Self-Supervised Contrastive Learning for Robust Audio Sheet Music Retrieval Systems
PROCEEDINGS OF THE 2023 PROCEEDINGS OF THE 14TH ACM MULTIMEDIA SYSTEMS CONFERENCE, MMSYS 2023(2023)
摘要
Linking sheet music images to audio recordings remains a key problem for the development of efficient cross-modal music retrieval systems. One of the fundamental approaches toward this task is to learn a cross-modal embedding space via deep neural networks that is able to connect short snippets of audio and sheet music. However, the scarcity of annotated data from real musical content affects the capability of such methods to generalize to real retrieval scenarios. In this work, we investigate whether we can mitigate this limitation with self-supervised contrastive learning, by exposing a network to a large amount of real music data as a pre-training step, by contrasting randomly augmented views of snippets of both modalities, namely audio and sheet images. Through a number of experiments on synthetic and real piano data, we show that pretrained models are able to retrieve snippets with better precision in all scenarios and pre-training configurations. Encouraged by these results, we employ the snippet embeddings in the higher-level task of cross-modal piece identification and conduct more experiments on several retrieval configurations. In this task, we observe that the retrieval quality improves from 30% up to 100% when real music data is present. We then conclude by arguing for the potential of self-supervised contrastive learning for alleviating the annotated data scarcity in multi-modal music retrieval models. Code and trained models are accessible at https://github.com/luisfvc/ucasr.
更多查看译文
关键词
multi-modal embedding spaces,audio-sheet music retrieval
AI 理解论文
溯源树
样例
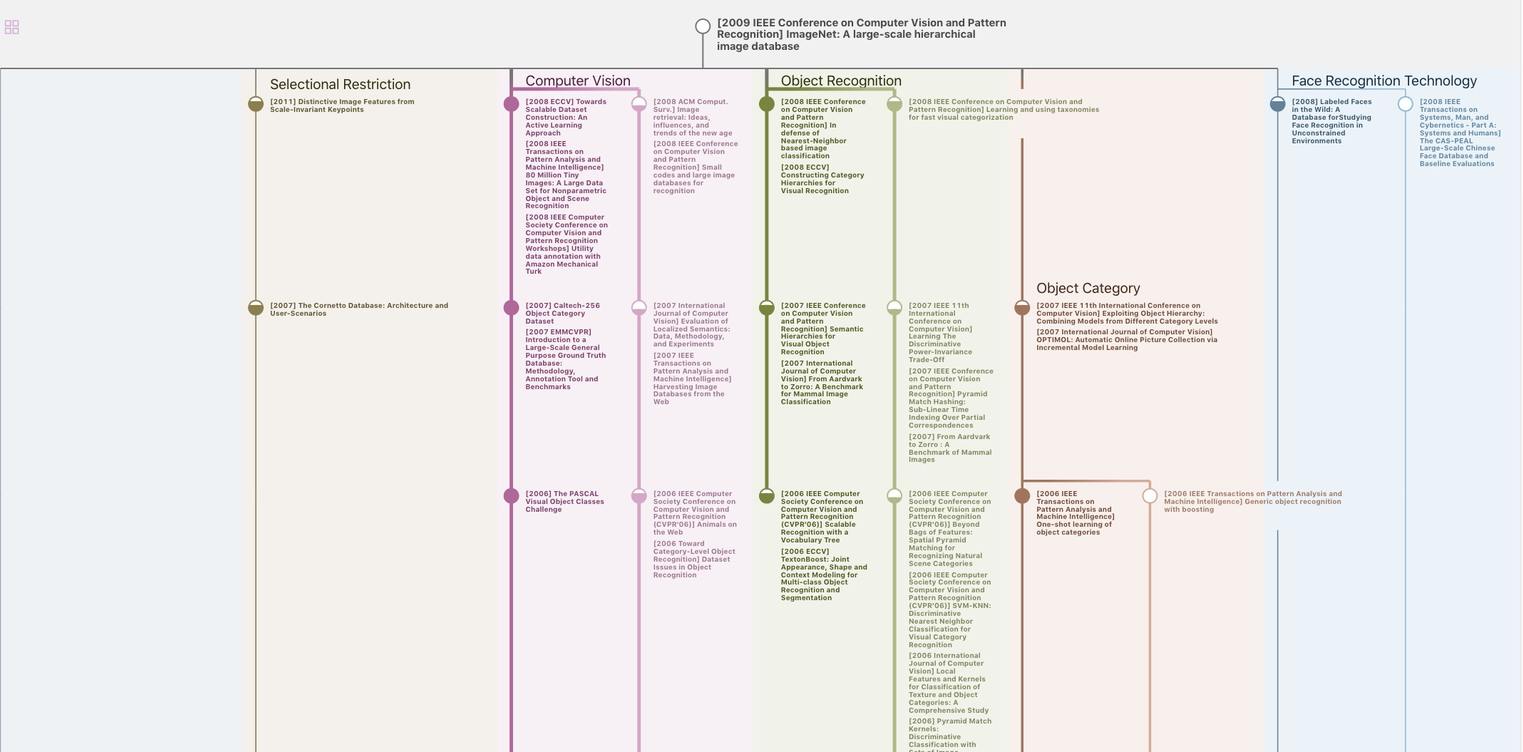
生成溯源树,研究论文发展脉络
Chat Paper
正在生成论文摘要