DiViNeT: 3D Reconstruction from Disparate Views via Neural Template Regularization
NeurIPS(2023)
摘要
We present a volume rendering-based neural surface reconstruction method that takes as few as three disparate RGB images as input. Our key idea is to regularize the reconstruction, which is severely ill-posed and leaving Our method, coined DiViNet, operates in two stages. The first stage learns the templates, in the form of 3D Gaussian functions, across different scenes, without 3D supervision. In the reconstruction stage, our predicted templates serve as anchors to help ``stitch'' the surfaces over sparse regions. We demonstrate that our approach is not only able to complete the surface geometry but also reconstructs surface details to a reasonable extent from few disparate input views. On the DTU and BlendedMVS datasets, our approach achieves the best reconstruction quality among existing methods in the presence of such sparse views, and performs on par, if not better, with competing methods when dense views are employed as inputs.
更多查看译文
关键词
3d reconstruction,disparate views,neural template
AI 理解论文
溯源树
样例
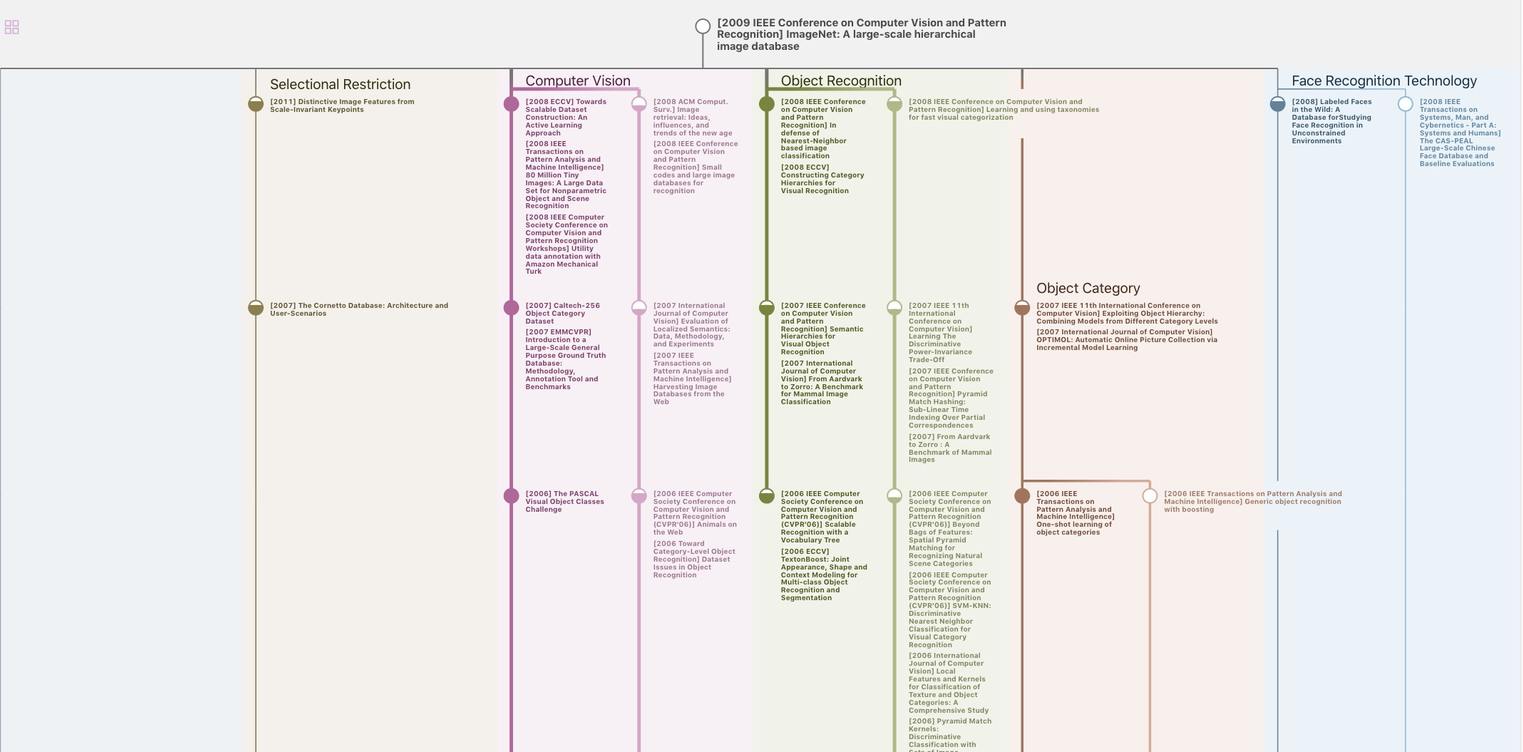
生成溯源树,研究论文发展脉络
Chat Paper
正在生成论文摘要