Improving Image Encoders for General-Purpose Nearest Neighbor Search and Classification
ICMR '23: Proceedings of the 2023 ACM International Conference on Multimedia Retrieval(2023)
摘要
Recent advances in computer vision research led to large vision foundation models that generalize to a broad range of image domains and perform exceptionally well in various image based tasks. However, content-based image-to-image retrieval is often overlooked in this context. This paper investigates the effectiveness of different vision foundation models on two challenging nearest neighbor search-based tasks: zero-shot retrieval and k-NN classification. A benchmark for evaluating the performance of various vision encoders and their pre-training methods is established, where significant differences in the performance of these models are observed. Additionally, we propose a fine-tuning regime that improves zero-shot retrieval and k-NN classification through training with a combination of large publicly available datasets without specializing in any data domain. Our results show that the retrained vision encoders have a higher degree of generalization across different search-based tasks and can be used as general-purpose embedding models for image retrieval.
更多查看译文
关键词
Content-Based Image Retrieval, Deep Learning, Generalization in Nearest Neighbor-Based Tasks
AI 理解论文
溯源树
样例
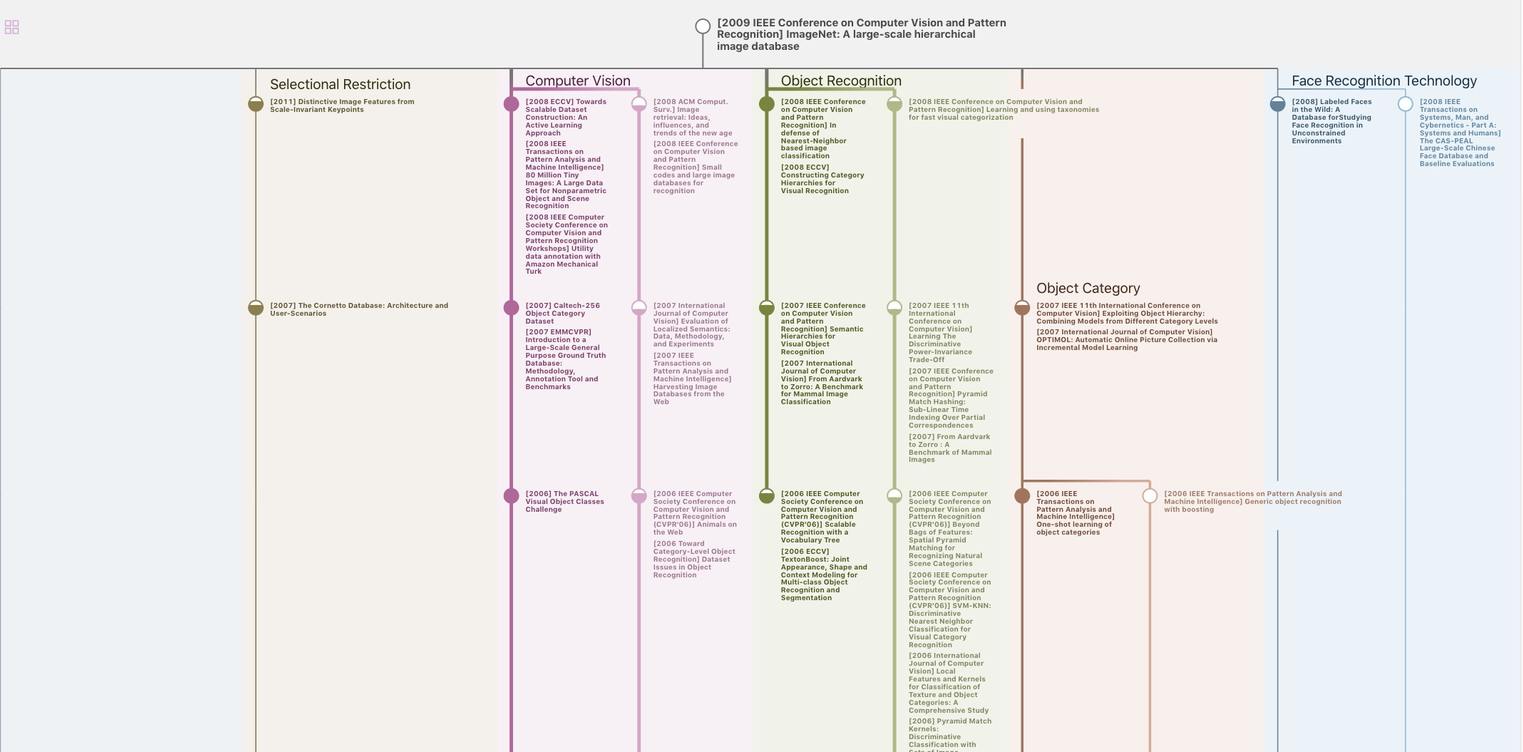
生成溯源树,研究论文发展脉络
Chat Paper
正在生成论文摘要