Background Prompting for Improved Object Depth
CoRR(2023)
摘要
Estimating the depth of objects from a single image is a valuable task for many vision, robotics, and graphics applications. However, current methods often fail to produce accurate depth for objects in diverse scenes. In this work, we propose a simple yet effective Background Prompting strategy that adapts the input object image with a learned background. We learn the background prompts only using small-scale synthetic object datasets. To infer object depth on a real image, we place the segmented object into the learned background prompt and run off-the-shelf depth networks. Background Prompting helps the depth networks focus on the foreground object, as they are made invariant to background variations. Moreover, Background Prompting minimizes the domain gap between synthetic and real object images, leading to better sim2real generalization than simple finetuning. Results on multiple synthetic and real datasets demonstrate consistent improvements in real object depths for a variety of existing depth networks. Code and optimized background prompts can be found at: https://mbaradad.github.io/depth_prompt.
更多查看译文
关键词
improved object depth,background,prompting
AI 理解论文
溯源树
样例
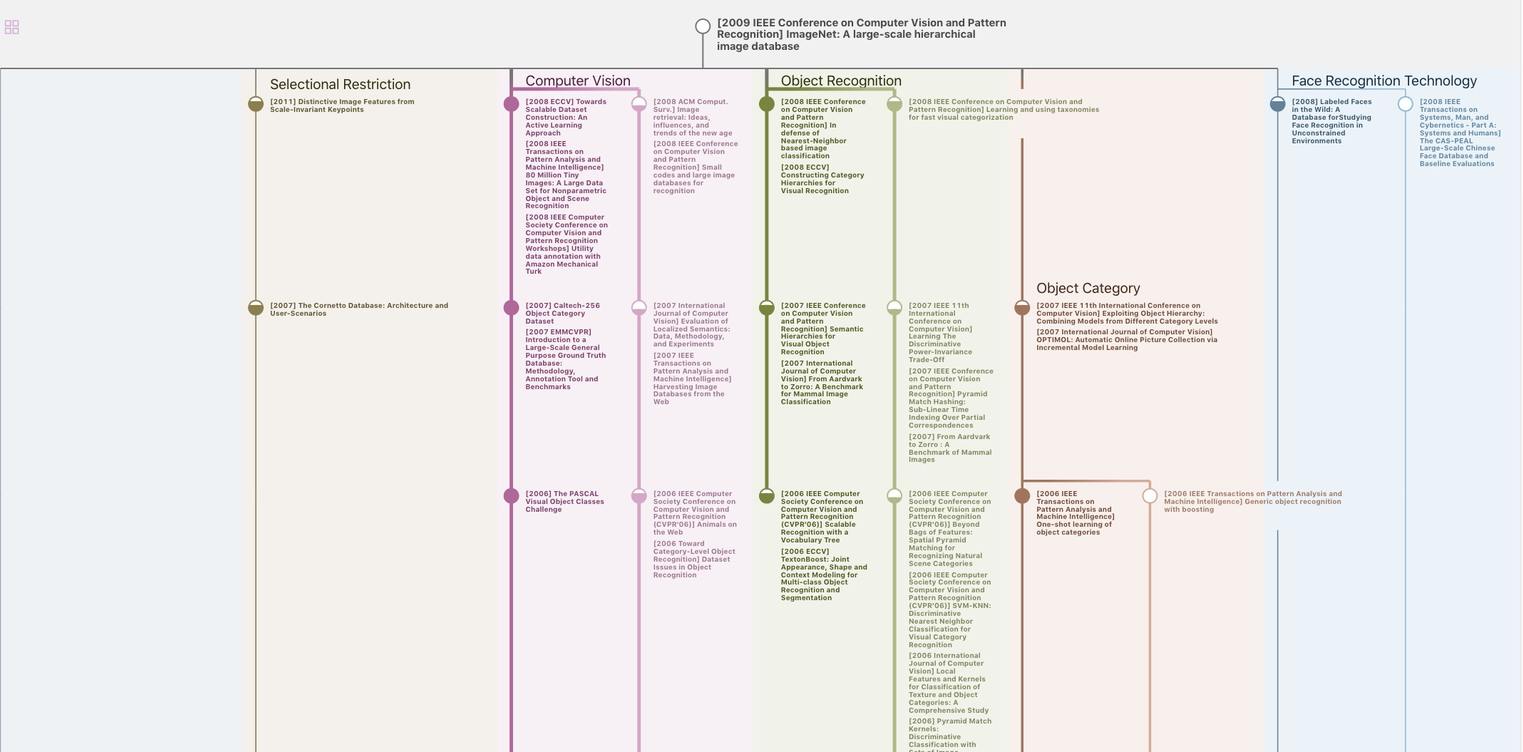
生成溯源树,研究论文发展脉络
Chat Paper
正在生成论文摘要