Judging LLM-as-a-judge with MT-Bench and Chatbot Arena
NeurIPS(2023)
摘要
Evaluating large language model (LLM) based chat assistants is challenging due to their broad capabilities and the inadequacy of existing benchmarks in measuring human preferences. To address this, we explore using strong LLMs as judges to evaluate these models on more open-ended questions. We examine the usage and limitations of LLM-as-a-judge, such as position and verbosity biases and limited reasoning ability, and propose solutions to migrate some of them. We then verify the agreement between LLM judges and human preferences by introducing two benchmarks: MT-bench, a multi-turn question set; and Chatbot Arena, a crowdsourced battle platform. Our results reveal that strong LLM judges like GPT-4 can match both controlled and crowdsourced human preferences well, achieving over 80\% agreement, the same level of agreement between humans. Hence, LLM-as-a-judge is a scalable and explainable way to approximate human preferences, which are otherwise very expensive to obtain. Additionally, we show our benchmark and traditional benchmarks complement each other by evaluating several variants of LLaMA/Vicuna. We will publicly release 80 MT-bench questions, 3K expert votes, and 30K conversations with human preferences from Chatbot Arena.
更多查看译文
关键词
chatbot arena,llm-as-a-judge,mt-bench
AI 理解论文
溯源树
样例
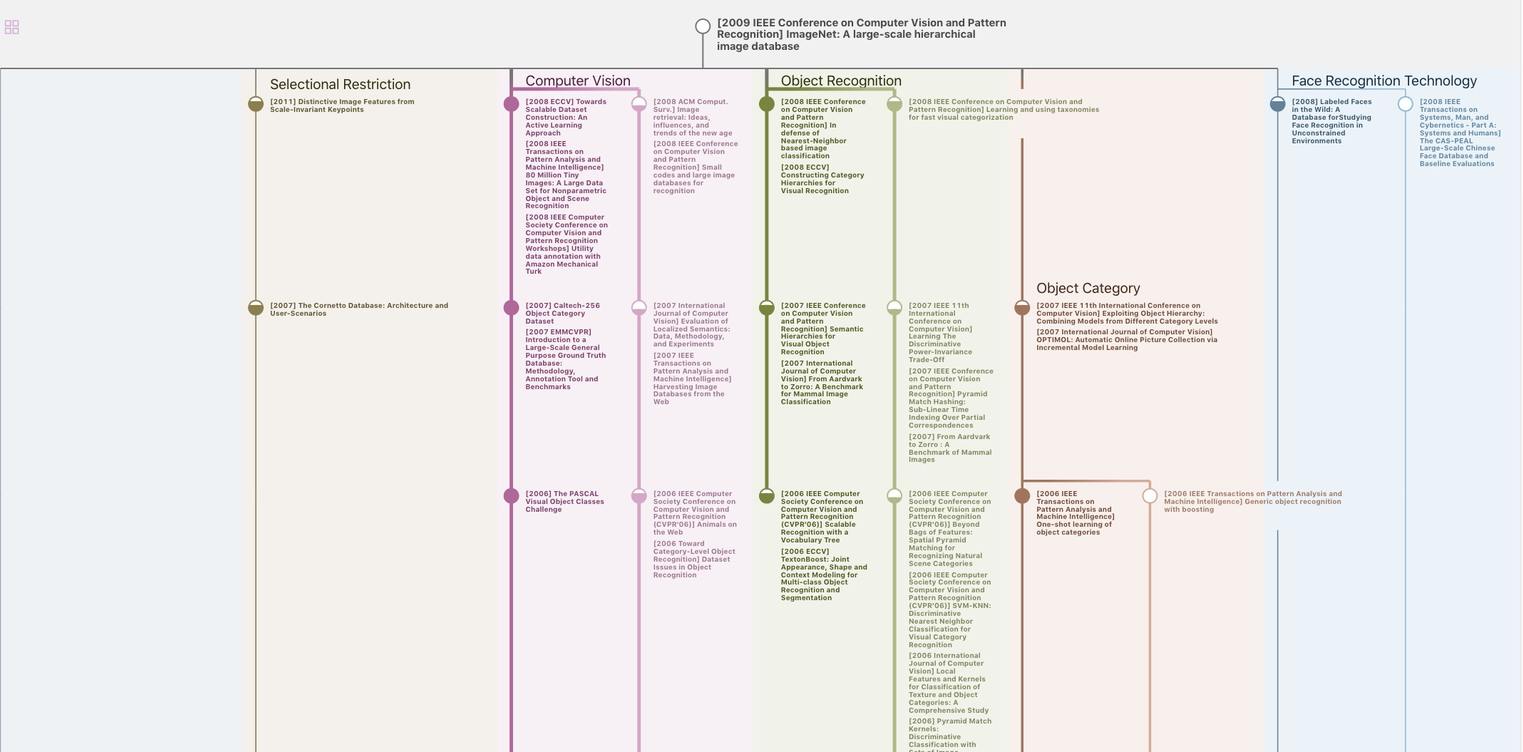
生成溯源树,研究论文发展脉络
Chat Paper
正在生成论文摘要