Robust Data-driven Prescriptiveness Optimization
CoRR(2023)
摘要
The abundance of data has led to the emergence of a variety of optimization techniques that attempt to leverage available side information to provide more anticipative decisions. The wide range of methods and contexts of application have motivated the design of a universal unitless measure of performance known as the coefficient of prescriptiveness. This coefficient was designed to quantify both the quality of contextual decisions compared to a reference one and the prescriptive power of side information. To identify policies that maximize the former in a data-driven context, this paper introduces a distributionally robust contextual optimization model where the coefficient of prescriptiveness substitutes for the classical empirical risk minimization objective. We present a bisection algorithm to solve this model, which relies on solving a series of linear programs when the distributional ambiguity set has an appropriate nested form and polyhedral structure. Studying a contextual shortest path problem, we evaluate the robustness of the resulting policies against alternative methods when the out-of-sample dataset is subject to varying amounts of distribution shift.
更多查看译文
关键词
optimization,data-driven
AI 理解论文
溯源树
样例
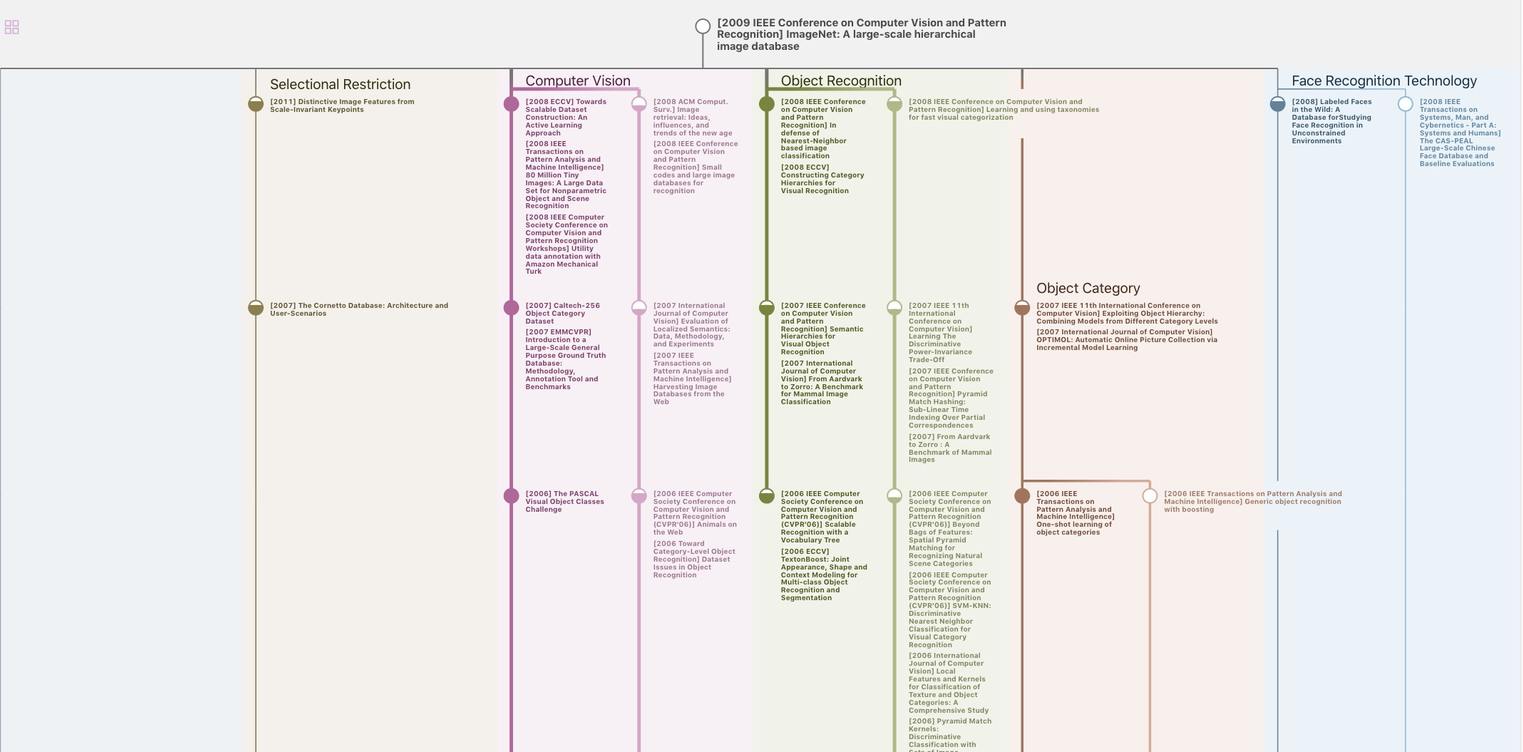
生成溯源树,研究论文发展脉络
Chat Paper
正在生成论文摘要