Redesigning spectroscopic sensors with programmable photonic circuits
arxiv(2023)
摘要
Optical spectroscopic sensors are a powerful tool to reveal light-matter interactions in many fields, such as physics, biology, chemistry, and astronomy. Miniaturizing the currently bulky spectrometers has become imperative for the wide range of applications that demand in situ or even in vitro characterization systems, a field that is growing rapidly. Benchtop spectrometers are capable of offering superior resolution and spectral range, but at the expense of requiring a large size. In this paper, we propose a novel method that redesigns spectroscopic sensors via the use of programmable photonic circuits. Drawing from compressive sensing theory, we start by investigating the most ideal sampling matrix for a reconstructive spectrometer and reveal that a sufficiently large number of sampling channels is a prerequisite for both fine resolution and low reconstruction error. This number is, however, still considerably smaller than that of the reconstructed spectral pixels, benefitting from the nature of reconstruction algorithms. We then show that the cascading of a few engineered MZI elements can be readily programmed to create an exponentially scalable number of such sampling spectral responses over an ultra-broad bandwidth, allowing for ultra-high resolution down to single-digit picometers without incurring additional hardware costs. Experimentally, we implement an on-chip spectrometer with a fully-programmable 6-stage cascaded MZI structure and demonstrate a < 10 pm resolution with a > 200 nm bandwidth using only 729 sampling channels. This achieves a bandwidth-to-resolution ratio of over 20,000, which is, to our best knowledge, about one order of magnitude greater than any reported miniaturized spectrometers to date. We further illustrate that by employing dispersion-engineered waveguide components, the device bandwidth can be extended to over 400 nm.
更多查看译文
关键词
spectroscopic sensors
AI 理解论文
溯源树
样例
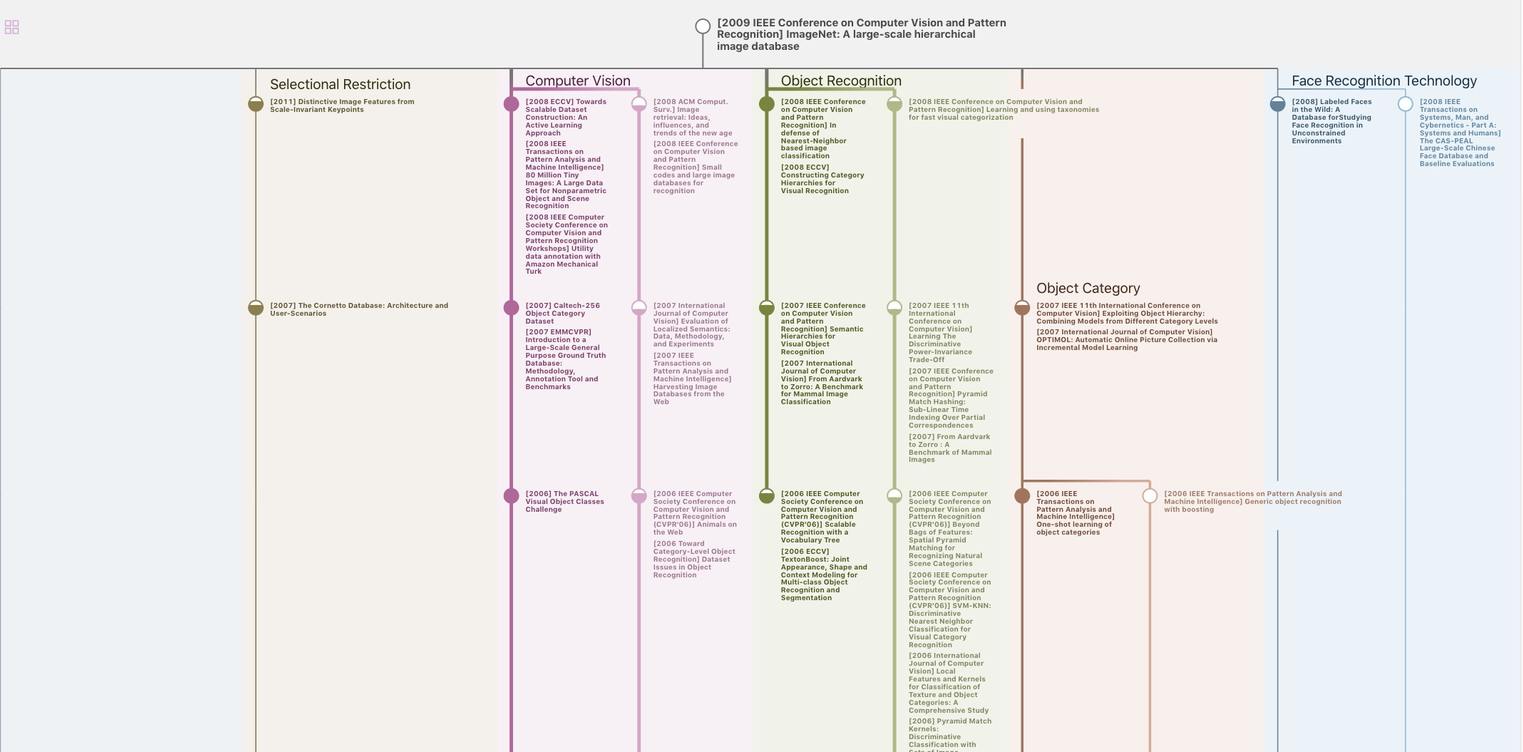
生成溯源树,研究论文发展脉络
Chat Paper
正在生成论文摘要