Analysing the Robustness of NSGA-II under Noise
CoRR(2023)
摘要
Runtime analysis has produced many results on the efficiency of simple evolutionary algorithms like the (1+1) EA, and its analogue called GSEMO in evolutionary multiobjective optimisation (EMO). Recently, the first runtime analyses of the famous and highly cited EMO algorithm NSGA-II have emerged, demonstrating that practical algorithms with thousands of applications can be rigorously analysed. However, these results only show that NSGA-II has the same performance guarantees as GSEMO and it is unclear how and when NSGA-II can outperform GSEMO. We study this question in noisy optimisation and consider a noise model that adds large amounts of posterior noise to all objectives with some constant probability.. per evaluation. We show that GSEMO fails badly on every noisy fitness function as it tends to remove large parts of the population indiscriminately. In contrast, NSGA-II is able to handle the noise efficiently on LeadingOnesTrailingZeroes when.. < 1/2, as the algorithm is able to preserve useful search points. We identify a phase transition at.. = 1/2 where the expected time to cover the Pareto front changes from polynomial to exponential. This is the first proof that NSGA-II can outperform GSEMO and the first runtime analysis of NSGA-II in noisy optimisation.
更多查看译文
关键词
Runtime analysis,evolutionary multiobjective optimisation,noisy optimisation
AI 理解论文
溯源树
样例
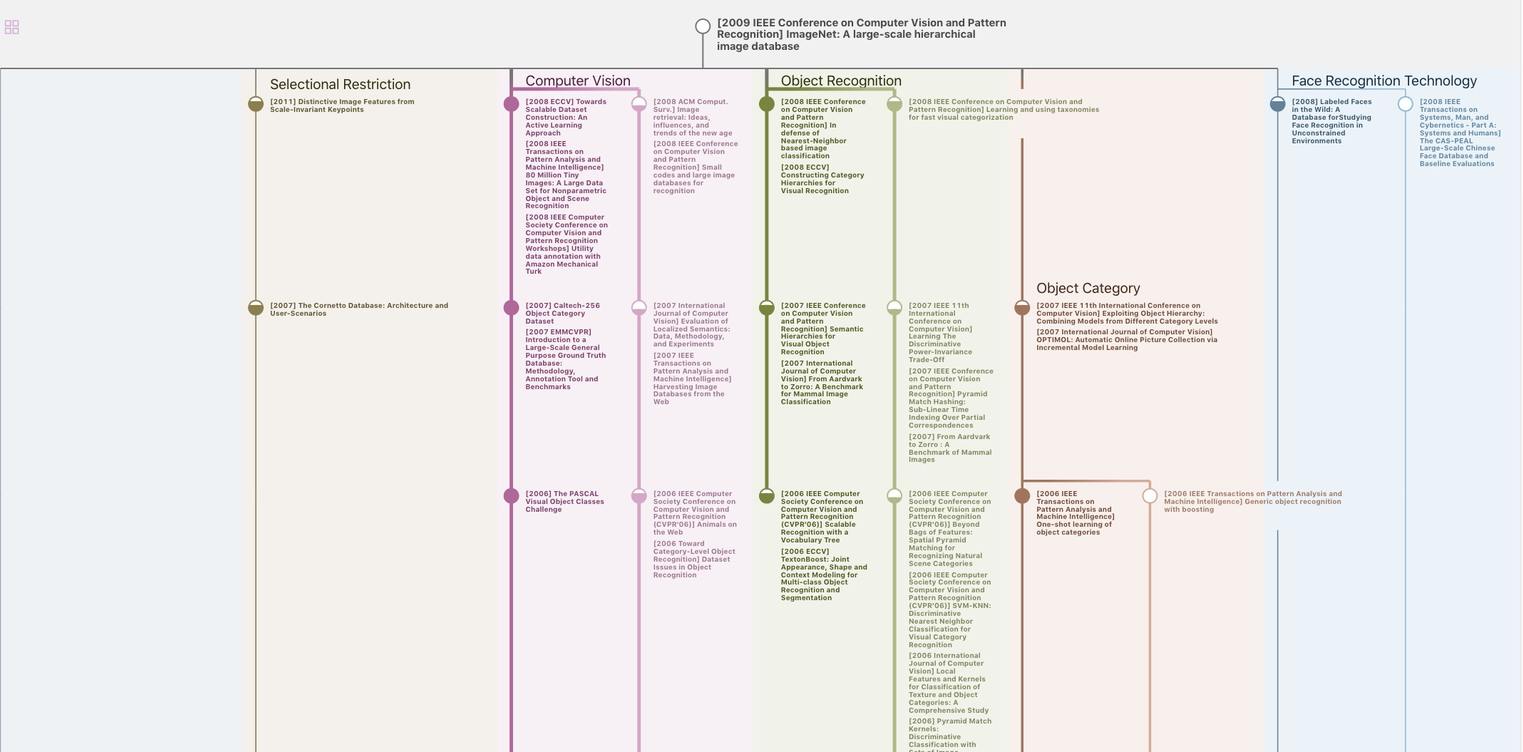
生成溯源树,研究论文发展脉络
Chat Paper
正在生成论文摘要