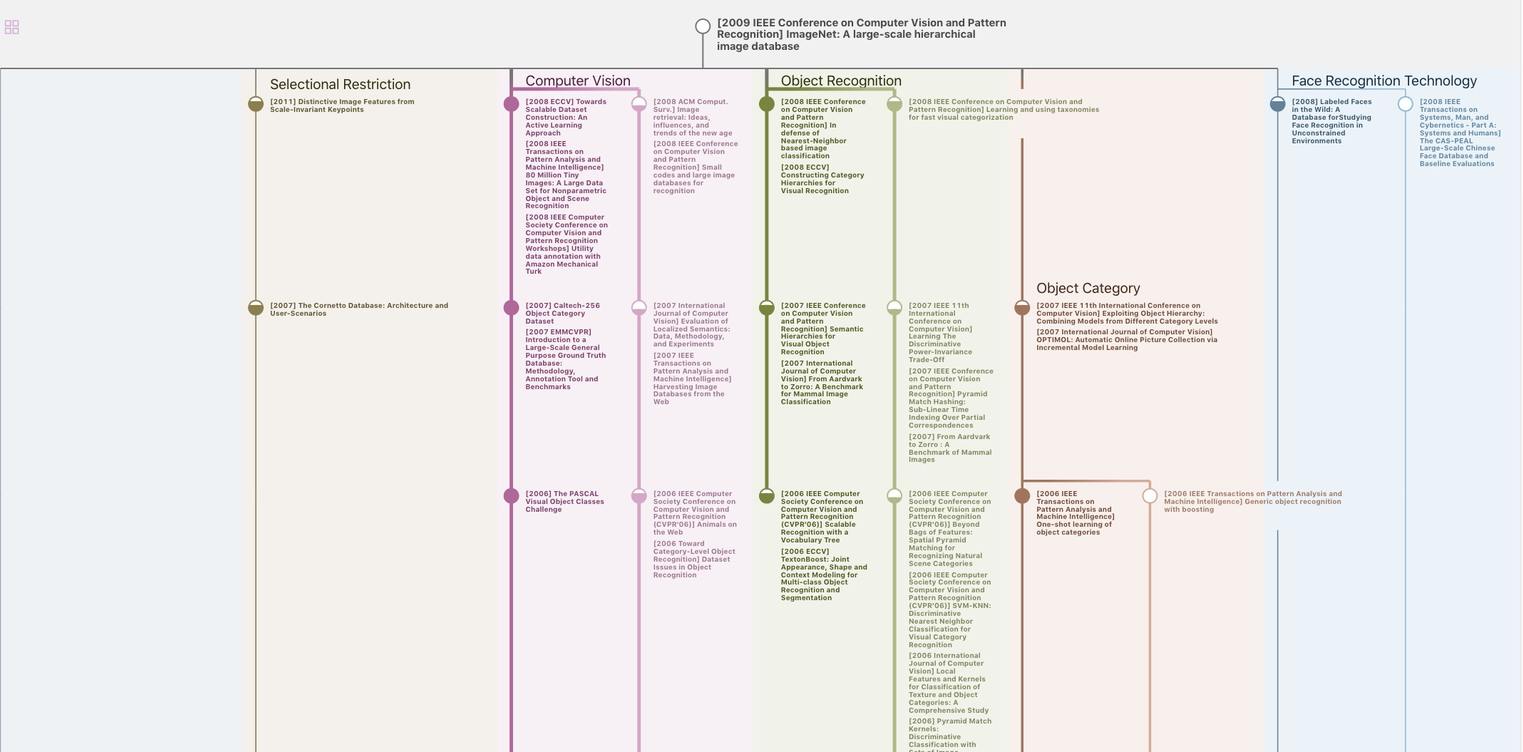
生成溯源树,研究论文发展脉络
Chat Paper
正在生成论文摘要