A statistical and machine learning approach to the study of astrochemistry
Faraday discussions(2023)
摘要
In order to obtain a good understanding of astrochemistry, it is crucial to better understand the key parameters that govern grain-surface chemistry. For many chemical networks, these crucial parameters are the binding energies of the species. However, there exists much disagreement regarding these values in the literature. In this work, a Bayesian inference approach is taken to estimate these values. It is found that this is difficult to do in the absence of enough data. The Massive Optimised Parameter Estimation and Data (MOPED) compression algorithm is then used to help determine which species should be prioritised for future detections in order to better constrain the values of binding energies. Finally, an interpretable machine learning approach is taken in order to better understand the non-linear relationship between binding energies and the final abundances of specific species of interest.
更多查看译文
关键词
machine learning,machine learning approach
AI 理解论文
溯源树
样例
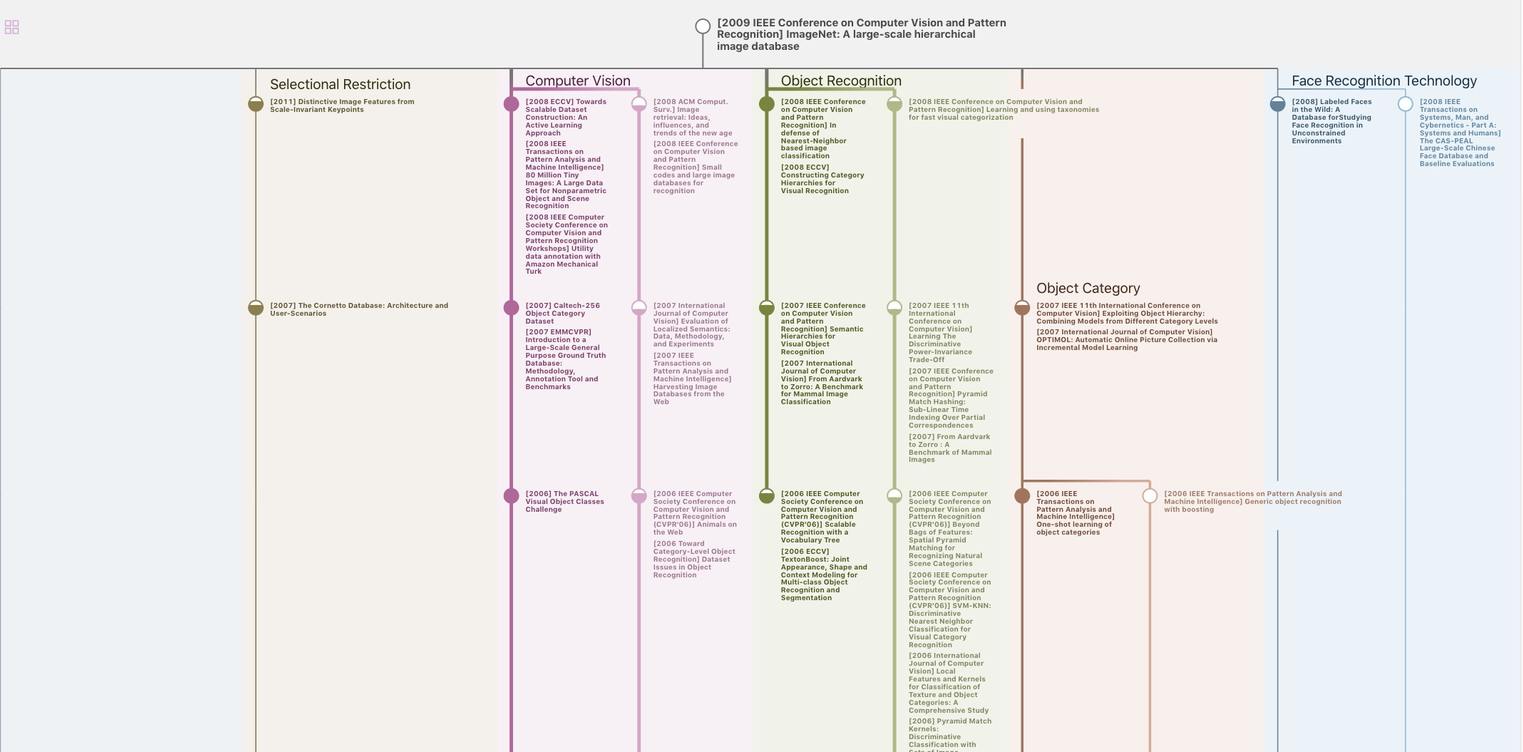
生成溯源树,研究论文发展脉络
Chat Paper
正在生成论文摘要