Multiscale Flow for robust and optimal cosmological analysis
PROCEEDINGS OF THE NATIONAL ACADEMY OF SCIENCES OF THE UNITED STATES OF AMERICA(2024)
摘要
We propose Multiscale Flow, a generative Normalizing Flow that creates samples and models the field -level likelihood of two-dimensional cosmological data such as weak lensing. Multiscale Flow uses hierarchical decomposition of cosmological fields via a wavelet basis and then models different wavelet components separately as Normalizing Flows. The log -likelihood of the original cosmological field can be recovered by summing over the log -likelihood of each wavelet term. This decomposition allows us to separate the information from different scales and identify distribution shifts in the data such as unknown scale -dependent systematics. The resulting likelihood analysis can not only identify these types of systematics, but can also be made optimal, in the sense that the Multiscale Flow can learn the full likelihood at the field without any dimensionality reduction. We apply Multiscale Flow to weak lensing mock datasets for cosmological inference and show that it significantly outperforms traditional summary statistics such as power spectrum and peak counts, as well as machine learning-based summary statistics such as scattering transform and convolutional neural networks. We further show that Multiscale Flow is able to identify distribution shifts not in the training data such as baryonic effects. Finally, we demonstrate that Multiscale Flow can be used to generate realistic samples of weak lensing data.
更多查看译文
关键词
field-level inference,deep learning,normalizing flow,large-scale structure,weak lensing
AI 理解论文
溯源树
样例
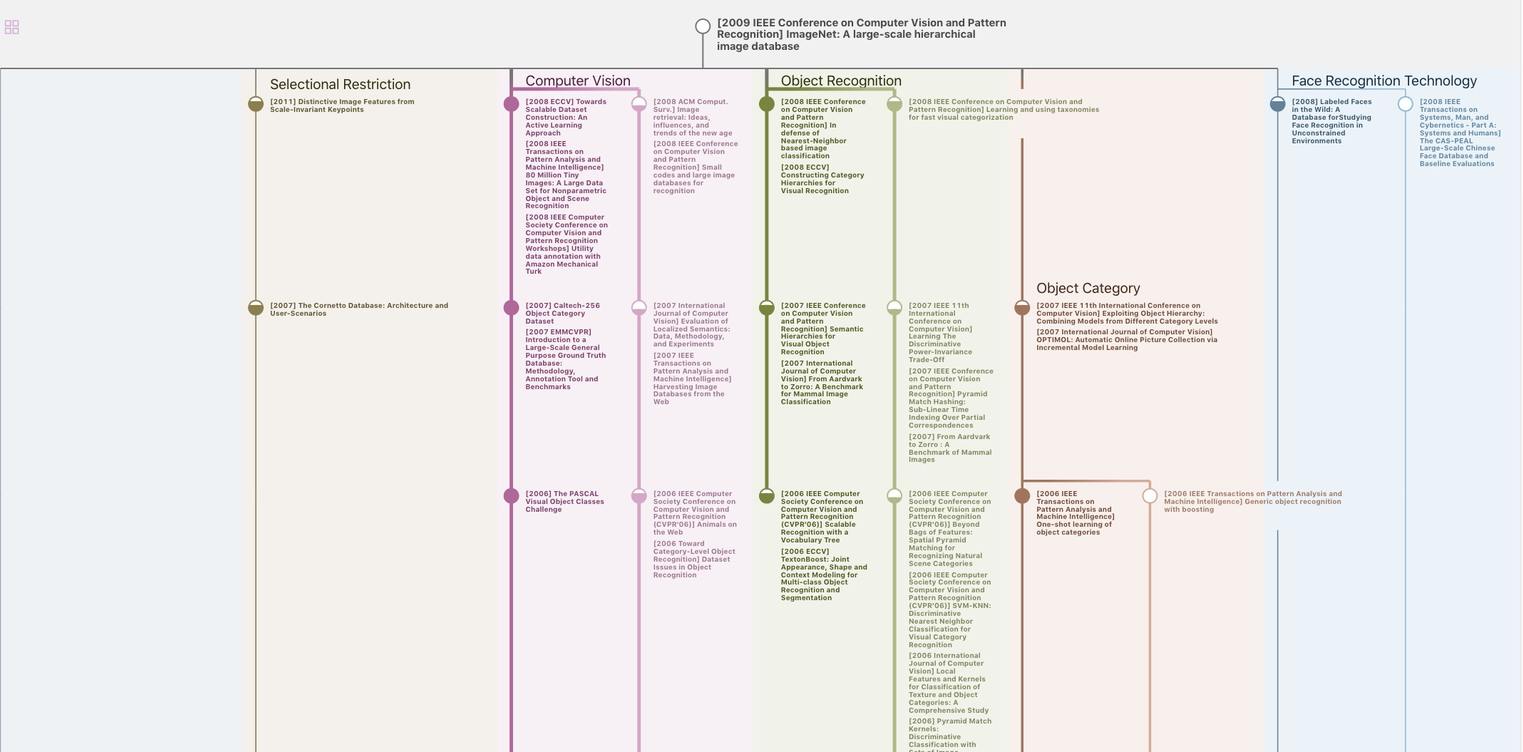
生成溯源树,研究论文发展脉络
Chat Paper
正在生成论文摘要