Graph Interactive Network with Adaptive Gradient for Multi-Modal Rumor Detection
ICMR '23: Proceedings of the 2023 ACM International Conference on Multimedia Retrieval(2023)
摘要
With more and more messages in the form of text and image being spread on the Internet, multi-modal rumor detection has become the focus of recent research. However, most of the existing methods simply concatenate or fuse image features with text features, which can not fully explore the interaction between modalities. Meanwhile, they ignore the convergence inconsistency problem between strong and weak modalities, that is, the dominant rumor text modality may inhibit the optimization of image modality. In this paper, we investigate multi-modal rumor detection from a novel perspective, and propose a Multi-modal Graph Interactive Network with Adaptive Gradient (MGIN-AG) to solve the problem of insufficient information mining within and between modalities, and alleviate the optimization imbalance. Specifically, we first construct fine-grained graph for each rumor text or image to explicitly capture the relation between text tokens or image patches in uni-modal. Then, the cross modal interaction graph between text and image is designed to implicitly mine the text-image interaction, especially focusing on the consistency and mutual enhancement between image patches and text tokens. Furthermore, we extract the embedded text in images as an important supplement to improve the performance of the model. Finally, a strategy of dynamically adjusting the model gradient is introduced to alleviate the under optimization problem of weak modalities in the multi-modal rumor detection task. Extensive experiments demonstrate the superiority of our model in comparison with the state-of-the-art baselines.
更多查看译文
关键词
rumor detection, fake news detection, multi-modal fusion, social networks, graph neural networks
AI 理解论文
溯源树
样例
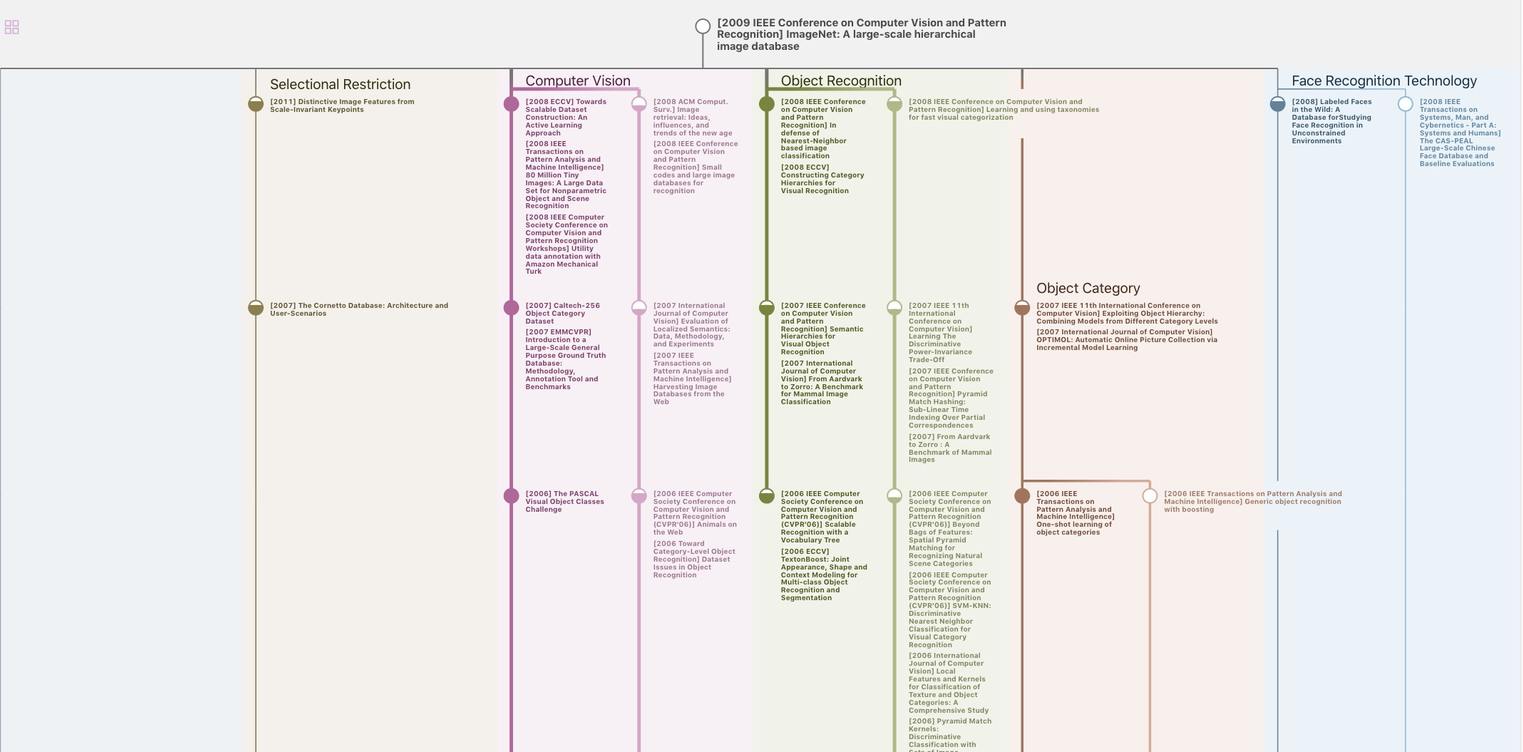
生成溯源树,研究论文发展脉络
Chat Paper
正在生成论文摘要