Sub-Entity Embedding for inductive spatio-temporal knowledge graph completion
Future Generation Computer Systems(2023)
摘要
Existing large-scale knowledge graphs generally contain rich spatial and temporal information. Knowledge graph completion has gained wide attention with a massive number of models proposed for inferring missing knowledge. Despite the fact that numerous approaches to knowledge graph completion exist, they often overlook the importance of simultaneously modeling spatial and temporal information, resulting in limited capacity to infer knowledge related to time and location. An intuitive solution to this issue is to use quintuple representation for spatio-temporal facts. To reduce the complexity of learning quintuples, in this paper, we propose sub-entity to tokenize every entity, relation, time stamp and location with a fixed-size vocabulary. Meanwhile, we design Spatio-Temporal Message Passing layer to learn the latent feature vectors of a knowledge graph. We conducted entity link prediction, relation link prediction, time prediction and location prediction experiments. The quantitative results demonstrate the effectiveness of our model in both predicting missing knowledge under both transductive link prediction and inductive link prediction. The visualization results also show that our model can capture meaningful time and location information.& COPY; 2023 Elsevier B.V. All rights reserved.
更多查看译文
关键词
Knowledge graph,Knowledge graph embedding,Knowledge graph completion,Spatio-temporal data
AI 理解论文
溯源树
样例
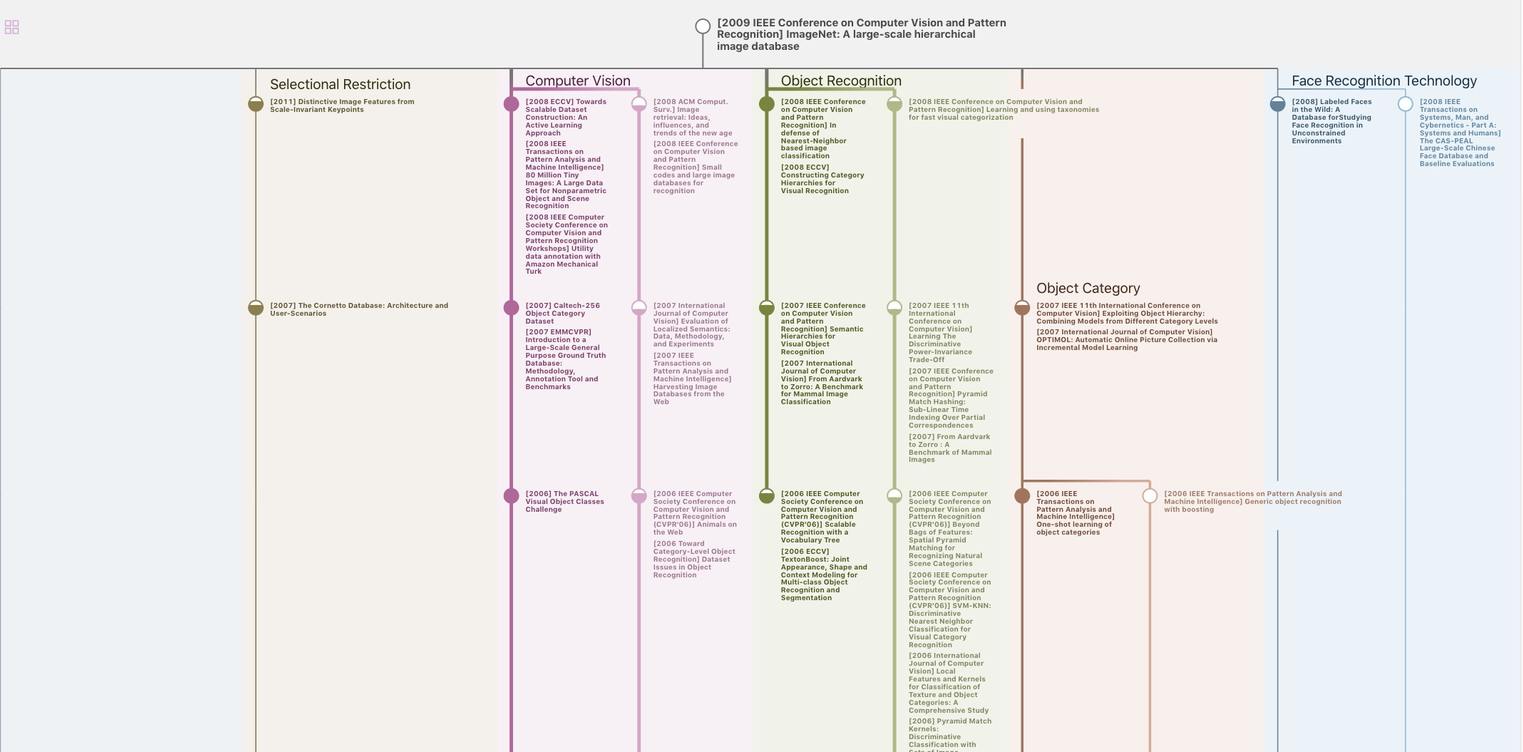
生成溯源树,研究论文发展脉络
Chat Paper
正在生成论文摘要