Differentiable Earth mover's distance for data compression at the high-luminosity LHC
MACHINE LEARNING-SCIENCE AND TECHNOLOGY(2023)
摘要
The Earth mover's distance (EMD) is a useful metric for image recognition and classification, but its usual implementations are not differentiable or too slow to be used as a loss function for training other algorithms via gradient descent. In this paper, we train a convolutional neural network (CNN) to learn a differentiable, fast approximation of the EMD and demonstrate that it can be used as a substitute for computing-intensive EMD implementations. We apply this differentiable approximation in the training of an autoencoder-inspired neural network (encoder NN) for data compression at the high-luminosity LHC at CERN The goal of this encoder NN is to compress the data while preserving the information related to the distribution of energy deposits in particle detectors. We demonstrate that the performance of our encoder NN trained using the differentiable EMD CNN surpasses that of training with loss functions based on mean squared error.
更多查看译文
关键词
Earth movers distance,data compression,convolutional neural networks,high energy physics
AI 理解论文
溯源树
样例
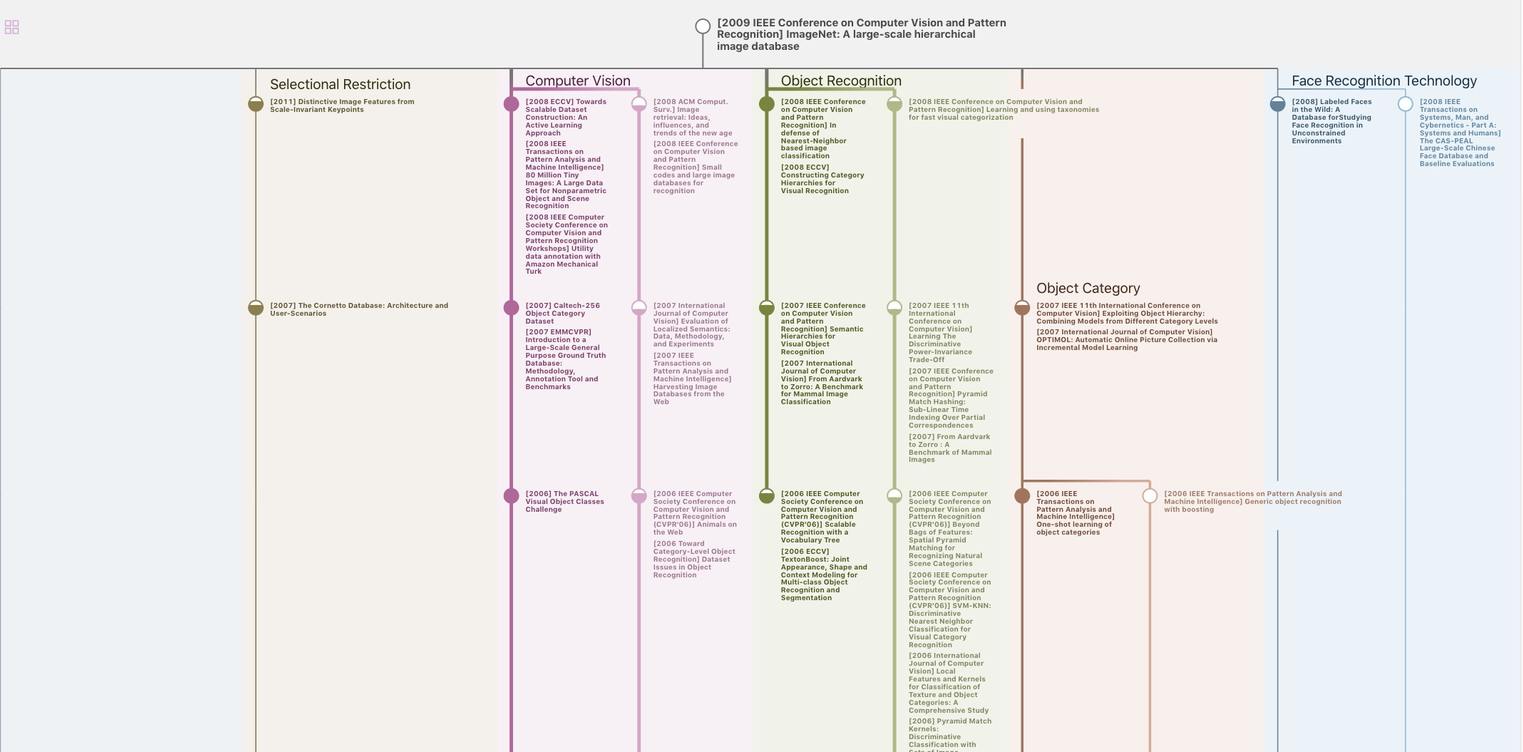
生成溯源树,研究论文发展脉络
Chat Paper
正在生成论文摘要