Focus for Free in Density-Based Counting
International Journal of Computer Vision(2024)
摘要
This work considers supervised learning to count from images and their corresponding point annotations. Where density-based counting methods typically use the point annotations only to create Gaussian-density maps, which act as the supervision signal, the starting point of this work is that point annotations have counting potential beyond density map generation. We introduce two methods that repurpose the available point annotations to enhance counting performance. The first is a counting-specific augmentation that leverages point annotations to simulate occluded objects in both input and density images to enhance the network’s robustness to occlusions. The second method, foreground distillation, generates foreground masks from the point annotations, from which we train an auxiliary network on images with blacked-out backgrounds. By doing so, it learns to extract foreground counting knowledge without interference from the background. These methods can be seamlessly integrated with existing counting advances and are adaptable to different loss functions. We demonstrate complementary effects of the approaches, allowing us to achieve robust counting results even in challenging scenarios such as background clutter, occlusion, and varying crowd densities. Our proposed approach achieves strong counting results on multiple datasets, including ShanghaiTech Part_A and Part_B, UCF_QNRF, JHU-Crowd++, and NWPU-Crowd. Code is available at https://github.com/shizenglin/Counting-with-Focus-for-Free .
更多查看译文
关键词
Object counting,Deep learning,Vision transformer
AI 理解论文
溯源树
样例
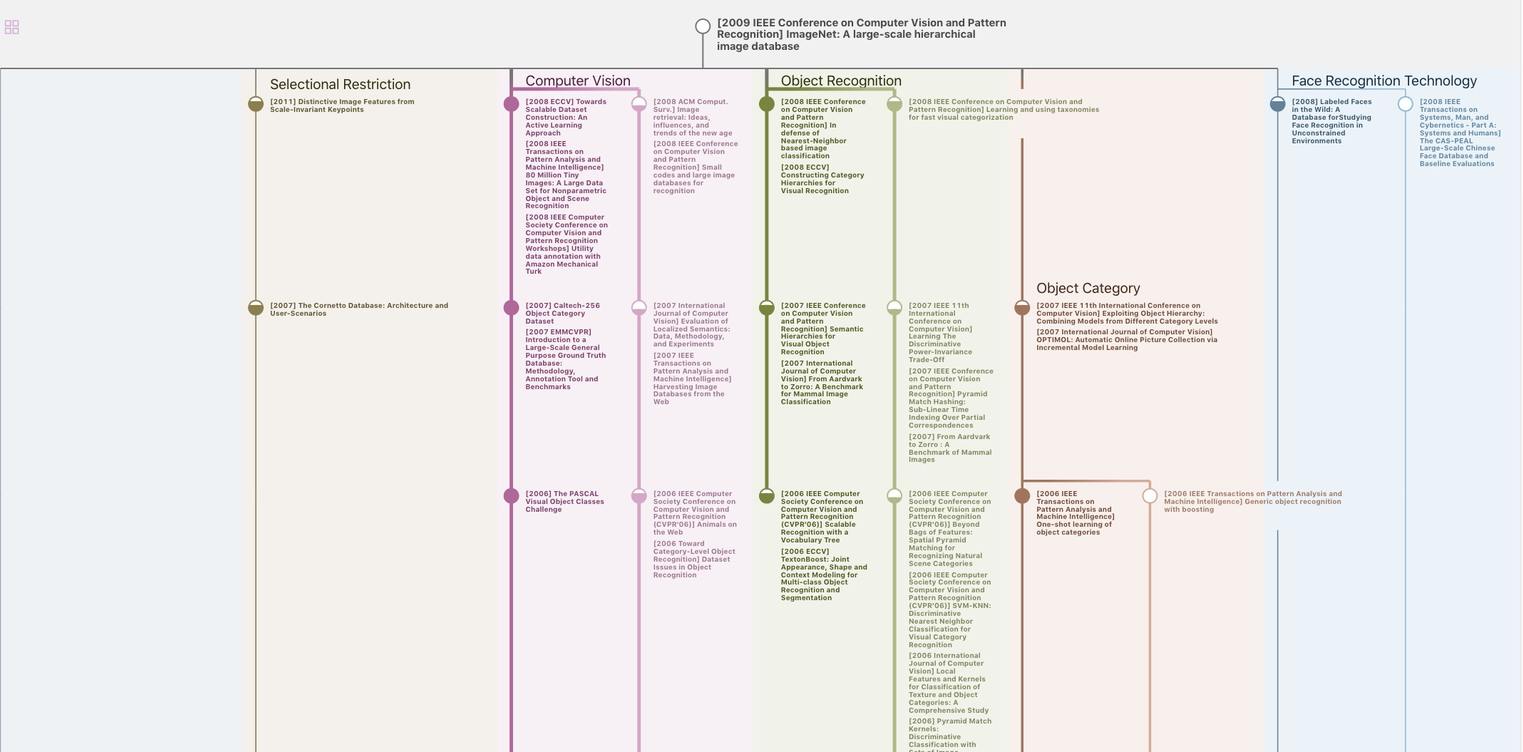
生成溯源树,研究论文发展脉络
Chat Paper
正在生成论文摘要