Learning Fine-grained View-Invariant Representations from Unpaired Ego-Exo Videos via Temporal Alignment
NeurIPS(2023)
摘要
The egocentric and exocentric viewpoints of a human activity look dramatically different, yet invariant representations to link them are essential for many potential applications in robotics and augmented reality. Prior work is limited to learning view-invariant features from paired synchronized viewpoints. We relax that strong data assumption and propose to learn fine-grained action features that are invariant to the viewpoints by aligning egocentric and exocentric videos in time, even when not captured simultaneously or in the same environment. To this end, we propose AE2, a self-supervised embedding approach with two key designs: (1) an object-centric encoder that explicitly focuses on regions corresponding to hands and active objects; (2) a contrastive-based alignment objective that leverages temporally reversed frames as negative samples. For evaluation, we establish a benchmark for fine-grained video understanding in the ego-exo context, comprising four datasets -- including an ego tennis forehand dataset we collected, along with dense per-frame labels we annotated for each dataset. On the four datasets, our AE2 method strongly outperforms prior work in a variety of fine-grained downstream tasks, both in regular and cross-view settings.
更多查看译文
关键词
representations,videos,fine-grained,view-invariant,ego-exo
AI 理解论文
溯源树
样例
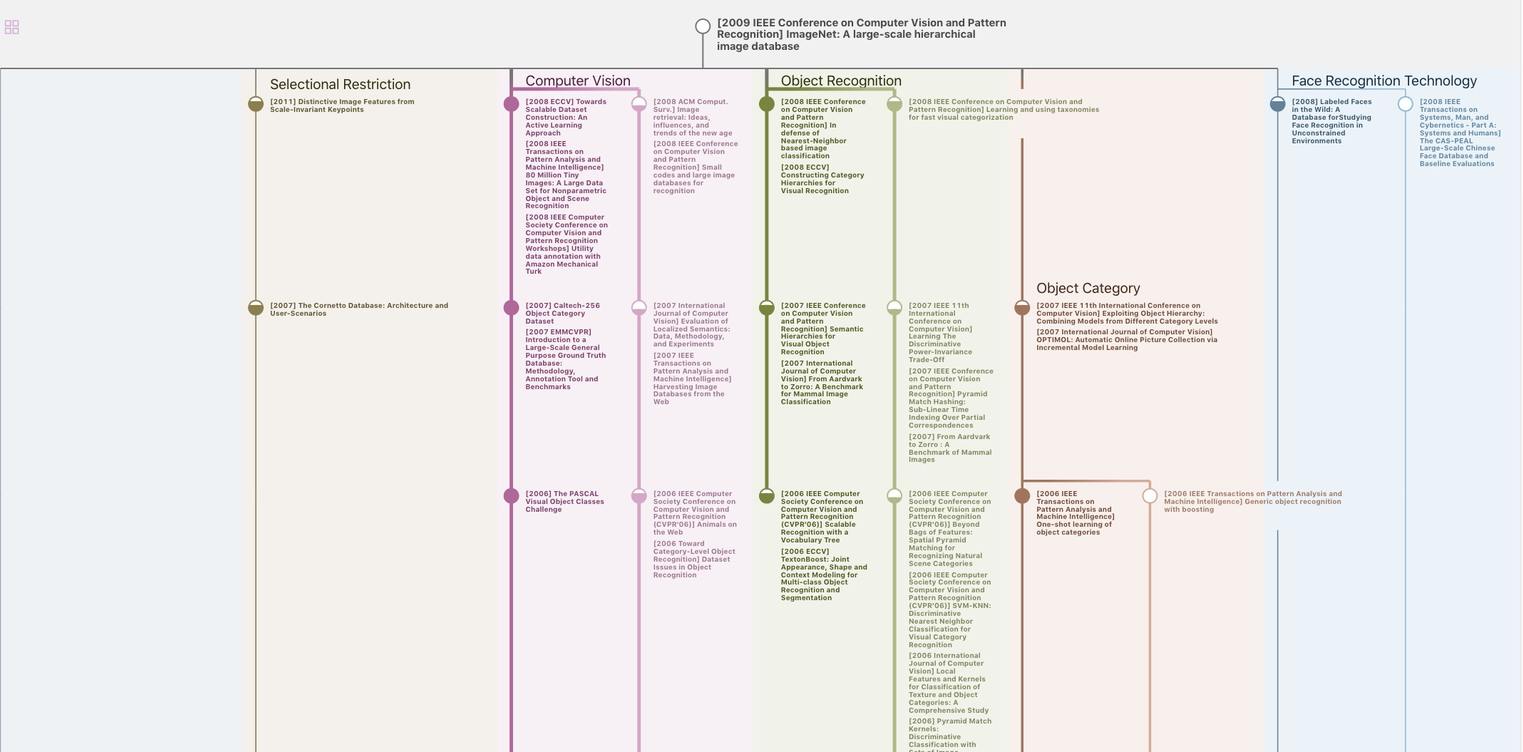
生成溯源树,研究论文发展脉络
Chat Paper
正在生成论文摘要