MLP-UNet: Glomerulus Segmentation
IEEE Access(2023)
摘要
Glomerulus segmentation in kidney tissue segments is a crucial nephropathology process used to diagnose renal diseases effectively. This study proposes a novel and robust application of MLP (Multi-Layer Perceptron) based architectures for the segmentation of glomeruli in PAS (Periodic AcidSchiff) stained whole renal images for effective diagnosis of renal diseases. For the segmentation challenge, the proposed unique solution uses MLP-UNet (Multi-Layer Perceptron U-Net), a novel design that evades using conventional convolution and self-attention mechanisms. Additionally, the study compares various approaches, including U-Net, and for the first time, trains the TransUNet model on the kidney WSI (Whole Slide Image) dataset. Dice Score and Dice Loss were used for training these models as the metric and loss function. Results showed that MLP-based architectures provide comparable results (89.96%) to pre-trained architectures like TransUNet (90.58%) with effectively 20% lesser parameters and no pre-training, and also produce superior Dice scores across the 5-fold cross-validation training and learn more quickly than conventional U-Net architectures.
更多查看译文
关键词
Computer vision,image segmentation,semantic segmentation,deep learning,biomedical image processing,artificial intelligence,machine learning,computational complexity,neural networks,residual neural networks,convolutional neural networks (CNNs),multi-layer perceptrons (MLP)
AI 理解论文
溯源树
样例
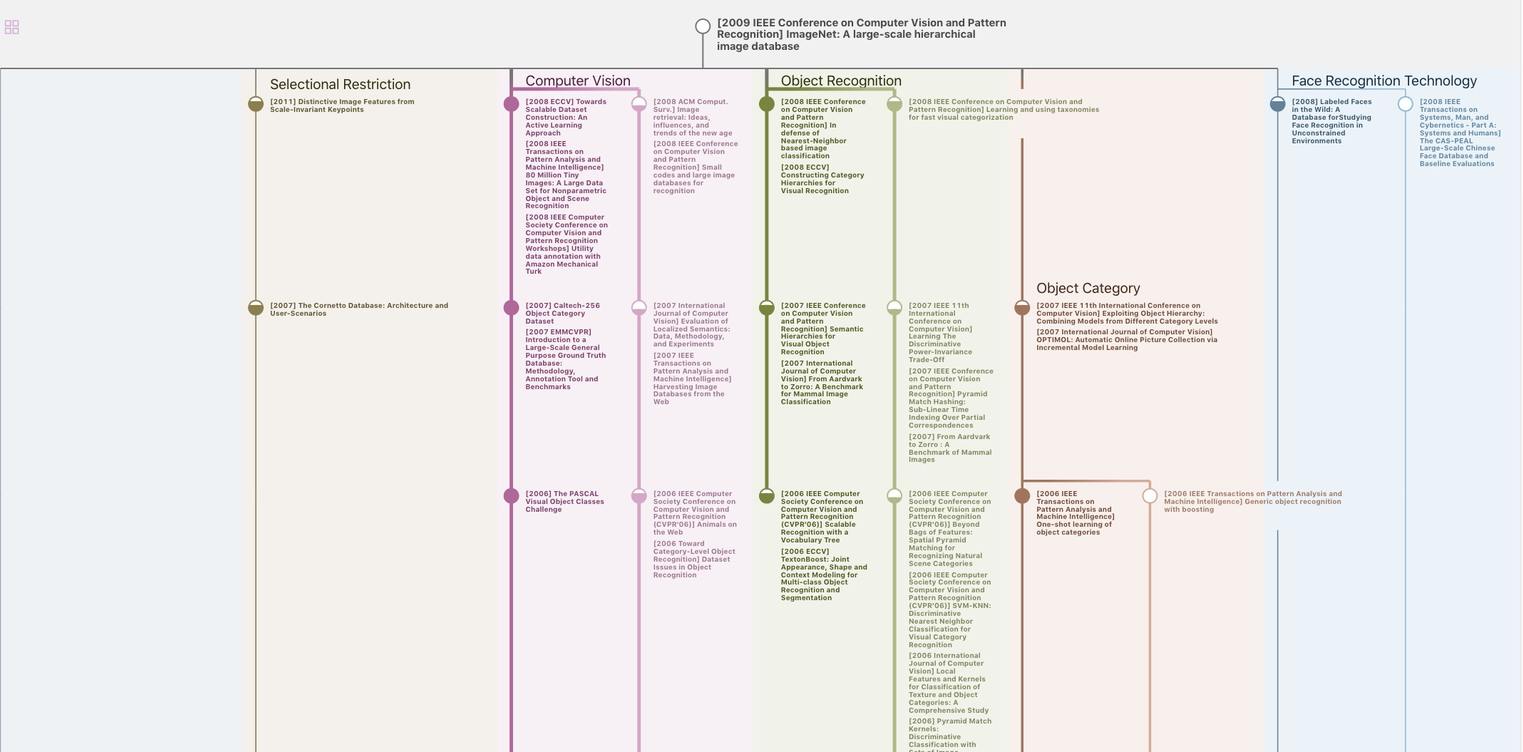
生成溯源树,研究论文发展脉络
Chat Paper
正在生成论文摘要